1 Introduction
Small molecule natural products are biosynthesized by biological systems to enable communication and interaction between cells, individuals, and species, serving as repellents, poisons, attractants, and signaling molecules. Owing to their biosynthetic enzyme origin and specific biological purposes, their chemical structures were designed by evolution to interact with macromolecules such as proteins, lipids, and nucleic acids [1–4]. This is in accordance with the finding of increased hit rates of natural product collections compared to synthetic and combinatorial collections in high-throughput screening campaigns [5, 6]. Analysis of such natural product collections revealed an exceptionally high diversity of molecular structures and properties, such as considerable molecular shape, stereogenic and ring-system complexity. They cover a broad chemical space, especially biologically relevant space [7–13] as outlined in detailed chapter “Cheminformatics Explorations of Natural Products” by Medina-Franco et al. in this volume (p. 1).
This makes natural products ideal candidates for drug discovery. Indeed, plants, fungi, and animals were almost the only source for pharmaceutical preparations for a long period of human history. Even with the advent of modern single-molecule medicines, natural products continued to play an important role [14]. A comprehensive analysis by Newman and Cragg points out that still 32% of all small-molecule approved drugs launched between 1981 and 2014 are unaltered natural products or natural product derivatives. Another 32% were inspired by natural products or their pharmacophores [15]. It is therefore tempting to speculate that natural product structures are privileged, possessing particular geometries; for instance, they exhibit a variety of novel, non-flat ring systems suitable for specific side chain substitutions, which are then prone to interact with an array of target proteins [16].
In contrast to the well-recognized high potential of natural products in drug discovery, the research engagement in this field has been dramatically scaled down in major pharmaceutical companies, mainly because it is stigmatized as an expensive endeavor [17]. The process of choosing a suitable biological source, its often limited or restricted access, the successful isolation of single active constituents from complex matrices, and deciphering their molecular structures seem too cumbersome compared to an increasingly automated and straightforward drug discovery process. New technologies like combinatorial chemistry, high-throughput screening using miniaturized and automatized assay batteries, and big data evaluation have triggered a great transformation in drug discovery [18]. The identification of ligands against specific targets as starting points for lead development is of utmost importance in this scientific field [19–21].
A major challenge in drug discovery from natural sources is hereby the identification of single bioactive constituents in order to establish unambiguous cause-effect relationships for later lead development. The classical approach for this task has been the bioassay-guided fractionation of crude or partly purified extracts [22]. Thus, a multicomponent mixture (extract) is separated step by step with subsequent assessment of the biological activities of the fractions obtained, followed by iterative rounds of separation and assaying [23–25]. Ideally, the goal is to end up with a single or a few purified active constituents—a goal which is certainly not often achieved because of certain shortcomings, such as insufficient robustness of bioassays used, potential solute adsorption to the solid phase during chromatographic fractionation, the re-isolation of previously known bioactive compounds, the failure to detect synergistic activity between the components present, and/or the decomposition of the constituents [14, 26].
The reverse path of testing pure natural products after isolation brings up several questions: (1) How to choose the natural starting organism? (2) Which components should be isolated? (3) How to choose a promising target for testing? Some of the most interesting natural products are difficult to isolate and only contained in small quantities in their natural source, for example, the yield of paclitaxel isolated from its source plant, Taxus brevifolia bark, was in the range of 0.01% [27]. Moreover, only 10% of all known natural products can be obtained by commercial suppliers [28], and these sometimes command very high prices. This is one reason their macromolecular targets remain largely unknown [29]. Natural products can be considered as too precious for dissecting their potential bioactivities by trial and error, and a rationale to streamline their biological evaluation is needed.
In this context, the application of in silico tools, in particular, virtual screening, has developed as an important strategy in natural product research for the prediction of ligand-target interactions and for rationalizing their bioactivity or even efficacy on a molecular level. Computational models can be created based upon already available information for the system under investigation and used to make predictions on new events. Without question, cheminformatics-based techniques are nowadays increasingly vital and substantial parts of modern-day drug discovery in medicinal chemistry, in both industry and academia. Their impact in natural product research is also increasing and has been reviewed elsewhere [30–32].
Here, we provide a comprehensive analysis of the strengths, weaknesses, opportunities, and threats (SWOT) of cheminformatics tools in natural product research. The analysis will provide a guide to facilitate their concatenation on the basis of past research projects, and aims to indicate gaps and caveats that exist. Therefore, the outcome of this analysis should give insight into strategic steps for further advances toward the combined use of cheminformatics and natural products drug discovery, to cope expediently with the challenges and opportunities in these two promising and prolific research areas.
2 S: Strengths of Cheminformatics in Natural Product Research
Cheminformatics is the use of computational and informational tools to understand and solve problems in the field of chemistry, particularly drug lead identification and optimization. The intended goal is to make better decisions faster [33]. In particular, virtual screening, which is the use of computational algorithms and models for the identification of bioactivities, has huge potential for more extensive application in natural product research [34, 35].
The implementation of cheminformatic tools can circumvent some of the costly and time-consuming bottlenecks prohibitive to drug discovery from natural sources. From a pharmacognostic perspective, the prediction of molecular properties, possible targets but also antitargets of secondary metabolites, may be extremely useful to streamline experimental efforts, and hence to accelerate research and development projects. The scarce availability of isolated test materials demands for in silico predictions to unravel natural product molecular modes of actions and to deploy a rationale in lead development [32, 36–38]. From a cheminformatics perspective, virtual screening of collections consisting of fewer, but more sophisticated chemical entities, which are designed by evolution to interact specifically with macromolecular targets, rather than large synthetic molecule collections, can be a straightforward and prolific approach for the identification of novel lead compounds. The exploitation of natural product chemistry to implement Nature’s privileged structures and chemical traits into synthetic compound repositories is another important topic [39–41].
- 1.
The availability and access to data providing available information on and the ability to obtain reliable data of the system under investigation
- 2.
Natural product collections including their annotation to meta-data, curation, and a well-analyzed content
- 3.
Availability and applicability of cheminformatic tools for the handling of natural products and specialized software and methods for event prediction
The following sections will provide more insight into the tools and most important databases available or literature dealing with this topic, without intending to provide a complete account.
2.1 Availability and Access to Data
A computational model’s predictive power can be correlated roughly to the state of knowledge for the system it describes. The access to resources such as chemical databases, bioactivity collections, and biological data and a viable linkage and curation of these data is required to perform successful projects [42–44]. Lots of these resources are deposited and freely accessible. Chemical molecular databases with close to a billion virtual molecular entities have been established [44]. In 2017, four big chemical databases, PubChem, ChemSpider, Scifinder, and UniChem, compiled 95, 63, 134, and 154 million chemical structure records, respectively [45].
Biological and biomedical data stored in publicly available bioactivity databases provide a huge amount of detailed information on chemical entities in combination with target proteins, quantitative binding, and bioactivity values. The ChEMBL database [46–48] connects 1.8 million 2D drug-like small molecule structure records with 12,000 molecular targets and 15.2 million bioactivities in an easily accessible interface. The data are derived mainly from seven medicinal chemistry journals (Bioorganic and Medicinal Chemistry Letters, Journal of Medicinal Chemistry, Bioorganic and Medicinal Chemistry, Journal of Natural Products, European Journal of Medicinal Chemistry, ACS Medicinal Chemistry Letters, MedChemComm) and selected articles from 200 journals and certain patents [48]. PubChem has compiled 239.6 million bioactivities for 3.4 million molecules, mainly from high-throughput screening experiments [49, 50]. Chemical patents represent another rich resource of chemical and biomedical information. The SureCHEMBL database aims to make the chemistry annotations of US, EP, WO, and JP patents available in a searchable interface. However, the connected biomedical data are not annotated [51, 52]. A smaller but highly curated database is the DrugBank with 12,000 chemical entries focusing on drugs and related molecules like nutraceuticals. Drug targets, pathways, indications and other pharmacological information are provided [53–55]. A large and comprehensive biomedical database of natural products does not yet exist. The Protein Data Bank (PDB) is a valuable resource for 3D information on biological macromolecules. It archives 144,000 experimentally determined structures and their complexes with metals, co-factors, crystal water, and small-molecule ligands [56, 57].
Biomedical databases
Database | Content | Size | References |
---|---|---|---|
BindingDB | Experimental protein-small molecule binding affinities | 1.2 million binding data for 55,000 proteins and 520,000 drug-like molecules | [59] |
CHEMBL | Data compiled from literature; PubChem and SureCHEMBL | 1.8 million drug-like small molecules 15.2 million bioactivities | |
Drugbank | Highly curated drug data combined with drug target, pathway, indication, and other pharmacological information | 12,000 nutraceuticals, approved and experimental drugs | |
DUD.E. | Active compounds and target affinities, includes widely used decoys in virtual screening | 22,886 actives 102 targets 50 decoys for each active | |
GLASS | Manually curated repository for experimentally validated GPCR-ligand interactions | 342.5 million ligands 3 million GPCR targets | [62] |
GOSTAR | Manually curated SAR database | 6.6 million inhibitors 22 million quantitative SAR points | [63] |
OCHEM | ADME data | 2.8 million property records | |
PDBbind | Binding affinities of PDB entries | 11,000 binding affinities | [60] |
PubChem | Chemical database with bioactivity data from HTS assays | 63 million molecules For 3.4 million molecules 239.6 million bioactivities are compiled | |
Binding MOAD | High-quality PDB subset of ligand-protein complexes | 33,000 structures | |
PDB | Databank of experimentally determined structures of proteins, nucleic acids and complex assemblies | 144,000 experimental determined macromolecule structures | |
SMPDB | Interactive and visual small molecule pathway database | 30,000 human pathways | |
TTD | Database of therapeutic targets | 3000 targets | [71] |
Chemical, biomedical, and other life science data can be estimated to grow further in the future as the integration of chemical information from multiple sources and analytical techniques, extracting and mining information from journal articles and patents is still improving. Collaborative efforts and the commitment to make generated data available in the public domain will stimulate this development.
2.2 Natural Product Collections
A prerequisite of conducting cheminformatics in natural product research is the existence of stereochemically well-defined molecules. Appropriate commercial and also free natural product databases are available. These important resources have been reviewed several times [28, 72–77].
The most comprehensive database is the Dictionary of Natural Products (DNP) with currently 260,000 natural products. Information on trivial names, physicochemical properties, and toxicity data are supplied. For pharmaceutical biologists the information on biological sources and experimental properties such as UV spectra and dissociation constants can be very useful. Caution should be given when used for 3D applications, because the stereochemistry is not annotated in the 2D connection tables. The database was built manually by a team of academics and freelancers, who enable reconciling of errors and ensure high quality data [28, 78]. Although this database is comprehensive and well curated and covers a large chemical diversity, its availability only on a commercial basis hampers its broader use by the interested scientific community.
Alternatives are free virtual natural product collections, like the Universal Natural Product Database (UNPD) , the TCM database@Taiwan, NPCARE, and the NuBBE database; these all have been made available free of charge [79–83]. Chen et al. recently have analyzed the content of natural product collections and observed a large overlap (108,000 molecules) of free virtual natural product collections with the DNP [28]. A thorough survey on natural product resources and their characteristics is provided in the chapter “Resources for Chemical, Biological, and Structural Data on Natural Products” by Kirchmair et al. in this volume (p. 37).
The use of cheminformatics tools to select natural products and natural product like compounds from large chemical (e.g., PubChem [50]), biomedical (e.g., ChEMBL [47], PDB [57]) or commercial vendor databases (e.g., ZINC [84], Aldrich Market Select [85]) would be a worthwhile strategy. Several tools able to identify natural products in large molecule sets have been developed. They are based on different machine learning tools such as rule-based approaches, similarity measurements of structural space, or connectivity fingerprints [86–91]. Recently, a random forest classifier with high accuracy was made available in a free online tool [92].
The diligent exploitation of natural product resources from widely unexplored organisms from different niches of our globe and the closer examination of already investigated marine and terrestrial organisms by advanced technical means will continue to extend the diversity and coverage of natural product collections. The exchange of virtual physically available collections between cooperation partners has been suggested to increase the access to natural products [93]. Efforts to compile, annotate, analyze, and finally enable their availability to a broad community will lead to an increasingly valuable resource for future drug discovery.
2.3 Applicability of Cheminformatics Tools
As summarized earlier, scientists have to learn from the vast amount of biomedical data generated and made available via data-sharing platforms. However, it is indisputable that the amount of data is far beyond traditional analysis and learning [94]. To create predictive cheminformatic models from big data, various approaches have been established ranging from comprehensive similarity measurements (e.g., pharmacophore, shape-based approaches, physicochemical property comparison) to complex molecular docking and sophisticated machine learning approaches (e.g., self-organizing maps). The basic concepts underlying these methods have been reviewed elsewhere [30–32, 95].
Approaches for the prediction of natural product binding modes
Technique | Software | Targeta | Target classb | Examples |
---|---|---|---|---|
Molecular docking | Ligandfit | HR | V | [96] |
GOLD | NA | V | [97] | |
5-LOX | E | [98] | ||
COX-2 | E | [98] | ||
11β-HSD1 | E | [99] | ||
Glide | MD-2 | PPI | [100] | |
5-HT2C | GPCR | [101] | ||
MOE | PPARγ | TF | [102] | |
Autodock | AChE | E | [103] | |
Autodock Vina | NF-κB | TF | [98] | |
CDOCKER | PPARγ | TF | [104] | |
Molecular dynamic simulation | AMBER | NA | V | [97] |
MD-2 | AG | [100] | ||
DNA | DNA | [105] | ||
AChE | E | [103] | ||
NAMD | NF-κB | TF | [98] |
Different approaches for the prediction of natural product molecular targets
Technique | Strategy/software | Examples |
---|---|---|
Artificial neural networks | Self-organizing maps, e.g. [106] | |
Hierarchical clustering | Based on in silico retrobiosynthesis [108] | [109] |
Virtual parallel screening | Ligandprofiler, PipelinePilot, Ligandscout, Catalyst | |
Reverse docking | Autodock Vina |
Different/complementary virtual screening approaches applied to natural products
Approach | Strategy/software | Targeta | Target classb | Examples |
---|---|---|---|---|
Pharmacophore-based virtual screening | Catalyst | AChE | E | [114] |
COX-1, COX-2 | E | |||
HR | V | [96] | ||
hERG | IC | |||
FXR | TF | |||
cPLA2α | E | [121] | ||
mPGES-1 | E | [122] | ||
IKK-β | E | [98] | ||
mGlu | GPCR | [123] | ||
PrPC | V | [124] | ||
Ligandscout | AChE | E | [114] | |
hERG | IC | |||
GPBAR1 | GPCR | [125] | ||
11β-HSD1 | E | |||
PPARγ | TF | [127] | ||
CETP | LTP | [128] | ||
PharmaGIST | AMA1-RON2 | PPI | [129] | |
MOE | PPARγ | TF | [102] | |
TbGAPDH | E | [130] | ||
2D similarity search | chemGPS [7] | Antichlamydial | – | [131] |
Connectivity fingerprints | FXR | TF | [132] | |
3D similarity search | ROCS | GPBAR1 | GPCR | [125] |
NA | V | [133] | ||
IKK-β | E | [134] | ||
SQUIRREL | mPGES-1 | E | [135] | |
Phase | HIV-1 RT | V | [136] | |
Molecular docking | Autodock | Complex III | E | |
NA | V | [139] | ||
AMPK | E | [140] | ||
Autodock Vina | ROCK1 | E | [141] | |
Complex III | E | |||
GOLD | AChE | E | [142] | |
CK2 | E | [143] | ||
Glide | HIV-1 RT | V | [136] | |
CK2 | E | [143] | ||
FXR | TF | [132] | ||
PPARγ | TF | [144] | ||
Sirt1 | E | [145] | ||
ACE | E | [146] | ||
LigandFit | mGlu | GPCR | [123] | |
CDOCKER | PrPC | V | [124] | |
MOE | CK2 | E | [143] | |
TbGAPDH | E | [130] | ||
Molsoft | TNF-α | PPI | [147] | |
DNA | DNA | [148] | ||
DOCK | AMPK | E | [140] | |
ROCK1 | E | [141] | ||
QSAR | GP regressionc | IRF-7 | TF | [149] |
Multiple linear regression | Antitrypanosomal | – | [150] | |
Machine learning | Self-organizing maps | AChE | E | [142] |
Random forest classifier | AMA1-RON2 | PPI | [129] | |
GP regressionc | PPARγ | TF | [151] |
Perhaps the most important cheminformatics application for natural product researchers is the prediction of molecular targets as thoroughly reviewed in the chapter “A Toolbox for the Identification of Modes of Action of Natural Products” provided by Rodrigues et al. (this volume, p. 73). Besides virtual target fishing of new isolates, it can help to fast forward the rationalization of traditionally used herbal remedies, the prediction of side effects, and the profiling of polypharmacologic actions [29, 30, 110, 112, 152]. The experimental validation of the target-predicting approaches is usually demonstrated on single molecules or only on few examples rather than on a large set of natural products [36, 108, 113], mainly owing to the major effort necessary for experimental testing and the limited physical availability of compounds.
The benefit of experimental testing based on virtual predictions compared to serendipitous experimental screening could be demonstrated convincingly by Doman et al. [153]. Their random in vitro screening for protein tyrosine phosphatase inhibitors revealed a hit rate of 0.02%, while assaying the virtually predicted hits yielded a hit rate of 34.8%. In general, the first evaluation of virtual hits does not require any physically available material but requires a critical check on various parameters before compounds are selected as candidates for experimental testing, e.g., availability; isolation efforts; physicochemical parameters referring to PAINS or inappropriate absorption, distribution, metabolism, excretion, and toxicity (ADMET); reported toxicity; and reliability of predictions [72, 30]. Rare biological material and precious isolates can be saved, and fewer bioassays are needed for the identification of active hits.
Computer-aided techniques have shown to be applicable to many natural product scaffolds such as polyketides [109], alkaloids [37, 118], coumarins [111, 125], flavonoids [133], and sesqui- and triterpenes, [99, 126, 150], and they have been used to make predictions on many biological drug target classes and phenotypic effects.
The concatenation of cheminformatics tools in combination with pharmacognostic expertise and complementary empirical knowledge, such as information from traditional medicine, in vivo studies, epidemiological or clinical investigations, bioassay-guided fractionation, and high-resolution mass spectrometry-based dereplication is able to dramatically enhance the true positive hit rates as discussed in Sect. 4.2 [116, 117].
The ever-increasing computing power and availability of augmented data analysis algorithms have led to a broad use of computational tools in drug discovery. Even big data quantities can be processed with increasingly clever algorithms. Moreover, some predictive methods have shown similar performance levels to a group of experienced medicinal chemists in predicting biological activities, and outperform the brains of experts in the ability to process large databases [154].
3 W: Weaknesses of Cheminformatics in Natural Product Research
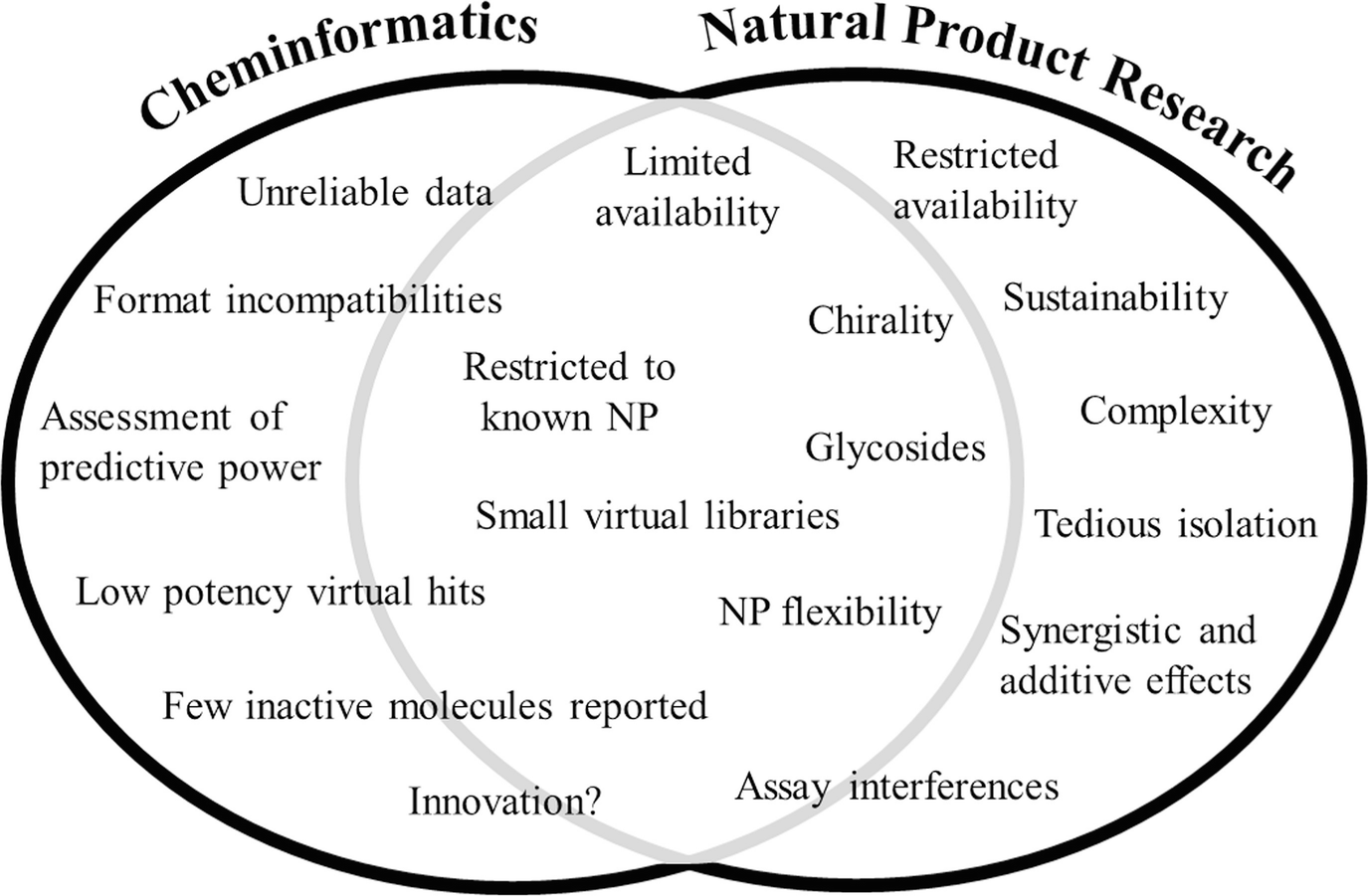
Weaknesses and challenges in cheminformatics, in natural product research, and at the interface of these two fields
The limited availability of natural starting material [155] and of readily available natural products by commercial vendors [28], the absence of elucidated molecular structures for the vast majority of natural products that exist, in addition to assay interference [156], are examples of drawbacks with respect to natural products. The complexity of multicomponent mixtures with difficult-to-predict additive effects and separation problems in isolation efforts are further caveats.
In the field of cheminformatics, there are recommended reviews on the pitfalls of virtual screening [157] also in combination with natural product research [72, 30]. The most fatal weakness of cheminformatics approaches is that they have an inherent incapability to find novel compounds or novel molecular mechanisms of action. They can just extend knowledge on existing topics; the predictive power is better the more knowledge is available already for the system under investigation. An investigator has to navigate on the one hand between innovation usually combined with interesting but ambitious topics with few relevant data available, and, on the other hand, probably trite, less risky targets with good prospects of success due to a vast amount of information already available. A number of molecular mechanisms have been explored by means of natural products, and some biological targets have even been named by their natural product ligands, as exemplified by the muscarinic acetylcholine receptor and cannabinoid receptors. Therefore, in silico tools should be used as part of an interconnected network combined with empirical knowledge and phenotype-directed and target-directed screening platforms [38, 158].
3.1 Structural Complexity of Natural Products
A main weakness appearing upon the handling of natural products with computational algorithms is the difference between natural products and synthetic small molecules [35], which was previously analyzed by several groups [11, 12, 76, 159–161]. Most algorithms were trained on synthetic molecules and might perform less well when they are confronted to unfamiliar molecules [35].
Natural products differ from other compound sets in several molecular properties. They are more hydrophobic and contain more oxygen atoms and fewer nitrogen atoms compared to synthetic drugs. The structural complexity, especially the differences in ring architecture with unsaturated ring systems and more three-dimensional molecular shapes but less aromaticity is, on the one hand, closely correlated to the concept of privileged structures but may cause a difference in performance [161].
Natural products are more flexible due to high numbers of sp3 hybridized atoms making computations with three-dimensional tools (e.g., molecular docking) and conformational sampling for 3D similarity searches or pharmacophore-based virtual screening slower and more error-prone. A large number of rotatable bonds can also lead to promiscuous results, where ligands are fitted to molecular shapes, pharmacophores, and molecular docking in implausible ways. Rotatable bond filters for shape matching experiments like the suggested Veber rule (rotatable bonds <12) [162] can be applied.
A characteristic of natural products is the frequent occurrence of one or even more chiral centers [11, 76, 160], which are not always annotated in natural product databases or catalogs of chemical vendors [78]. Moreover, the exact configuration is not always reported in the primary literature. The generation of all stereochemical configurations is time-intensive and error-prone.
Projects are more likely to be successful if the input information is related to the test subjects. Screening of natural product collections with a synthetic molecule query may be problematic concerning the reliability of the prediction. Similarly, the screening of synthetic molecule collections with a natural product-like query may lead to disappointing results. It is obvious that different ligands can occupy different regions on the same protein, even in the same binding site, making 3D alignments like pharmacohore- and shape-based screening prone to high rates of false-negative results [157].
3.2 Handling of Glycosides
Glycosides play an important role in living organisms and are abundant moieties of natural products with different biological roles. Glycosides like amygdalin are used by different plants as storage and transport forms of their aglycone molecules. Upon disruption of compartments (e.g., by grazing herbivores), enzyme hydrolysis cleaves the glycosides and sets free the toxic aglycone. Other glycosides are natural prodrugs, enabling improved drug-likeness of the transformed metabolites [163, 164].
At first glance and from a medicinal chemistry perspective, sugars and sugar-like moieties are not in the focus of drug discovery. They are easily cleaved in the gut by microbes or by first-pass metabolism, increase the molecular weight, and lead to steric hindrance. Further, the polar glycoside moiety hinders the lipophilic effect between protein and ligand. Therefore, algorithms were created to cleave sugars from their aglycone counterparts for creation of virtual screening databases [28, 165].
The molecular docking force field was adjusted to the binding of comparably rigid and nonpolar molecules and performs therefore well on such molecules; however, the performance with carbohydrates and carbohydrate-containing molecules is questionable. The frequently used molecular docking tool Autodock Vina was able to produce acceptable structures within the top five ranked poses in only 55% of experimental crystallographic carbohydrate-protein complexes [166].
Notably, glycosides have been important drugs for a long time. In herbal medicines, it is acknowledged that glycosides decrease capillary fragility and exert secretolytic, diuretic, and antiexudative effects [167–169]. Carbohydrates play important biological roles such as cell signaling, infection, and protein function [170–172]. These effects are mediated generally by nonclassical modes of action such as membrane activity and interaction with protein surfaces yet difficult to describe with algorithms [173–175].
There are also examples of classic ligand-target interactions with natural product glycosides. Thus, phlorizin, a dihydrochalcone derivative, was the blueprint for sodium-dependent glucose transporter 2 inhibitors. The sugar moiety of phlorizin represents a vital part of the necessary pharmacophore to block the transporter [176]. From perspectives such as this, it may be a fallacy to exclude glycosides from virtual screening databases.
The handling of glycosides may be dependent on the individual target and project but definitely needs consideration. Further improvement of virtual screening tools toward a better applicability for glycosides is certainly needed.
3.3 Tiny Databases

Amounts of purchasable compounds in virtual collections on a logarithmic scale; white, natural product; black, primarily synthetic molecules; CA-NP, commercially available natural products; AMS Aldrich Market Select
Model rigidity has to be balanced according to the size of the databases screened. Assuming a restrictive model with a hit rate of 0.2% will lead to estimated 50 virtual hits from commercially available natural product databases and 16,000 virtual hits from commercially available synthetic molecules.
Natural product chemical diversity, however, is insufficiently explored and is biased toward molecules from extensively exploited sources making a final statement on their extent speculative. This is underlined, for example, by the discovery of naturally occurring organohalogens, which were considered until quite recently as rare and exotic isolates and often suspected to be artifacts. With the exploitation of unexplored sources such as marine organisms, algae, and lichens, thousands of these have been described [177]. Also, improved isolation and analytical methods, which enable the characterization of natural products contained at even lower traces, constantly change our perception of natural products chemistry.
Two main issues in future will be to continue the present rate of natural product discovery and to properly exploit what is found [178].
4 O: Opportunities of Cheminformatics in Natural Product Research

Opportunities and areas of applications of cheminformatics in natural product research
4.1 Virtual Screening of Natural Product Databases
When considering the innate character of natural product collections (prolific, but low number of entities, difficult availability, high cost to obtain, etc.), as discussed in the previous chapters, it is highly recommended to first validate the predictive power of the model used by experimental testing of a set of virtual hits from easily accessible and inexpensive, physically available (synthetic) databases. Also, a proper preparation of the database subjected to virtual screening, e.g. by pre-connected filtering experiments may help to (1) focus on the most interesting candidates and (2) economize computational power.
For example, Su et al. prepared a virtual screening collection with fingerprint clustering and drug-likeness filters. Natural products unsuitable for the molecular docking algorithms due to their size and polarity could be removed in advance. The virtual screening of only 24,000 molecules with a stepwise workflow employing molecular docking led to the identification of baicalein and phloretin as new natural Rho kinase inhibitors [141].
Considerable database preparation was also performed by Costa et al. for the identification of HIV-1 reverse transcriptase inhibitors. They generated a natural product database from 11 vendors and natural product databases publicly available in the ZINC repository. They narrowed down the database by removing molecules violating the Lipinski Rule of Five [179], and with predicted poor solubility and permeability. A parallel molecular docking protocol as well as a 3D similarity search led to the selection and experimental testing of several virtual hits. β-Carboline derivatives were identified as HIV-1 reverse transcriptase inhibitors and their binding mode was examined using the molecular docking predictions as well as molecular dynamic simulations [136].
Insufficient capacities to obtain large sets of natural products for experimental testing may be circumvented by the application of a set of ligand-based pharmacophore models previously validated mainly on synthetic molecules for the most prevalent antitarget in drug discovery and development, i.e., the hERG channel [38, 117, 118]. For a detailed insight into the performance of different hERG prediction tools toward a fast and efficient cardiotoxic risk assessment, reference is made in the contribution in the chapter “Open Access Activity Prediction Tools for Natural Products. Case Study: hERG Blockers” by Schuster (this volume, p. 175). Kratz et al. used the previously generated, best performing pharmacophore model for the subsequent virtual hERG screening of natural product databases. They validated their predictions in a patch clamp assay by testing small-scale lead-like enhanced extracts from 12 plant materials known to contain the virtual hits. At 100 μg/cm3, 4 out of the 12 extracts exerted a hERG tail current inhibition of more than 30%, among them Ipecacuanhae Radix. Use of an appropriate phytochemical workflow resulted in the isolation and identification of five out of the six virtually predicted alkaloids, among them the major constituents emetine and cephaeline with IC 50 values of 21.4 and 5.3 μM, respectively [118]. Similarly, Vuorinen et al. [126] used pharmacophore models for the identification of hydroxysteroid dehydrogenase inhibitors from Nature using previously validated models [180, 181].
Virtual screening can also predict phenotypic efficacy as shown by work of Karhu et al. [131]. They performed a principal component analysis [7] of the physicochemical properties of a natural product database and an antichlamydial reference set and compared the Euclidian distances in the chemical space. Out of 26 virtual hits, 6 molecules were confirmed as active and 1 high-potency lead was identified.
4.2 Exploitation of Pharmacognostic Knowledge
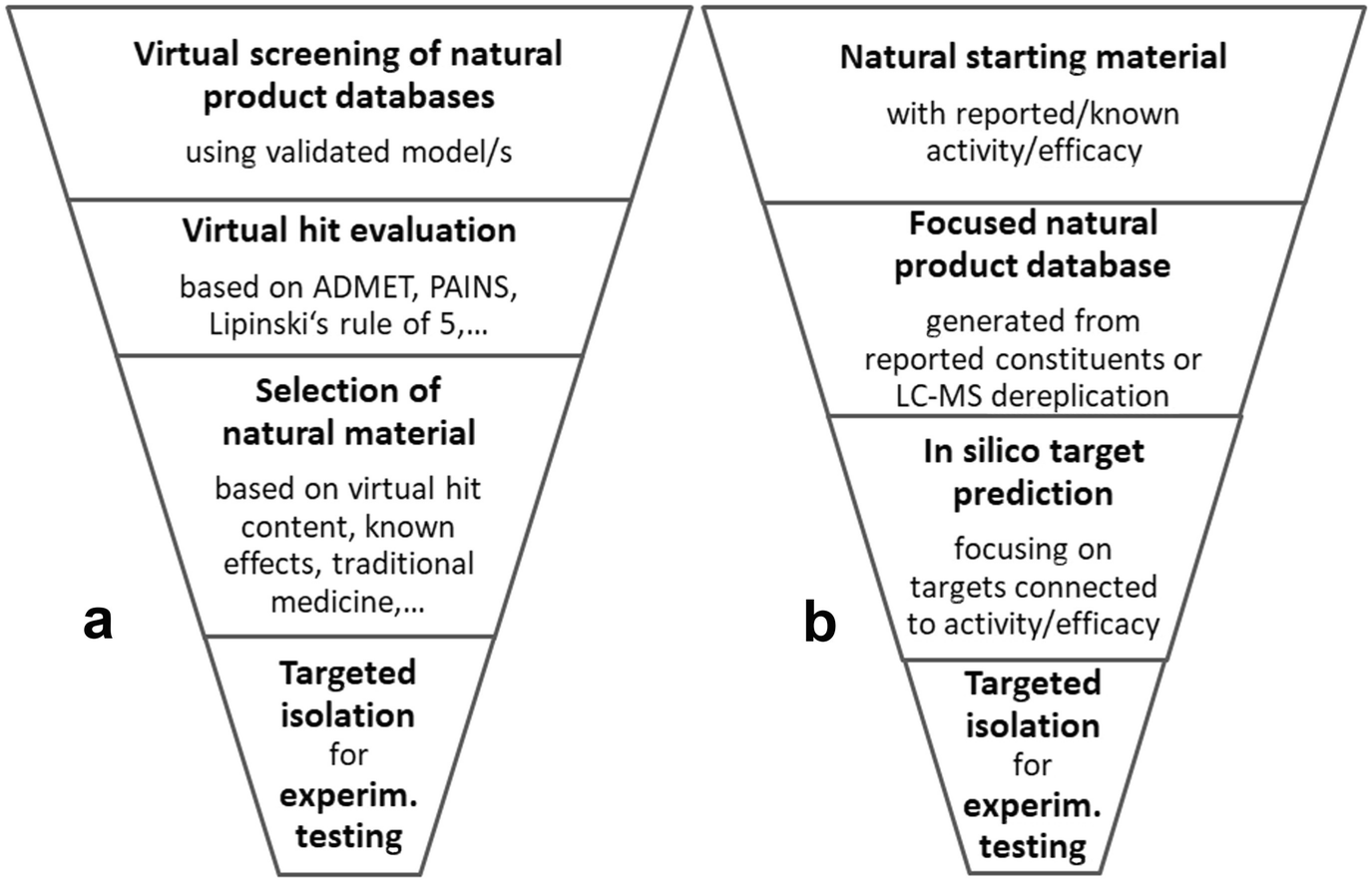
Examples of strategies for the implementation of cheminformatics in pharmacognostic workflows: (a) starting from validated in silico model/s; (b) starting from bioactive natural material
Kirchweger et al. [125] performed a virtual screening of several small natural product databases and a larger synthetic small molecule collection (SPECS) for the identification of activators for the G protein-coupled bile acid receptor 1 (GPBAR1) using a ligand-based pharmacophore virtual screening approach. The virtual hits were ranked according to a shape-focused similarity score and the molecules were clustered according to their physicochemical properties. This approach enabled the selection of chemically diverse compounds endowed with the putative structural requirements to act as ligands of the envisaged target for experimental validation using a reporter-gene based assay. Both synthetic and natural product-derived virtual hits were subjected to experimental testing. Accordingly, the yield of active synthetic compounds (>15% receptor activation at 20 μM) was 10.5% (2 out of 19); natural products resulted in a five-time higher hit rate (57%; 8 out of 14). The latter group also included two novel GPBAR1 activating scaffolds, namely, the sesquiterpene coumarins farnesiferol B and microlobidene, which at 20 μM increased the receptor activation to 61% and 84%, respectively, thus showing an activity comparable to that of the endogenous ligand, lithocholic acid.
Cheminformatics can also be used in a straightforward manner for the identification of active principles of traditionally used medicines and unravel their molecular modes of actions. Schuster et al. [120] generated a set of validated pharmacophore models for the transcription factor FXR, a drug target for inflammatory liver diseases [182]. Grienke et al. [119] used this model for virtual screening of the Chinese herbal medicine database, and, from this work, lanostane-type triterpenes from the fruit body of Ganoderma lucidum were predicted as virtual hits. As this mushroom is traditionally used against hepatitis, liver disease, and arthritis, a full mycochemical investigation and isolation was performed. Five isolated lanostane triterpenes were confirmed experimentally to induce FXR activation with EC 50 values in the low micromolar range.
4.3 Virtual Target Fishing
It is a frequent observation that a herbal drug shows a well-documented biological or clinical effect, but the constituents responsible as well as their underlying mechanisms of action remain elusive [95, 108]. Binding mode prediction and virtual target fishing can help to fast forward the rationalization of research and identify possible drug leads. Similar to already described nutritional and medicinal effects in humans, an observed phenotypic effect such as cytotoxicity, antimicrobial, or hypoglycemic activity can be followed up with focused isolation and experimental efforts.
In 2014, Reker et al. [29] presented a novel method for target fishing, which is independent of the target structure. The approach uses topological pharmacophore features of query compound fragments to compare them to pre-calculated drug compound clusters. The constituent can then be assigned to the cluster with the smallest Euclidian distance. Target information for the cluster was derived from confirmed interaction partners of reference drugs within the cluster. As a prospective application example, the macrolide archazolide A (ArcA) was investigated. This compound exerts potent cancer-related effects by inhibiting the ion pump vacuolar-type H+-ATPase at the nanomolar level. However, it was suggested that additional targets might be responsible for the pronounced antitumor effect. The analysis predicted several targets involved in arachidonic acid-associated signaling cascades as potential interaction partners, and subsequent biological testing confirmed a concentration-dependent effect of ArcA on half of these targets. In addition, weak effects on two further targets were observed. The experimental results validated the applicability of the natural product-derived fragment-based approach for the identification of novel macromolecular targets. Remarkably, all newly identified interaction partners of ArcA have also been linked to putative anticancer effects [29].
Mastic gum has been used traditionally against metabolic disorders [183] and has also shown to exert a hypoglycemic in vivo activity [184]. Its bioactive constituents and the molecular targets responsible were largely unknown. The virtual screening of a natural compound database against 11β-HSD1 pharmacophore models retrieved triterpenoids from Pistacia lentiscus as virtual hits. Together with empirical and preclinical data, the prediction seemed plausible. Therefore, mastic gum and its acidic fraction, which is known to contain the predicted hits, were subjected to experimental testing. Both samples inhibited 11β-HSD1 in a concentration-dependent manner; the two virtually predicted main triterpenes showed IC 50 values in the low micromolar range [126].
Gong et al. [112] used a similar approach based on reverse docking against 211 cancer-related targets to explain an observed cytotoxic effect against two cancer cell lines of two novel sponge metabolites. The precious isolates were only tested against the two most promising targets according to the docking scores. The experimental testing explained the phenotypic effects as attributed to the inhibition of histone acetyltransferase h(p300).
Several target prediction tools have been made accessible online such as the self-organizing map-based prediction of drug equivalence relationships (SPIDER) [106] and the Antibiotic'ome [108].
4.4 Binding Pose and Activity Predictions
If a broad set of structurally very similar molecules and their biological activity in a certain assay is well described, quantitative structure-activity relationship (QSAR) models can be calculated. Schmidt et al. used the information on 69 sesquiterpene lactone structures and their antitrypanosomal activities to generate a predictive model. The query was able to predict correctly furanoheliangolides with highly potent antitrypanosomal in vitro activity out of a virtual sesquiterpene database [150].
Molecular docking in combination with molecular dynamic simulations but also pharmacophore alignments have been demonstrated to accurately predict the binding mode of natural products to their respective targets offering valuable support for the understanding of bioactivities on a molecular level. Rollinger et al. used a combination of molecular docking and pharmacophore-based virtual screening to identify experimentally novel inhibitors of the human rhinovirus (HRV) capsid binders and to give insights into the interaction of natural product-derived inhibitors in the binding pocket. They proposed an eight-feature pharmacophore necessary for the identified ligands interacting in the binding site in addition to their fitting and binding mechanism into the highly lipophilic pocket [96].
The structure and function of membrane-bound GPCRs is still not well understood due to their difficult crystallization. Binding mechanisms of their ligands are nevertheless crucial, since approximately one third of all drugs target these proteins. After identifying several alkaloids as 5-HT2C receptor ligands with a combined virtual and experimental screening, Peng et al. used a homology model to predict the interaction pattern of the ligands. Molecular docking and molecular dynamics suggested key interactions such as a conserved salt bridge and π stacking [101].
5 T: Threats of Cheminformatics in Natural Product Research
At first glance, the broad use of natural products in the field of cheminformatics should not lead to overestimated perceptions. As outlined in Sect. 3 weaknesses are pervasive and experiments are mandatory for confirmation of results. However, commonly, this is not the case for binding mode predictions, which frequently are reported without any proof of correctness.
Molecular target prediction tools are similarly hard to evaluate experimentally and natural product researchers should scrutinize retrieved predictions with healthy skepticism. The biomedical data for natural products is comparatively small when compared to other molecule classes. Therefore, it must be assumed that they are generally underrepresented in generation and validation of computational models. This might not only be the case for target prediction but also for the estimation of lipophilicity, conformer generation, assay interference prediction, molecular docking force-field adjustment, and other tasks.
In silico models must follow scripted instructions and generate only predictions. Flexibility, dynamics, entropic issues along with many more aspects can only be approached with extensive computational efforts. Virtual screening experiments still produce many false-positive virtual hits and incorrect or distorted results. Accordingly, predictions without any solid and unbiased experimental validation are not able to stand any test of scientific meaningfulness and therefore have to be regarded as “preliminary.” On the other hand, even if experimentally validated, the probability of not being able to gain access to information of experimentally proven wrong hypothesis/models is very high. This not only refers to models that failed a proof of concept but also to test data of compounds showing no activity on a specific target. With special regard to the correct feeding and training of prediction tools with structural data covering a broad range of activity, ideally from inactive compounds to highly potent ones, learning from previous mistakes and non-working hypotheses would be extremely valuable. The fact that so many successful projects have been reported disguises the fact that other projects failed.
The availability of natural products in sufficient purity from commercial suppliers or obtaining these by isolation from a suitable natural source can be very costly or time-intensive. The natural starting material should be accessible and legally available for collection/acquisition considering issues on bioprospecting, intellectual property rights, and transfer of natural material to the outside its country of origin (Nagoya protocol, [155]). Also reliable reports on the natural product isolation procedure as well as compound structure elucidation parameters and the description of relevant physicochemical properties should be accessible for a target-oriented re-isolation and identification using mass spectrometry-based dereplication.
Special attention should be devoted to broadly distributed PAINS motifs in natural products such as catechols, hydroquinones, epoxides, peroxide bridges, and phenolic Mannich bases. Other concerns are solubility problems and compound aggregation. However, it might be inadvisable to generate a naive black-box application of PAINS and general drug-likeness filters [156] without looking beyond these parameters.
6 Conclusion
The process of small-molecule drug discovery can be described as being deterministic and nonlinear (e.g., activity cliffs) resembling a chaotic system. This is particularly true for drug discovery from natural products, where researchers are confronted along with nonlinear behavior, serendipitous events, errors, and incompleteness also from biological variance, complex multicomponent mixture interactions, and frequent assay interferences. The current challenge of medicinal chemists is to choose which of the possible 1060 drug-like molecules should be synthesized and tested [18]. Considering the historical impact of natural products on the pharmaceutical arsenal and their infinite (however, incompletely known) diversity, secondary metabolites have already been synthesized by the most trained chemist on earth and thus are hidden gems designed to have key functions. In natural product research, the application of cheminformatics-based strategies is limited to already structurally disclosed molecules; accordingly, their potentially very large impact relies on properly performed and trustworthy chemical studies on natural resources and their constituents and their documentation and dissemination.
The technological advances and experimental exploration of the last centuries, in particular, have afforded the opportunity of accessing enormous amounts of data. Selecting the appropriate computational tools for handling these data and for addressing the research question is a key step but still requires a healthy skepticism and an unbiased attitude.
The Nobel Laureate Rolf Zinkernagel once made a piercing summary of different research strategies and their chance for success [185]: Having no rationale and performing no experiments is cheap but will not lead to results. To start from a rationale, but renounce experimental work is another relatively cheap method, but similarly does not lead to results. Lots of experiments without any rationale may produce interesting and serendipitous results, but with a disproportionate effort and waste of capacities. To perform experimental studies with a rationale is without surprise the gold method with a good yield of results and appropriate expenses. The generation of this rationale assisted by the use of already available data and with modern computational techniques based on the combined expertise from natural product researchers and computational chemists harbors the key to successful drug discovery processes in the field of remedies from Mother Nature.