1 Introduction
Natural products have intimate relationships with medicine and chemistry, with various examples from ancient civilizations throughout history. Most of these uses include those in traditional or herbal medicine, to which also mystical properties to the plants or fungi concerned have sometimes been attributed. For example, sage is a herb that was thought to ward off evil. Nowadays, it is known that sage possesses several biological effects, for example, antibacterial, antioxidant, and cholinergic [1]. In a similar manner, other traditional uses have been validated by scientific research [2–5].
As such, natural sources have driven the early stages of medicinal chemistry and drug discovery, yielding valuable therapeutic agents still in use today. Prominent examples of drugs approved for clinical use from natural sources include, but are not limited to, penicillin, pilocarpine, reserpine, and salicylic acid. Furthermore, the role of natural products as novel avenues for therapy increased after the so-called Golden Age of Antibiotics (circa 1960) when the larger companies in the pharmaceutical industry began the development of numerous projects, searching for molecules with diverse bioactivities [6]. However, the “golden age” of natural products as antibiotics was quite short, since most companies reduced such endeavors by the turn of the twenty-first century [7]. Several reasons have been given that help explain the decreased enthusiasm of pharmaceutical companies to work on natural products. Two major points are the inherent complexity of crude extract compound mixtures and the slowness of natural product optimization [8]. Additionally, with the rapid development of combinatorial chemistry and high-throughput methods, the search for chemical diversity was considered a solved problem. Unfortunately, this has not been the case, as it has been shown that combinatorial collections tend to get trapped in the same area of chemical space [9]. Moreover, even with the ability to produce compounds in high numbers, only a handful of Food and Drug Administration (FDA)-approved drugs come from such methods [10]. Therefore, it can be argued that the solution of the problem “quantity over quality” is “quality over quantity”.
As a result, natural products have seen a “rebirth” with novel methods and synthesis strategies to produce diverse collections [11]. Additionally, in most cases, vegetal sources are the major players in natural product research. Thus, other sources like marine, bacterial, and fungal metabolites offer untapped potential [12, 13]. As recently reviewed, there are several recently approved drugs that are natural products or are synthetic analogs of hit compounds initially identified from natural sources. A clear and recent example is the fungal metabolite migalastat (Galafold®) approved in 2018 for the treatment of Fabry disease [14].
Due to these considerations, current efforts involve multidisciplinary approaches, which help mitigate the problems inherent to natural products. This mainly focuses on the improvement of extraction, isolation, and quality control of metabolites, including “omics technology” [15]. Nonetheless, other technological approaches have arisen. Take, for example, the high volume of information available on natural products and their activities. We now live in an era of “big data”, with different dedicated repositories [16]. The rational and effective mining of such databases could yield important breakthroughs.
It is well known that many natural products exert multiple effects in vitro, and, because of this promiscuous nature, some classes of natural products are among the Pan Assay Interference Compounds (PAINS, see Sect. 3) [17]. It follows that a screening campaign might well filter scaffolds of natural products to identify promising ones, while also discarding PAIN-like moieties. In practice, this can be accomplished rather easily, by conducting a virtual screening that is an in silico method (part of cheminformatics) aimed at selecting compounds with potential biological activity.
A rather “young” discipline, cheminformatics, is envisioned as the answer for chemical information problems using several numerical, statistical, and physicochemical methods to work with two- and three-dimensional chemical structures [18]. This aims to optimize resources more effectively and to focus on the more viable molecules. Therefore, cheminformatics relies heavily on concepts like chemical space, molecular similarity, and chemical representation [19]. More recently, the scope of cheminformatics has shifted toward in silico evaluation, using molecular modeling approaches and machine learning.
The goal of this chapter is to discuss the progress of selected cheminformatic strategies to further advance the identification of bioactive molecules from natural origin. This contribution is organized in five major sections. After this introduction, Sect. 2 discusses examples of mining the space of natural products using several virtual screening strategies, including similarity searching, automated docking, and consensus methods. In this section, case studies are described of virtual screening for the identification of bioactive molecules against epigenetic targets. Section 3 discusses the in silico toxicity profiling of natural product datasets. Next, Sect. 4 covers the analysis of the chemical diversity and coverage in chemical space as well as the design of natural product-like molecules and natural product mimetics. Section 5 presents summary conclusions and perspectives.
2 Mining Natural Product Spaces: Identification of Bioactive Compounds
Representative computational methods and concepts used for virtual screening
Method/concept | Brief description | Refs. |
---|---|---|
Chemical space | Abstract representation of compounds, using different descriptors. This allows the profiling of chemical collections | [20] |
Molecular similarity | Using graph decomposition, molecular structures are codified as vectors. These in turn can be compared using different equations to measure similarity | [21] |
QSAR | Mathematical models supported by descriptors that quantify the impact of substituents in biological activity. Their main aim is the prediction of biological activity | [22] |
Molecular docking | Simulation that approximates protein-ligand binding. This is accomplished by the conformational searches of ligands and the evaluation of these using dG values as criteria | [23] |
Molecular dynamics | Physical simulations that allow the study of protein behavior, using equations of motion and potential energy functions (forcefields) | [24] |
Free energy perturbations | Derivatives of molecular dynamics, in this case the simulation goes across a thermodynamic cycle. This can be used for the approximation of binding energy and the change in its value due to fragment changes | [25] |
Usually, a virtual screening protocol involves various methods in consecutive order, trying to filter large databases to “cherry-pick” putative ligands of interest. Thus far, virtual screening has been applied successfully to identify hit compounds that are usually later optimized [26–28].
In the early days of in silico research, the quintessential approaches were descriptor-based, mostly inspired by the success of the Hansch-Fujita method. This led to the birth of Quantitative Structure Activity Relationships (QSAR) and their more refined counterparts: CoMFA and CoMSIA [29]. A prominent success case being the Lipinski Rule of Five, which describes a general profile of “drug-like” molecules with optimal bioavailability (no more than 5 hydrogen bond donors, no more than 10 hydrogen bond acceptors, M ≤ 500, logP ≤ 5) [30]. Alas, it can be argued that over-reliance on such approaches has led to molecular attrition [31]. In addition, it has been shown that the overall performance of descriptor-based classification depends on the correct assessment of relevant properties [32].
On the other hand, there are receptor-based approaches, with the most well-known of them being molecular docking. One such technique uses the GRID method, developed by Goodford et al., which generates molecular interaction maps in protein cavities [33]. Hence, docking can be used to model drug–protein complexes and perhaps the most appealing aspect of this, the calculation of relative binding energies.
Even so, molecular docking has critical points that may be often overlooked by naive users, for example, structure selection, protein preparation, the inclusion of water molecules and metal ions, and protein flexibility [23, 34]. Furthermore, one of the most important flaws in molecular docking is the pose versus scoring phenomena that are related to the uncertainty of significant results without the proper knowledge of the binding site. Consequently, some protocols and good practices have been proposed for reliable results [35, 36]. In this sense, proper ligand selection has been suggested as a preferred method for docking candidate selection [37].
Of the several approaches for molecule mining, chemical similarity is perhaps the most powerful. Most chemists have encountered this principle, sometimes inadvertently. The rather simple axiom, “similar structures share similar activities,” holds significantly true in a pharmacological context. In practice, chemical similarity provides a tool for systematic and objective comparison of compound pairs. To do this, chemical structures are codified as strings, known as Simplified Molecular Input Line Entries (SMILES). Then follows a comparison based on topology or fragment substructures, commonly performed with the Tanimoto coefficient to compute similarity values [38].
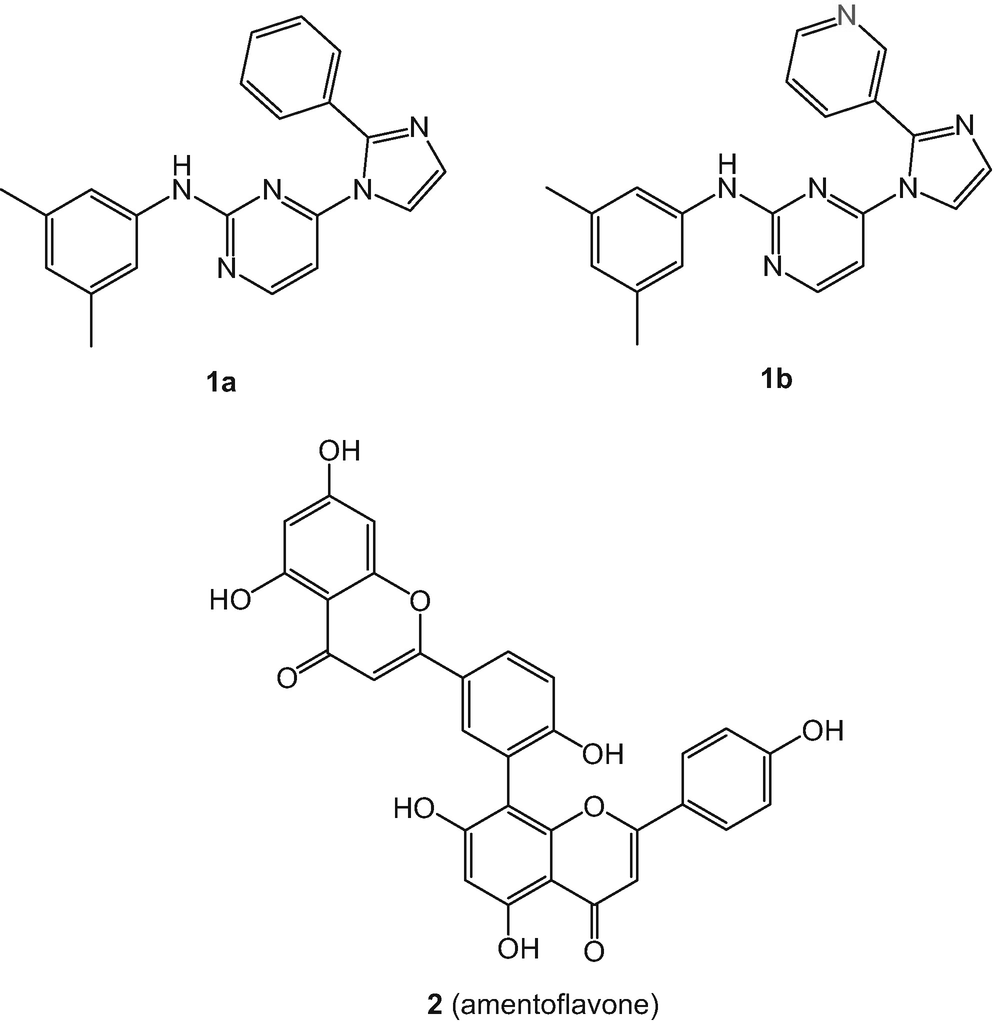
Example of an activity cliff, with the most potent structure being 1b. In this case, the difference in activity between 1a and 1b is almost 400 times. Of note, this large change in activity is due to a single heteroatom. Below, structural formula of amentoflavone (2)
This phenomenon deeply impacts the performance of virtual screening as a whole, not just similarity methods [40]. Accordingly, the best results of virtual screening campaigns are obtained by complementary approaches, also known as consensus [41].
Virtual screening protocols may be implemented rather easily and with such potential, they have been adopted in natural product research. Correspondingly, screening and optimization of natural products has benefited from computational tools. In turn, computational chemists saw the potential of natural products as privileged scaffolds for lead searching, ending in a symbiotic relationship early on. As may be expected, there have been some inherent difficulties and successes along the way. Still, this interdisciplinary environment has led to the development of public repositories and the overall improvement of computational algorithms [42].
Generally, the proposal or study of putative mechanisms of action is the main goal of computational methods in natural product research. For example, DNA topoisomerases have been studied with a wide array of natural products, identifying interaction patterns crucial to enzyme inhibition [43]. These concepts have been scaled further as “target fishing” or reverse virtual screening. In this case, the molecule of interest is used as filter, that is, it is evaluated against several targets to identify significant activities. The value of such studies cannot be overstated, as their utility may range from structure–activity relationship optimization to multi-activity map pathways [44].
Likewise, molecular modeling tools have been used to identify natural product leads with micromolar activities in targets such as acetylcholinesterase (AChE), cytochrome P-450, angiotensin-converting enzyme 2 (ACE-2), kinase CK2, and estrogen receptor-β [42]. On the other hand, consensus protocols have been successful in the screening of marine compounds with assorted activities [13].
As may be seen, natural product mining with virtual screening protocols has proven effective. Of course, there are more examples in different fields, but we consider that among them, the epigenome provides an interesting application for natural products as chemoprotective agents. Here, we discuss recent applications with emphasis on epigenetic targets that are emerging as promising targets for the treatment of several diseases [45–49].
2.1 Case Studies of Virtual Screening for Epigenetic Targets
Epigenetics has become an attractive area of study, first described in 1940 by Conrad Waddington [50]. It refers to heritable changes in gene expression that occur independent of alterations in DNA sequence, but are rather based on modifications of histone proteins or nucleic acids. Since its description, epigenetics is linked to factors such as diet or the environment to explain the biogenesis of some diseases [51].
Currently, epigenetics has provided a novel approach to search for therapies in the treatment of cancer, diabetes, hypertension, or even Alzheimer’s disease. Still, epigenetic modulation is not “black or white”, as several epigenetically modifying enzymes modulate a wide array of physiological functions. In addition, the epi-pocketome continues to grow at steady pace, increasing target diversity and complexity [52, 53]. Hence, the overall safety and scope of epi-therapies are yet quite blurry [54].
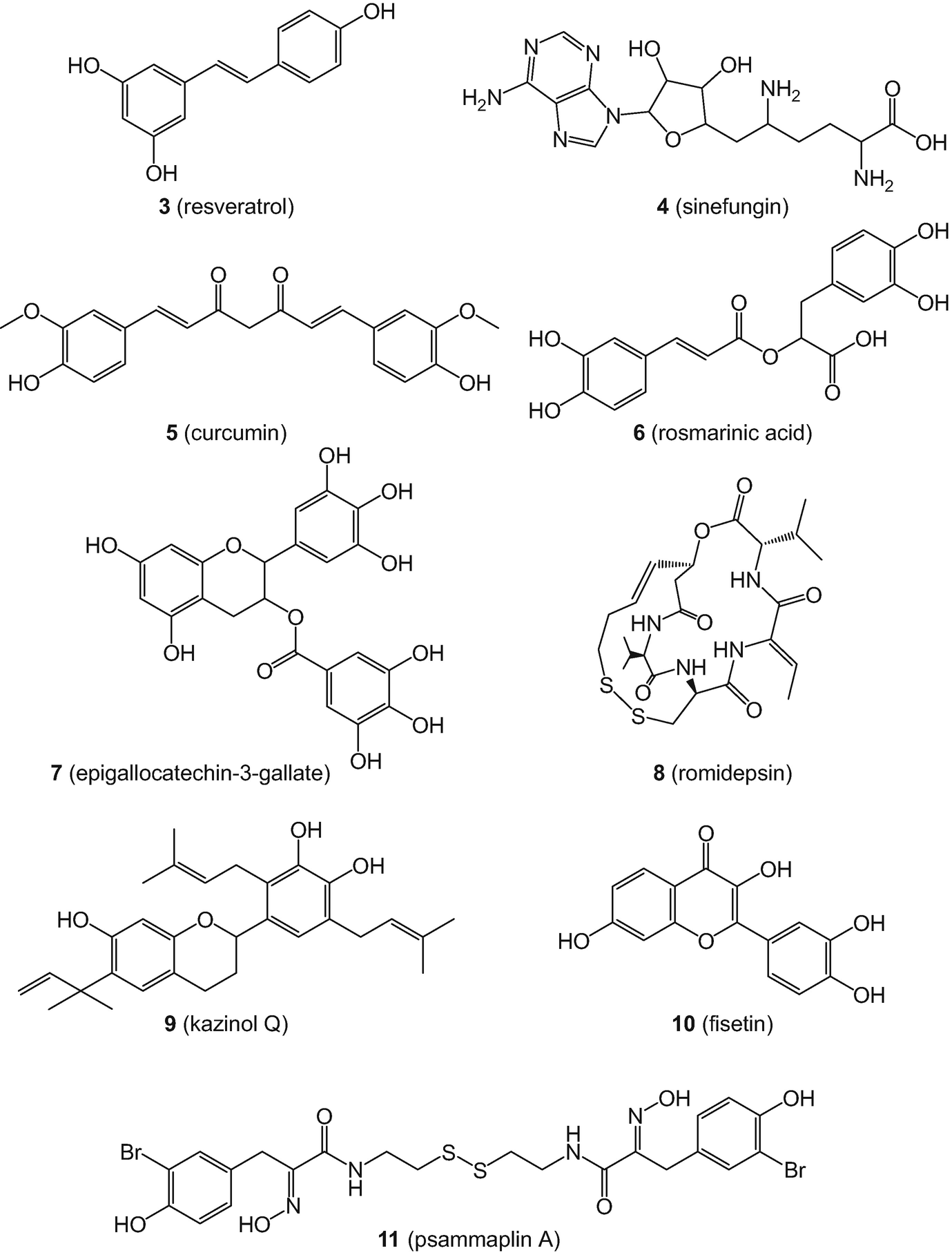
Illustrative examples of natural products reported as epigenetic modulators, as identified by direct or indirect mechanisms. Most of the examples have supportive in silico modeling studies that help to explain their effect
Of note, flavonoids have a privileged place among natural products as therapeutic agents. Often regarded as natural polydrugs, this scaffold has a plethora of biologic actions beyond their antioxidant potential [57]. Considering their abundance in human diet, flavonoids have a well-documented nutraceutical potential [58].
In the next sub-sections, we further comment on some case studies where natural products are involved in serving as leads or to uncover interesting structure–activity relationships.
2.1.1 Bromodomains
Bromodomains (BRDs) are small proteins (around 120 residues) that are classified as epi-readers, that is, enzymes for which the function is focused on recognizing patterns of a given moiety. In this case, bromodomains identify acetylated lysine residues [59]. Currently, over 60 isoforms of bromodomains have been identified from the human proteome; of those, bromodomain and extraterminal domains (BETs) have attracted the most interest so far. This is mainly due to their relation to cancer cell lines and inflammatory processes [60].
One of the pitfalls in bromodomain inhibition is the lack of structural diversity in current inhibitors [61]. As a result of this, there is an ongoing search for novel inhibitors of these targets. Additionally, BET isoforms exhibit high values of sequence similarity in their binding site, making the search more difficult for selective and potent inhibitors.
Recent endeavors in the field include fragment-based virtual screening [62], lead optimization based on receptor structure [63], development of bivalent inhibitors [64], and molecular dynamics of active sites [65]. With this background, our group focused on molecular modeling methods to further advance the understanding of BET inhibition [66].
Following a virtual screening protocol using molecular similarity and docking, two hits were identified. The more promising was amentoflavone (2) (Fig. 1), a biflavonoid produced by Gingko biloba and Hypericum perforatum among other plants, with previous reports of antitumor-related activity [67, 68]. Similarly, other groups identified the flavonoid scaffold as a putative ligand for bromodomains [69, 70]. Yet, this was the first report for biflavonoids, which is interesting due to their atropisomeric properties [71]. In addition, all these studies suggested that flavonoids bind at the ZA channel (a flexible region connecting the Z and A loops). This region has been suggested as significant for selectivity due to its interaction with a conserved water network [72].
Further characterization was performed with molecular dynamics simulations, which showed that amentoflavone (2) can interact with D145, a residue specific to BRD4-BD1 [73]. This is an interesting observation considering that RVX-297 (a quinazolone) is a specific inhibitor of BRD4-BD2 [74]. Biological evaluation of amentoflavone showed an IC 50 in the micromolar range, with evidence suggesting selectivity for BRD4-BD1 [75].

Protein-ligand interactions as obtained from molecular dynamics of BRD4-biflavonoid complexes. (a) BRD4-ochnaflavone, (b) BRD4-taiwanoflavone, (c) BRD4-sumaflavone, (d) BRD4-talbotaflavone
Recently, isothermal titration calorimetry assays have shown that binding in the pocket of BETs is mostly enthalpy driven [76]. This in addition to the flexibility of the ZA channel suggests that constrained structures can show BET selectivity and specificity. This is a notable observation considering the rather “simple” scaffold of flavones. Nevertheless, this shows the undeniable potential of natural products, not just as leads but as pharmacophore templates.
2.1.2 Sirtuins
While not yet discussed in the previous Section on bromodomains, histone acetylation is crucial for chromatin opening. This happens as a result of the recruitment of histone acetyl transferases, and to reverse this process, histone deacetylases (HDACs). The latter are intensively studied to develop novel therapies for several cancer lines, by reactivating silenced genes [77]. Currently, 18 HDAC isoforms are classified into four different classes in regard to their homology to yeast proteins. Class III is the only one for which the function relies on nicotine adenine dinucleotide (NAD+), also known as sirtuins due to their relation to Sir2 [78].
There are seven isoforms of sirtuins in humans expressed at different cellular locations, with highly conserved active sites, but functionally different structures and domains [79]. Recently, it has been shown that sirtuins exert functions beyond epigenetic silencing [80]. For example, sirtuins have an active role in DNA protection and repair by several mechanisms, which include PARP activation, glutamine anaplerosis, reactive oxygen species, and activation of reactive oxygen species neutralizing enzymes [81]. Moreover, sirtuin expression has a direct correlation with caloric restriction. This has been related to extended life span and overall health status provided by NAD+ upregulation [82]. Hence, the investigation of sirtuins becomes quite interesting, as the focus diverges for the search of both inhibitors and activators, according to the effect desired.
One of the first inhibitors of the HDACs was romidepsin (8), a depsipeptide with a disulfide bond and a caged structure, identified from Chromobacterium violaceum [83]. In subsequent studies, it was shown that romidepsin activity was mediated by rupture of the disulfide bond, followed by covalent inhibition of catalytic zinc ions [84]. As a result of this, 8 has pleiotropic effects via pan-HDAC inhibition [85]. Romidepsin (8) has been approved by the FDA for the treatment of T-cell lymphoma [86].
Psammaplin A (11) also contains a disulfide bond, which gives it a potent but nonspecific inhibition of HDACs. Synthesis optimization of this structure led to UVI5008, a compound with the added capacity to inhibit SIRT1/2 [87].
As such, with the off-target effects and nonspecific binding, some researchers have used in silico methods in order to further investigate the inhibition of sirtuins. Early studies focused on splitomicin, an inhibitor of yeast sirtuins. Using molecular docking and molecular mechanics methods, structure–activity relationships were obtained for splitomicin derivatives. These studies provided insight into the rationale behind the activity of (R)-enantiomers of these scaffolds, which were also non-competitive SIRT2 inhibitors [88].
Kokkonen et al. [89] conducted a 3D QSAR study based on SIRT1. Using the CoMFA method a model of significant predictive power was obtained, which resulted in peptide-like ligands for SIRT1 with IC 50 values around 10 μM. Following a subsequent ligand-based virtual screening by Sun et al. [90] using data from public repositories and literature records, 36 representative ligands were selected to obtain binding models using molecular docking. With this model, 12 compounds from Traditional Chinese Medicine were identified as putative ligands of SIRT1. That same year a classic screening of the same database was carried out, identifying four actives out of 19 candidates for SIRT1 activation [91].
A recent study by Karam et al. [92] presented a virtual screening protocol followed by in vitro testing, with a focus on SIRT1, 2, and 3. Using a dataset of African-derived natural products (p-ANAPL), 13 compounds were selected by molecular docking. Seven of these compounds contained a chalcone scaffold with modest activity against SIRT1 and 2. Further modeling showed that the putative binding poses correlate with known crystallographic structures.
Another isoform of interest is SIRT6, as it is related to inflammatory and aging processes. Several studies in mice have shown the importance of this enzyme, particularly its role in cardioprotective mechanisms [93]. Rahnasto-Rilla et al. [94] focused on several flavonoids as putative SIRT6 modulators. The authors of this work used first in vitro screening to identify inhibition/activation of this enzyme. Remarkably, the nature of the modulation was concentration-dependent, with anthocyanidins being identified as effective activators of SIRT6. To gain further insights, molecular docking and in silico residue mutations were carried out, identifying the putative site for activators and the possible mechanism being conformational changes induced by the amino acid residues G156, D185, W186, E187, and D188.
Finally, we discuss the role of sirtuin inhibitors as putative antiparasitic agents. This arises from the phylogenetic characterization of sirtuins, identifying SIR2 homologous enzymes in pathogens, for example, Toxoplasma spp., Plasmodium spp., Trypanosoma cruzi, Leishmania spp., and Trichomonas vaginalis [95]. This opens an avenue for novel therapies of the so-called neglected diseases, as it has been shown that these enzymes have direct relationship with growth and infectivity of pathogens [96, 97].
In this regard, in silico modeling has been used to assess the viability of these macromolecules as potential targets for the treatment of infections. Mostly by homology modeling, studies have suggested that parasitic sirtuins have enough differences from human isoforms to warrant low toxicity [98, 99].
With this in mind, and as a proof of concept, we selected Trypanosoma cruzi Sir2-related protein 3 (TcSir2rp3), as a potential target for the treatment of Chagas disease, and conducted representative virtual screening. Beginning with a homology model for T. cruzi, sirtuin coupled with NAD+, to conduct molecular docking with putative ligands. Also, we focused on flavonoids, due to their background discussed above.
2.1.3 DNA Methyltransferases
Deoxyribonucleic acid may be modified by the addition of methyl groups. This may be conducted over the CpG islands, specifically position 5 of cytosine nucleotides. These regions on DNA are related to gene promoters, so methylation-induced silencing is a recurring feature in most types of cancer [100]. This process involves de novo methylation carried out by the enzymes DNA methyltransferases (DNMTs) 3A and DNMT3B, while “maintenance” is done by the isoform DNMT1. Abnormal function of DNMTs has been related to other malignancies, such as asthma, lupus erythematosus, and myelodysplastic syndrome [101].
An indirect inhibition of DNA methylation, with the use of the nucleotide 5-azacytidine, resulted in re-expression of silenced genes and inhibition of tumor growth [49]. As a result of this, analogs of S-adenosyl methionine and S-adenosyl homocysteine (SAM/SAH, respectively) have been studied to uncover the mechanisms of methyltransferases [102]. Sinefungin, a natural analogue of SAM is a pan-inhibitor of methyltransferases that continues to serve as template for rational design due to the “transition state model” presented earlier [103].
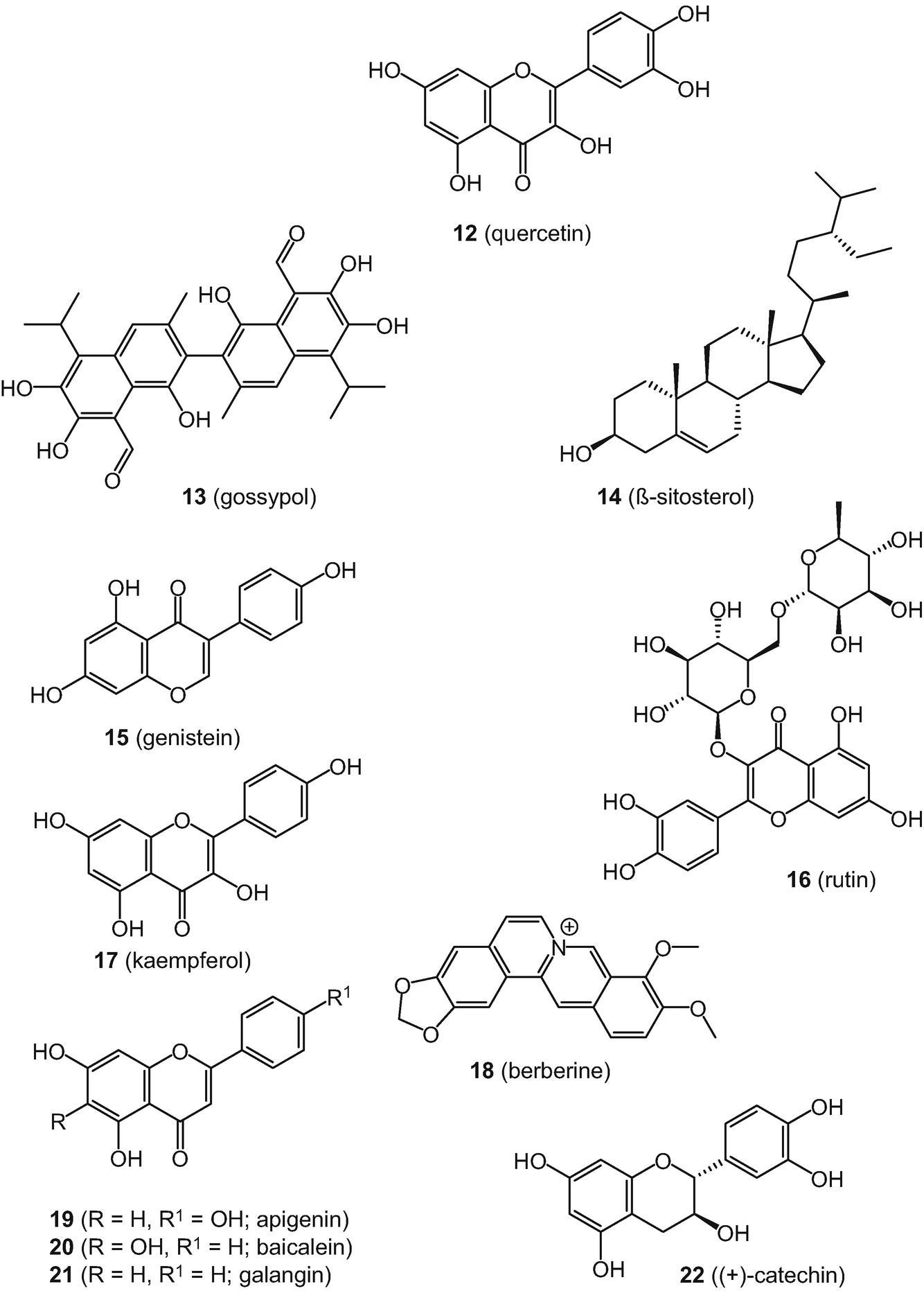
Chemical structures of ten invalid metabolic panaceas (IMPs), a category that also includes curcumin (5)
Using (E)-resveratrol analogs, the study of Aldawsari et al. showed that salicylate moieties provide putative DNMT3 selectivity [107]. By means of molecular modeling and in vitro testing it was assessed that these analogues may have activity independent of SAH, with an increased potency when compared to the parent compound.
Similarly, kazinol Q (9), a hydroxy-chromane derivative, showed antiproliferative activity at 10 μM. Using molecular docking, it was shown that 9 binds to DNMT1 at the SAM site, sharing pharmacophoric traits with epigallocatechin-3-gallate (EGCG), despite the lack of a galloyl moiety [108].
As demonstrated above, natural products continue to offer numerous leads for epigenetic modulation. A focus toward multi-target activity and interdisciplinary research should together continue to uncover other mechanisms such as protein-protein interaction (PPI) modulation. However, the possible toxicity of natural products may still be an issue, as it is a main problem in drug discovery. Hence, in the next section, we address some of the advances and challenges to predict toxicity.
3 Toxicity Profile
Despite the fact that natural products are regarded by the public domain as “safe” because they are “natural compounds” and indeed have been strongly associated with many health benefits, they can contain undesirable, for example, reactive or functional groups. They may also have other toxicological and other properties rendering them not suitable for drug discovery or human consumption such as preservatives or flavoring compounds. Certainly, there are secondary metabolites that are used as pesticides and are toxic.
In drug discovery, calculating or whenever feasible measuring or quantifying experimentally the toxicity profile of chemical compounds is mandatory. In the early stages of drug development, it is common to assess the toxicity related to cytochrome P450 or the human ether-a-go-go-related gene ion-channel (hERG). In later stages, other toxicity endpoints are commonly evaluated such as skin sensitization, potential for genotoxicity and carcinogenicity [109, 110]. This is because many research programs have failed due to toxicity concerns [110]. One of the strategies in order to anticipate toxicity issues is applying commercial, public or in-house algorithms [111, 112]. Indeed, the serious toxicity issues in drug discovery have boosted the need to develop tools to reliably and rapidly predict toxicity endpoints of compounds. Despite the fact that much progress has been made in in silico toxicology, this research area is still under development [110]. In this regard, it is relevant to bear in mind that accurate models become more challenging to develop as the complexity of the toxicity endpoint increases. Complex endpoints are characterized by having various mechanisms of action, that is, due to the interaction of one compound with multiple targets (“polypharmacology”) [113] or the interaction of multiple ligands with the same target (“polyspecificity”) [114], or the combination of both such as the case for certain fragrances (Hernández-Alvarado RB et al. 2019, personal communication). Moreover, the biggest challenge in toxicity modeling is that all chemical compounds are toxic at some level. Therefore, it is expected that a computational approach would be able to predict the type and level of toxicity. As commented by Gleeson et al., the prediction of the absolute toxic potential of a compound, either from in silico or animal models, is very difficult because there are a large number of ways in which toxicity (related to the primary pharmacology or many secondary pathways) can arise [110].
For practical purposes in many current drug discovery projects, structural alerts are used to rapidly identify small molecules that are reactive under common test conditions [115] or are associated with other undesirable properties [116]. These types of compounds have been termed PAINS in the literature (see above). The importance of PAINS structural alerts in natural product research for drug discovery has been discussed extensively by Baell [117].
Examples of recent cheminformatic toxicity-related analysis of datasets of natural products
Study | Outcome | Refs. |
---|---|---|
In silico toxicological screening of natural products | This study compares the predicted vs. experimental toxicity profile for the naturally occurring dietary chemicals: estragole, pulegone, aristolochic acid I, lipoic acid, 1-octacosanol, and epicatechin. It was found that consensus predictions appear to be more accurate than the use of only one or two software programs. In silico results were in agreement with the experimental toxicity data | [118] |
In silico toxicity profiling of natural product compound libraries from African flora | Analysis of the diversity and chemical toxicity assessment of three chemical collections of compounds from African flora. The predictions were done through the identification of chemical structural alerts. It was concluded that only a small fraction of the libraries could have toxicities beyond acceptable limits | [119] |
In silico prediction of the toxic potential of lupeol | Lupeol is a triterpenoid found in many plant species. The interaction of lupeol and 11 of its analogues toward a series of 16 proteins known or suspected to trigger adverse effects was investigated. It was found that there is a moderate toxic potential for lupeol and some of its analogues, by targeting and binding to nuclear receptors involved in fertility | [120] |
Toxicity assessment of natural products from Mexican plants with antinociceptive activity | Assessment of the toxicological profile of molecules with analgesic activity from the UNIIQUIM database. Most of the compounds are likely to interact with opioid receptors. The predicted acute toxicity is low and none is predicted as mutagenic | [121] |
PAINS alerts of a Brazilian dataset and other reference datasets | A large number of molecules in NuBBEDB are promising sources of molecules for medicinal chemistry and drug discovery projects | [122] |
Promiscuity predictions for 208,000 natural products | Predictions of promiscuous compounds with the free online server Hit Dexter 2.0. Overall, flavonoids, in particular chalcones, are predicted as highly promiscuous. In contrast, alkaloids are predicted to be less promiscuous in general | [116] |
A visual representation of 24 ADME (absorption, distribution, metabolism, and elimination)-related properties for a TCM database [123] and natural products from the ZINC database [124] was obtained with principal component analysis (PCA). The so-called ADME space of the natural product collections was compared to a collection of approved drugs, commercial vendor compounds, a general diverse collection obtained from the National Cancer Institute database, and combinatorial collections. It was concluded that TCM covers a vast region of this property space, including areas uncharted by drugs. Natural products from ZINC occupy the same area as drugs [123].
Physicochemical properties along with sub-structural features, for example, functional groups are also used as criteria to filter out compounds with potential toxicity issues early in the drug discovery process. To exemplify this point in recent work, Saldívar-González et al. classified seven natural product collections into six subsets including drug-like, extended drug-like, fragment-like, lead-like, PPI-like, and PAINS [122]. The collections were 2214 compounds from Brazil assembled in the NuBBE database, that is, the first collections of natural products of Brazilian biodiversity, with 473 cyanobacteria and 206 fungal metabolites, 6253 marine natural products, 4103 purified natural product screening compounds, 26,318 semi-synthetic molecules (the last two are commercially available for screening), 17,986 compounds from TCM, and 209,574 molecules in the Universal Natural Products Database (UNPD). Overall, it was found that all seven natural product types had a similar profile except cyanobacteria metabolites. In particular, it was concluded that the NuBBE database had a small percentage of PAINS molecules. In turn, cyanobacteria metabolites had a small fraction of drug-, extended drug-, and lead-like molecules with an increased fraction of PPI-like compounds.
Furthermore, in a recent investigation, Storck et al. profiled approximately 208,000 natural products with a new generation of machine-learning models to identify frequent hitters. The models are freely accessible through the web service Hit Dexter 2.0 [116]. Among the different results, it was found that there was a large percentage of flavonoids (more than 60% of the compounds analyzed) that were found to be promiscuous and approximately 20% highly promiscuous. Of the different flavonoids, chalcones showed the highest rates of promiscuity. In contrast to the predictions for flavonoids, the predictions found by Hit Dexter 2.0 suggested that alkaloids were much less promiscuous [116].
3.1 Privileged or Promiscuous Natural Products?
For some natural products, there is a debate and fine line between highly active or privileged compounds with numerous associated health-related benefits or non-specificity (or high reactivity) [125]. Perhaps one of the most notorious examples in this regard is curcumin (5), a constituent of turmeric (Curcuma longa), a traditional medicine. Curcumin (5) has been classified as both a PAIN [117] and “invalid metabolic panacea” (IMP) compound [126]. Despite the fact there are a large number of reports associating 5 with a plethora of biological activities, there are no conclusive positive results in randomized, placebo-controlled clinical trials for any studied indication as recently discussed by Nelson et al. [127]. Figure 4 shows the chemical structures of nine additional natural products regarded as IMPs in the study by Bisson et al. [126], namely: quercetin (12); gossypol (13); β-sitosterol (14); genistein (15); rutin (16); kaempferol (17); berberine (18); apigenin (19); and (+)-catechin (22) (selected from a list of 39 compounds in total).
3.2 Examples of Toxicity Profiling of Natural Product Databases
As commented above, it is common to evaluate the toxicity related to hERG during the first steps of drug development. Inhibition of this ion channel has been associated with a potentially fatal cardiac arrhythmia, Torsades de Pointes [128]. Several varied experimental tests are routinely used to evaluate hERG inhibitory potential. A number of in silico methods have been developed to assess hHERG inhibition as reviewed by Gleeson et al. [110]. In turn, the Salmonella/microsome assay (Ames assay) is a bacterial short-term test for identification of carcinogens using mutagenicity in bacteria as an endpoint. It is one of the most widely used short-term tests. A high (but not conclusive) association has been found between carcinogenicity in animals and mutagenicity in the Ames assay. Despite the fact there is still controversy over the value of Salmonella/microsome assay results in risk assessment, the results of the Ames assay can provide valuable information to aid in the development of further studies, and may form part of the data, which can be used in evaluating potential biological effects or projected lack of adverse effects [129].
Examples of in silico Ames toxicity and hHERG affinity profiles of six natural product datasets and compared to drugs approved for clinical use
Ames | |||||||
Dataset | Size | Yes | Yes (%) | No | No (%) | NA | NA (%) |
Cyanobacteria | 473 | 11 | 2.3 | 456 | 96.4 | 6 | 1.3 |
Fungi | 206 | 22 | 10.7 | 180 | 87.4 | 4 | 1.9 |
MEG x | 4103 | 333 | 8.1 | 3660 | 89.2 | 110 | 2.7 |
NAT x | 26,318 | 860 | 3.3 | 25071 | 95.3 | 388 | 1.5 |
NuBBE | 2214 | 231 | 10.4 | 1925 | 86.9 | 58 | 2.6 |
Marine | 6253 | 420 | 6.7 | 5700 | 91.2 | 133 | 2.1 |
Approved drugs | 1806 | 156 | 8.6 | 1610 | 89.1 | 39 | 2.2 |
hHERGa | |||||||
Dataset | Size | Yes | Yes (%) | No | No (%) | Inconclusive | NA (%) |
Cyanobacteria | 473 | 8 | 1.7 | 445 | 94.1 | 20 | 4.2 |
Fungi | 206 | 1 | 0.5 | 202 | 98.1 | 3 | 1.5 |
MEG x | 4103 | 53 | 1.3 | 3977 | 96.9 | 73 | 1.8 |
NAT x | 26,318 | 2841 | 10.8 | 21,008 | 79.8 | 2469 | 9.4 |
NuBBE | 2214 | 44 | 2.0 | 2054 | 92.8 | 116 | 5.2 |
Marine | 6253 | 73 | 1.2 | 5924 | 94.7 | 256 | 4.1 |
Approved drugs | 1806 | 243 | 13.5 | 1435 | 79.5 | 126 (+2 empty) | 7.0 |
Regarding the predicted toxicity due to hERG affinity, all six natural product datasets had lower proportions of compounds predicted with high affinity as compared to approved drugs (13.5%). In particular, the datasets with the lowest proportion were fungal metabolites (0.5%) followed by marine and natural products from the commercial screening collection MEGX (1.2 and 1.3%). These results further support that, overall, the six natural product collections can be used as a starting point in drug discovery studies, for instance, in virtual screening to identify potential hits. Of course, the prediction of the toxicity (such as illustrated in Table 3) can be used as a guide to filter compounds for selection.
4 Diversity Analyses of Natural Products
In addition to the applications of computational methods to study natural products, diversity analysis is one of the most classical and useful applications of cheminformatics. In this section, we describe briefly the sources of natural products with emphasis on the public domain. The reader is referred to a recent chapter of Kirchweger and Rollinger [42] for a more in-depth analysis of this topic. We describe the importance of diversity analysis and discuss representative work on cheminformatic-based analysis of the diversity of natural product collections.
4.1 Overview of Collections of Natural Products
Compound collections are a crucial resource for keeping, searching, mining, and sharing chemical information. Currently, there are several compound databases that enable storing and sharing biological screening data. The relevance of chemical datasets to drug discovery projects has been discussed in detail elsewhere [130]. Interestingly, Clark et al. published initiatives in different countries to promote collaboration in drug discovery projects with research groups in academia [131]. In addition to commercial sources of compounds for computational screening, there are publicly available large compound databases annotated with biological activity. Representative resources in this regard are ChEMBL, PubChem, and Binding Database, collectively reviewed by Nicola et al. [132]. Of note, as recently commented by Saldívar-González et al. [122], databases annotated with information of the bioactivity profile against one or several biological endpoints are useful for multiple applications including analysis of polypharmacology and structure multiple-activity relationships [133], characterization of activity landscapes [134] and the reexamination of the currently explored chemical space (vide infra).
In 2012, the first databases of natural products available in the public domain at that time were reviewed by Yongye et al. [135]. Six years ago, there were approximately five databases publicly available containing between 560 and 89,000 molecules. Today, many more databases are available with over 250,000 natural products in the public domain as reviewed in the excellent report of Chen et al. [136]. A significant number of natural product resources are built and maintained by academic groups and non-for-profit initiatives. A classic example is the TCM database@Taiwan [137]. Based on this database, iScreen was developed. This is a web server for docking TCM followed by customized de novo drug design [138]. Another example of a previous academic effort is the development of the UNPD [139]. Unfortunately, at the time of writing UNPD is not available. There are other compound collections that are focused on specific geographical regions. A few examples include the NuBBE database that is a collection representative of the Brazilian biodiversity [140, 141]. In turn, the AfroDb collection [142] is an initiative that collects information on the constituents of African medicinal plants, and contains around 1000 three-dimensional structures. The same group developed the ConMedNP collection [143]. Very recently, the VIETHERB database was made available as a compound collection for Vietnamese plant species [144]. In Mexico, Esquivel et al. are building a comprehensive database of natural products that have been published by the Institute of Chemistry of the National Autonomous University of Mexico (UNAM). This database is called UNIIQUIM (http://uniiquim.iquimica.unam.mx). Another initiative from an academic group of the same institution is constructing the BIOFACQUIM database. Currently, BIOFACQUIM contains 423 compounds mostly isolated from Mexican plants and fungi [14]. A comprehensive review of other natural product collections and resources available to the public has been prepared by Chen et al. [136].
4.2 Design of Nature-Inspired Compound Collections
In addition to existing collections of natural products, compounds of natural origin have inspired the synthesis of natural product datasets. This comes from the apparent, previously mentioned misapprehension using combinatorial chemistry, as the chemical diversity of the collections made was low [11]. To improve this, natural product scaffolds have been suggested as novel means to access uncharted regions of therapeutic and chemical space [9].
For example, Stratton et al. provided a comprehensive comparison of the chemical space of natural products and drugs [145]. This study highlighted the inherent complexity of natural products as the main tool to effectively optimize lead compounds. A similar observation had previously been suggested in a series of studies by Lovering et al. which tackled the issue of molecular attrition, because of low complexity or “flat molecules” as leads [146, 147]. In addition, the use of natural product scaffolds may provide other advantages, such as the improvement of pharmacokinetic properties, intellectual property [148], and even prodrug design [149].
A noteworthy example of a cheminformatics tool to drive biology-oriented synthesis is Scaffold Hunter [150]. Originally envisioned as a visualization tool, it has overgrown its original purpose allowing further types of analysis. A prominent feature is the so-called Periodic Table of Natural Products, which conducts structural deconvolution to provide vantage points for synthesis routes. Successful cases using this method include 11β-hydroxysteroid dehydrogenase, 5-lipoxygenase, phosphatase, and kinase inhibitors [151].
4.3 Concept and Importance of Diversity Analysis
The continued increase in the number of compounds available in compound databases has led to the notion of chemical space [152] and makes necessary to characterize the content and diversity of the molecules stored in those collections. Indeed, comparison of the content and overall the contents of the molecular databases is important in sortiment design and selection [153] as diversity analysis aids in the assessment of the structural novelty of molecules. Systematic analysis of the diversity and chemical space of compound collections, in particular large collections, usually needs cheminformatic approaches [123].
Approaches to assess the diversity of compound databases can be divided into two main groups that largely depend on the molecular representation [14], namely, graphs and descriptor vectors [21, 154]. Graph methods are employed to conduct structural and sub-structural analysis. These approaches are relatively easy to interpret. Representation using descriptor vectors is commonly used in cheminformatics for database processing, similarity searching, clustering, and developing descriptive and predictive models. The choice of descriptors used to analyze compound datasets—with more than five thousand available thus far—gives rise to different types of chemical spaces as pointed out by Varnek and Baskin [154]. The structural diversity of natural product databases using structural fingerprints, molecular scaffolds, and other representation was published in several reports. Analysis of the chemical space of natural product databases has recently been published [14]. In the next section, we will discuss representative studies with emphasis on the diversity analysis that have appeared most recently.
4.4 Representative Diversity Analysis of Natural Products
Representative studies of chemical diversity of natural products
Datasets | Descriptors/representation | Refs. |
---|---|---|
TCM, combinatorial libraries, drugs approved for clinical use, and screening collections | Molecular fingerprints, scaffolds, physicochemical properties | [155] |
Natural products, human metabolites, bioactive compounds, clinical candidates, and drugs | Topological and physicochemical | [156] |
Fragment-sized and no fragment-sized natural products | Pharmacophore and radial fingerprints, and molecular scaffolds | [157] |
Eighteen virtual and nine existing natural product libraries. As reference, the “Dictionary of Natural Products” was used | Physicochemical properties | [136] |
Cyanobacteria, fungi metabolites, marine, purified natural product screening compounds, TCM, NuBBEDB, UNPD. As reference, semi-synthetic and approved drugs were used | Molecular fingerprints, scaffolds, physicochemical properties; drug-, extended drug-, lead-, fragment-, PPI-like, and PAINS profiling; molecular complexity | [122] |
BIOFACQUIM, NuBBEDB, TCM. As reference, approved drugs were used | Molecular fingerprints, scaffolds, physicochemical properties | [14] |
In 2015, Pascolutti et al. published the generation of fragment screening collections that aim to capture the broad range of molecular recognition building blocks included within natural products as included in the “Dictionary of Natural Products” (DNP; Chapman and Hall/CRC Press, Boca Raton, FL, USA). The structural diversity of the fragment versus a reference non-fragment assortment was analyzed using three complementary approaches, namely, atom function analysis (based on pharmacophore fingerprints), atom type analysis (with radial fingerprints), and scaffold analysis. Among the various conclusions made, Pascolutti et al. found that naturally derived fragments could be used as the starting point for building chemical collections with high diversity for medicinal chemistry projects.
Chen et al. [136] reported recently a comprehensive analysis toward the understanding of the population of the chemical space by currently known and accessible natural products and by individual natural product collections. As stated by the authors, among the relevant results of this work was that the easily accessible natural products have a large diversity and cover regions of medicinally relevant chemical space. In some instances, the authors observed a significant difference in the coverage of the chemical space of different classes and individual datasets of natural products.
Saldivar-González et al. reported a comprehensive cheminformatic characterization of seven natural product databases inclusive of cyanobacterial, fungal metabolites, marine, purified natural product screening compounds, TCM, NuBBE, and UNPD databases [122]. As references, a semi-synthetic compound collection and a set of drugs approved for clinical use were employed. The datasets were profiled and compared using a number of different and complementary representations and descriptors, namely, molecular fingerprints of different design (Extended Connectivity fingerprints radius two and Molecular Access System (MACCS) keys), scaffolds, and six physicochemical properties of pharmaceutical interest. In addition, the chemical databases were profiled using empirical rules that have been developed to classify drug-, extended drug-, lead-, fragment-, PPI-like, and PAINS compounds. Finally, the datasets were profiled using two descriptors associated with molecular complexity: fraction of carbon atoms with sp3 hybridization (FCsp3) and the fraction of chiral carbons (FCC). Among the conclusions, it was found that the NuBBE database, the main focus of this work, had a restrained chemical space, with the majority within the region of the drug-like physicochemical properties. It was also concluded that the main source of diversity in the compounds in NuBBE database was driven by the side chains. Overall, the results were supportive of a large number of molecules in NuBBE database being promising sources of lead molecules for medicinal chemistry and drug discovery projects [122].
Recently, Pilón-Jiménez et al. discussed the collection and first diversity analysis of BIOFACQUIM, a database of natural products isolated from organisms in Mexico [158]. In that work, the authors characterize the diversity of BIOFACQUIM using molecular fingerprints (MACCS keys), molecular scaffolds, and six drug-like physicochemical properties, namely, molecular weight, topological surface area, number of hydrogen bond donors and acceptors, number of rotatable bonds and the n-octanol/water partition coefficient, logP. BIOFACQUIM was compared to other natural product and reference databases such as NuBBE, TCM, and approved drugs. It was found that BIOFACQUIM and AfroDb are diverse in terms of scaffolds, but both have relatively low fingerprint diversity. It was also concluded that AfroDb is more diverse than BIOFACQUIM, in terms of relevant physicochemical properties. In contrast, the set of approved drugs had a medium diversity based on fingerprints and relatively low diversity using the scaffolds. In turn, TCM had the largest scaffold and fingerprint diversity, relative to the datasets compared in that work [14].
4.4.1 Global Analysis of Chemical Diversity
As explained above, chemical representation and descriptors are at the core of diversity analysis and basically any cheminformatic application [114]. Therefore, the perception of the chemical space and assessment of the diversity of a compound collection in general is relative to the molecular representation. In order to reduce (although not eliminate entirely) the dependence of the diversity with molecular representation, it has been proposed to use a consensus approach through the assessment of the global diversity using Consensus Diversity Plots (CDPs) [159]. Consensus Diversity Plots are two-dimensional graphs to represent simultaneously four diversities (typically fingerprint-based, scaffold, whole molecular properties—associated with drug-like characteristics, and size of the database). Consensus Diversity Plots have been employed to characterize quantitatively the total or global diversity of fungal metabolites [160], natural products from Panama [161], from Brazil as available in NuBBE [122], and from Mexico (as deposited in the BIOFACQUIM database) [14].
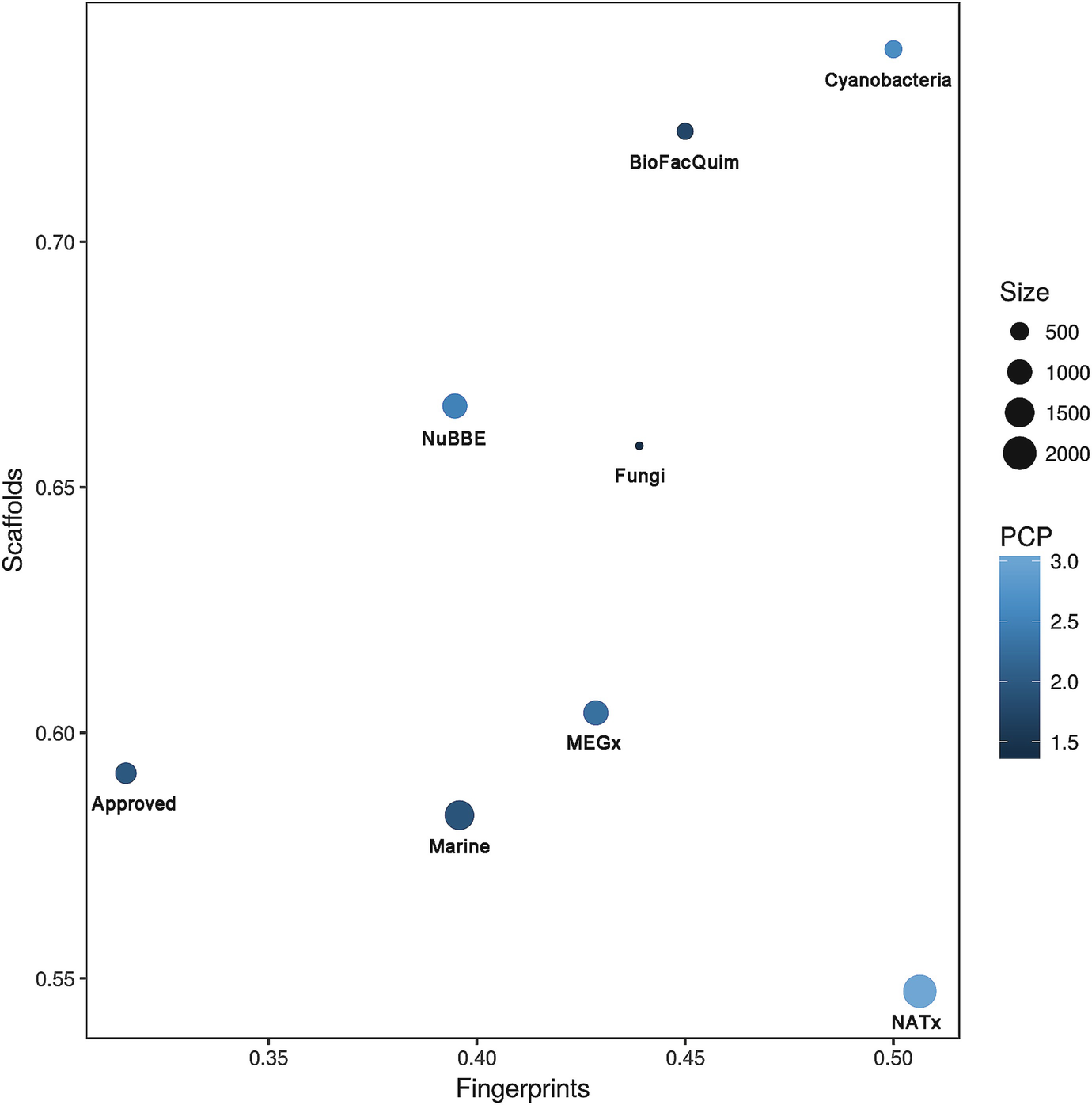
Consensus Diversity Plot comparing the global diversity of BIOFACQUIM with other natural product databases. The structural diversity (fingerprint diversity) was calculated with the median Tanimoto coefficient of MACCS keys fingerprints is plotted on the x-axis. The scaffold diversity of each database was defined as the area under the curve (AUC) of the respective scaffold recovery curves, and it is represented on the y-axis. The diversity based on physicochemical properties (PCP) was calculated with the Euclidean distance of six scaled properties (SlogP, TPSA, MW, RB, HBD, and HBA) and is shown in a color scale. The distance is represented with a continuous color scale from light blue (more diverse) to dark blue (less diverse). The relative size of the dataset is represented with the size of the data point: smaller data points indicate compound datasets with fewer molecules
5 Conclusions and Future Directions
Natural products retain a fundamental role in the drug discovery process, despite the implicit difficulties involved. Nonetheless, the industrial setting has favored other approaches leaving such endeavors to academia. With the emergence of multidisciplinary studies, natural products have seen a renaissance. In this sense, in silico methods provide flexible tools to analyze screens and describe in a qualitative and quantitative basis the diversity, presumptive activity, and even the potential toxicity of natural products.
With several instances of success across different targets, it would seem that natural product research driven by computational methods is “a match made in heaven.” Still, some aspects of computational methodologies cannot be applied “as is,” due to current limitations of the techniques and algorithms. This has had a positive impact in the computational field, stimulating the development of more robust protocols and methods or even a focus toward natural product modeling as a whole. As discussed in this chapter, the availability of new and improved algorithms has led to the development and implementation of a plethora of applications that range from the collection of data to the in silico profiling and screening of natural products. In this sense, the overall projection of computational-based natural product research will continue to thrive, given the increasing number of data sources and the array of metabolites that remain unexplored.
Hence, perspectives on this field regard the construction and optimization of proper databases to enhance fragment-based campaigns and the expansion of chemical space. These include improvement of cheminformatic filters for the identification of activity cliffs.
Acknowledgments
Fernando Prieto-Martínez is grateful for a Ph.D. scholarship from the Consejo Nacional de Ciencia y Tecnología (CONACyT) No. 660465/576637. The authors also thank the Programa de Nuevas Alternativas de Tratamiento para Enfermedades Infecciosas (NUATEI-IIB-UNAM). José Medina-Franco acknowledges the School of Chemistry of the Universidad Nacional Autónoma de México (UNAM), the Programa de Apoyo a Proyectos de Investigación e Innovación Tecnológica (PAPIIT) grant number IA203718, UNAM and the Consejo Nacional de Ciencia y Tecnología grant number 282785. Fernando Prieto-Martínez and José Medina-Franco also thank Dirección General de Cómputo y de Tecnologías de Información y Comunicación (DGTIC), project grant LANCAD-UNAM-DGTIC-335 for the computational resources to use Miztli supercomputer at UNAM. The authors thank Fernanda I. Saldívar-González for providing the datasets on natural products used to compute the toxicity profile, Dr. Sharon Luna for assisting in the analysis of the toxicity data, and Edgar López-López for helpful discussions.