Having studied the technological and social forces shaping our societies, we now turn to the evolutionary forces. Among the millions of species on earth, humans are truly unique. What is the recipe for our success? What makes us special? How do we make decisions? How will we evolve? I argue that the particular combination of social abilities, diversity and our hunger for information drives societies and their cultural evolution at a speed, which is a thousand times faster than biological evolution! In fact, humans are curious by nature – we are a social, information-driven species. And that is why the explosion in the data volume and processing capacity will transform our societies more fundamentally than any other technology in the past.
People have been amazed by the power of many minds at least since the “wisdom of the crowd” was first discovered and demonstrated. The “wisdom of the crowd” means a phenomenon whereby the average of many independent judgments tends to be superior to expert judgments. A frequently cited example first reported by Sir Francis Galton (1822–1911) is the estimation of the weight of an ox.1 Galton observed villagers trying to estimate the weight of an ox at a country fair, and noted that, although no one villager guessed correctly, the average of everyone’s guesses was extremely close to the true weight. Today, there are many more examples demonstrating the “wisdom of crowds”, and this is often considered to underpin the success of democracies.2
Of course, an argument can also be made for the “madness of crowds”. In fact, at the time of Gustave Le Bon (1841–1931), the attention focused on undesirable aspects of mass psychology as exhibited by a rioting mob, for example. This was seen to be the result of a dangerous emotional contagion, and governments to this day tend to feel uneasy about crowds. However, there is a way to immunize people to some extent against emotional contagion: good education. This is important, because crowds can have many good sides, too.
Today, we have a much more nuanced understanding of crowds and swarm intelligence.3 We can explain why crowds are sometimes benign, but cause trouble in other circumstances.4 For the wisdom of crowds to work, first, independent information gathering is crucial. Second, opinion formation should be decentralized, drawing on local knowledge and specialization. Third, a larger diversity of opinions tends to increase the quality of the outcome. The same applies to the diversity of in communication patterns.5 Too much communication, however, can make the group as a whole less intelligent.
In fact, if people collect information and evaluate it independently from each other, and if these diverse streams of information are suitably aggregated afterwards, this often creates better results than even the best experts can produce. This is also more or less the way in which prediction markets work. These markets have been surprisingly successful in anticipating election outcomes or the success of new movies, for example.6 Interestingly, prediction markets were inspired by the principles that ants and bees use to find the most promising sources of food.7 Such complex self-organizing animal societies have always amazed people, especially because they are based on surprisingly simple rules of interaction.
In contrast, the “wisdom of the crowd” is undermined, if people are influenced by others while searching for information or making up their minds. This can sometimes create very bad outcomes, as misjudgments can easily spread if individuals copy each others’ opinions. For example, in Asch’s conformity experiments, people had to publicly state which one of three lines was equal in length to another line presented to them.8 Before the participant answered the question, other people gave incorrect answers (as they were instructed). As a consequence, the participant typically answered incorrectly, too, even though the recognition task was simple. Recent experiments that Jan Lorenz, Heiko Rauhut, Frank Schweitzer and I performed, show that people are even influenced by the opinions of others when they are not exposed to social pressure.9 This demonstrates that the wisdom of crowds is sensitive to manipulation attempts and social influence, and that’s why one shouldn’t try to influence how people search for information and make decisions, as nudging does.
But why are our opinions influenced by others at all? This is mainly, because imitation, which builds on the experience of others, is often a successful and efficient strategy. In the following, I will discuss, how we decide, why we behave in a social way, and how information systems may change our behavior. If we want to understand how the digital revolution might change our society, we must identify the various factors that influence our decision-making. In particular, we need to find out how growing amounts of information and the increased interconnectedness of people create new opportunities. It becomes possible, for example, to overcome “tragedies of the commons” and to support the generation of collective intelligence.
9.1 Modeling Decision-Making: The Hidden Drivers of Our Behavior
One of the best-known models of human decision-making so far is that of “homo economicus”. It is based on the assumption that humans and firms are completely egoistic, rationally striving to maximize their utility in every situation. The “utility function” supposedly quantifies the payoffs (i.e. earnings) or individual preferences of every actor. Any behavior deviating from such selfish utility maximization is believed to be irrational and to create disadvantages. The obvious conclusion—sometimes referred to as “economic Darwinism”—is that humans or companies who aren’t selfish would ultimately lose the evolutionary race with those who are. Thus, natural selection is expected to eliminate other-regarding behavior based on the principle of the “survival of the fittest”. Therefore, it seems absolutely logical to assume that everyone acts selfishly and optimizes the own payoff.
For those who are familiar with this conventional economic model of human behavior, it may come as a surprise that it does not stand up to empirical scrutiny. Appendix 9.1 shows that this model is actually quite misleading. Therefore, I am offering a new, multi-faceted explanation of human decision-making in the following. I argue that self-regarding rational choice is just one possible mode of decision-making. Human decisions are often driven by other factors in ways that make it impossible to integrate them all in one “utility function”. Trying to do this, as it is common, compares “apples with oranges”. Instead, I claim that people are driven by a variety of different incentive systems, and that they switch between them. In fact, rational decision-making is commonly believed to take place in the neocortex—our most powerful brain area. But there are other parts of the brain (such as the cerebellum), which were in control before and might still sometimes be. Thus, it is my contention that there are many other drivers governing people’s behavior.
9.2 Sex, Drugs and Rock ‘n Roll
It is clear that the first and foremost priority of our body is to ensure our survival, for example, to watch out for enough water and food. To achieve this, our body produces the feelings of hunger and thirst. If we don’t drink or eat for a long time, it will be pretty difficult to focus on mathematical calculations, strategic thinking, or maximizing payoff.
Our sexual desires are similarly strong. There is an obvious, natural drive to engage in reproduction, and many people who are deprived of sexual opportunities may find sexual fantasies to occupy their thoughts pretty much. Thus, the desire to gain sexual gratification can influence human behavior substantially. This explains why people who are sexually deprived may display some pretty irritating, “irrational” behavior.
The human desire to possess has very early roots, too. Our distant ancestors were hunter-gatherers. Accumulating food and other belongings was important in order to survive difficult times, but also to trade and gain power. This desire to possess can, in some sense, be seen as the basis of capitalism.
But besides the desire to possess things, some of us also like to experience adrenaline kicks. These rushes of adrenaline were originally there to prepare our bodies for fight or flight from predators and other dangers. Today, people watch crime series or play “first-person shooter” computer games to get a similar thrill. Like sexual satisfaction, the desire to possess things and to experience adrenaline kicks is related to concomitant emotions such as greed and fear. Financial traders know this very well.
9.3 Hunger for Information
Intellectual curiosity is a further driver of human behavior. It primarily comes into play when the needs mentioned above have been sufficiently satisfied. Curiosity drives us to explore our environment and seek to understand it. By explaining how our world works, we can manipulate it better to our advantage. A trade-off between exploration and exploitation is part of all long-term reward maximizing algorithms. Individuals who solely focus on conventional sources of reward are quickly surpassed by those who discover new, more profitable avenues. In order to encourage sufficient efforts to explore our environment, our brain rewards insights with a dopamine-based hormonal rush. The effect of these hormones is excitement. In fact, as many intellectuals and others will attest, thinking can create really great pleasure!
9.4 Lessons Learned
In summary, our body has several different incentive and reward systems. Many of them are related with intrinsic hormonal, emotional, and nervous processes (the latter include the „amygdala” area of the brain and the part of the nervous system called the “solar plexus”). If we neglect these factors, we cannot gain a good understanding of human behavior. Hence, any realistic description of human decision-making in future must incorporate knowledge from those sciences that study our body and brain. Currently established theoretical models appear to be insufficient.
For example, why do many people invest much time and energy in sport that has little reproductive or material benefit? Why do people buy fast and expensive cars that do not match their stated preferences? Why do people race or fight, ride rollercoasters or go bungee jumping? It’s the related adrenaline kicks that explain it! This is also the reason why the Roman concept of “bread and circuses” (whereby the public is best appeased by satisfying their basic needs and entertaining them) is so enduring.
The above observations have important implications. It’s too simple to assume that humans would just maximize their payoffs. We are driven by a variety of different incentive and reward systems. Humans have evolved in a way, which makes them strive to satisfy many different multi-faceted desires, which are often mutually incompatible. We engage in survival, reproduction, spreading of ideas and other goals. This gives rise to multiple different goals, which steadily compete with each other.
In addition, the importance of the different reward systems varies from one person to the next. This implies different preferences and personalities (“characters”). Some people are driven to possess as much as they can, while others prefer to explore their intellectual cosmos. Further people prefer “carnal” pursuits such as sex or sports. If nothing grants satisfaction over a long period of time, an individual might turn to drugs, get sick, or even die.
9.5 Suddenly, “Irrational Behavior” Makes Sense
In other words, if we move beyond the concept of self-regarding rational choice, the behavior of intellectuals, sportsmen, sex addicts, divas and other “special” people suddenly becomes understandable. In such cases, one reward system takes precedence over the others. For most people, however, all of these rewards are important. But this does not mean that it is possible to define one personal utility function, which stays constant over time (or changes slowly). Instead I believe that, at any point in time, one kind of reward is given priority and the others are temporarily relegated. Once this reward has been gained, another desire is given priority to, and so on. This might be compared to how competing traffic flows are managed at an intersection—temporarily prioritizing one flow after another. Once a queue of vehicles has been cleared, another one is prioritized by giving it a green light. Similarly, when one of our desires has been satisfied, we give priority to another one et cetera, until the first drive becomes strong again and demands our attention.
We can also understand what happens when people are deprived, meaning that they cannot satisfy one of their desires for a long time. In such cases, it stands to reason that they try to compensate for this lack of satisfaction with other kinds of rewards. For example, some people may find it rewarding to eat or go shopping, when they are frustrated. But options for compensation are more restricted for people in poor economic conditions. If they cannot derive pleasure from consumption and possessions, from social recognition, or from intellectual activities, then, sexual adventures, adrenaline flashes or other kicks may become more important. The results may be prostitution, violence, hooliganism, crime, or drug consumption. Therefore, a better understanding of human nature and decision-making, as I have proposed it above, may enable us to address long-standing social problems in entirely new ways, benefiting society as a whole.
9.6 Multi-Billion Dollar Industries for Each Desire
It turns out that our whole lives are structured around the various rewards which drive human behavior. In the morning, we have breakfast to satisfy our hunger and thirst. Then, we go to work to earn the money we want to spend on shopping, in order to satisfy our desire to possess. Afterwards, we may engage in sports to get adrenaline kicks. To satisfy our social desires, we may meet friends or watch a soap opera. At the end of the day, we may read a book to stimulate our intellect or have sex to satisfy this desire, too. In conclusion, I would venture that most of the time, our behavior is not well understandable as result of the strategic optimization of a static utility function that does not change over time (or very little).10 Human nature can be better explained by assuming a switching between multiple desires or goals.
Even though the basis of decision theory in its current form is pretty flawed, our economy fits human nature surprisingly well!11 We have created entire multi-billion-dollar industries around each of our drives. We have developed a food industry, supermarkets, restaurants and bars to satisfy our hunger and thirst. We have built shopping malls to satisfy our desire to possess. We have constructed stadia and sports facilities to enable adrenaline kicks, either as spectators or participants. We have a porn industry and, in some jurisdictions, prostitution to meet our sexual desires. We read books, solve puzzles, travel to cultural sites, or participate in interactive online games to stimulate our intellect and satisfy our curiosity. Our media and tourism industries also aim to satisfy these needs.
However, there seems to be a natural hierarchy of desires, explaining the order in which these industries came up. When a new industry emerges, it changes the character of our society by placing additional weight on desires that didn’t previously exist or were looming in the background. So, which desires will determine the future of our society?
Currently, the fastest growing economic sector is information and communications technology. As all our other needs have been taken care of, we are now building industries to satisfy our desires as an “information-driven species”. This trend will place additional emphasis on everything related to information. In other words, the digital society to come will be much more determined by ideas, curiosity and creativity. But that’s not all…
9.7 Being Social Is Rewarding, Too
Humans are not only driven by the reward systems mentioned above. We are also social beings, driven by social desires and that’s why social media are so successful, changing our behavior, too. In fact, most people have empathy (compassion) in that they feel for others. Empathy is reflected by emotions and expressed to others by gestures and facial expressions. It even seems that all humans on our globe share some fundamental facial expressions (anger, disgust, fear, happiness, sadness, and surprise). According to Paul Ekman (*1934), these expressions are universal, as they are independent of language and culture.12 However, our social desires go further than that. For example, we seek social recognition.
The main reason for the propensity of humans to network is that we are fundamentally social beings. In some sense, we are “networked minds”. The increasing tendency of many people to form networks using social media such as Facebook, Twitter and WhatsApp underlines and reinforces this. It has even the potential to fundamentally change the way our society and economy work. Social networking through information and communication systems can stimulate our curiosity, strengthen our social desires, and support collective intelligence.
9.8 The Evolution of “Networked Minds”
It’s interesting to ask why humans are actually social beings at all? Why do we have social desires? And how is this compatible with our hypothetical selfishness and the principle of the survival of the fittest? To study this, together with Thomas Grund and Christian Waloszek I developed a computer model which simulates the interactions between utility-maximizing individuals exposed to the merciless forces of evolution. Specifically, we simulated the “prisoner’s dilemma”, a special kind of social dilemma. Here, two actors engage in a social interaction where it would be favorable for everyone to cooperate, but non-cooperative behavior is tempting and, hence, cooperative behavior is risky.
In such scenarios, the selfish “homo economicus” would never cooperate, as non-cooperative behavior yields a higher payoff than cooperation in every single decision. This undermines cooperation, and the result is a “tragedy of the commons”: the most favorable situation—where everyone cooperates—does not occur naturally, because it is unstable, and the resulting outcome is undesirable. The reasons are similar to why free-flowing traffic breaks down when a road is too busy.
In our own computer simulations of the prisoner’s dilemma, we distinguished between the actual behavior of simulated individuals (cooperative or not) from their individual preferences.13 We assumed that the preferences result from a trait called “friendliness”, which governs how much somebody considers the consequences of the own actions on others. To be consistent with mainstream economics, the computer agents representing individuals were assumed to maximize their utility function, given the behavior of the “neighbors” they were interacting with.14 However, the utility function was specified in such a way that individuals could consider more than just their own payoff from an interaction—they could also give some weight to the payoffs of their interaction partners. This weight was proportional to the “friendliness” of the individual and was set to zero for everyone at the beginning of the simulation. Thus, initially, everyone was absolutely selfish, and the payoff that others received from an interaction was given no weight (corresponding to what we call “homo economicus”).
Additionally, we assumed that the friendliness trait could be inherited to others, either genetically or through education. In our computer simulations, the likelihood that someone would have offspring increased solely depending on their own payoff (not utility). If a person cooperated but was exploited by everyone they interacted with, they would receive no payoff. As a result, this person would also have no offspring. Finally, if people managed to have payoffs and offspring, the friendliness of an offspring tended toward that of the parent, but there was also a certain natural mutation rate. The mutation rate was specified such that it did not implicitly promote significant values of friendliness.

Illustration of the outcome of computer simulations of the evolution of the trait of “friendliness”. While the familiar “homo economicus” results for most parameter combinations, an other-regarding “homo socialis” results after many generations, if offspring (children) grow up close to their parents. Note that intergenerational migration is low when local reproduction is high and vice versa. (Reproduction from Grund et al. [14], with kind permission of the Springer Nature Publishing Group.)

Snapshots of a computer simulation illustrating the emergence of other-regarding preferences (blue and green) in two initially separated populations (red and orange). The mixture of green and blue agents also indicates a cooperation between strangers. (Reproduced from Helbing [16], with kind permission of Springer Publishers.)
9.9 Making Mistakes Is Important!
How does this preference to show regard for others evolve? First, it is important to recognize that a low, but finite level of friendliness is generated due to the random mutations of the friendliness values of offspring. Second, this slight preference to show regard for others creates conditionally cooperative behavior. That is, if just enough neighbors would cooperate, a “conditional cooperator” will be cooperative as well.
Third, unconditionally cooperative individuals with a high level of friendliness are born very rarely, and only by chance. These rare “idealists” will usually be exploited. They receive poor payoffs, and have no offspring. However, idealists may catalyze cooperative behavior if he/she is born by chance into a neighborhood with a sufficient number of conditionally (or unconditionally) cooperative individuals.17
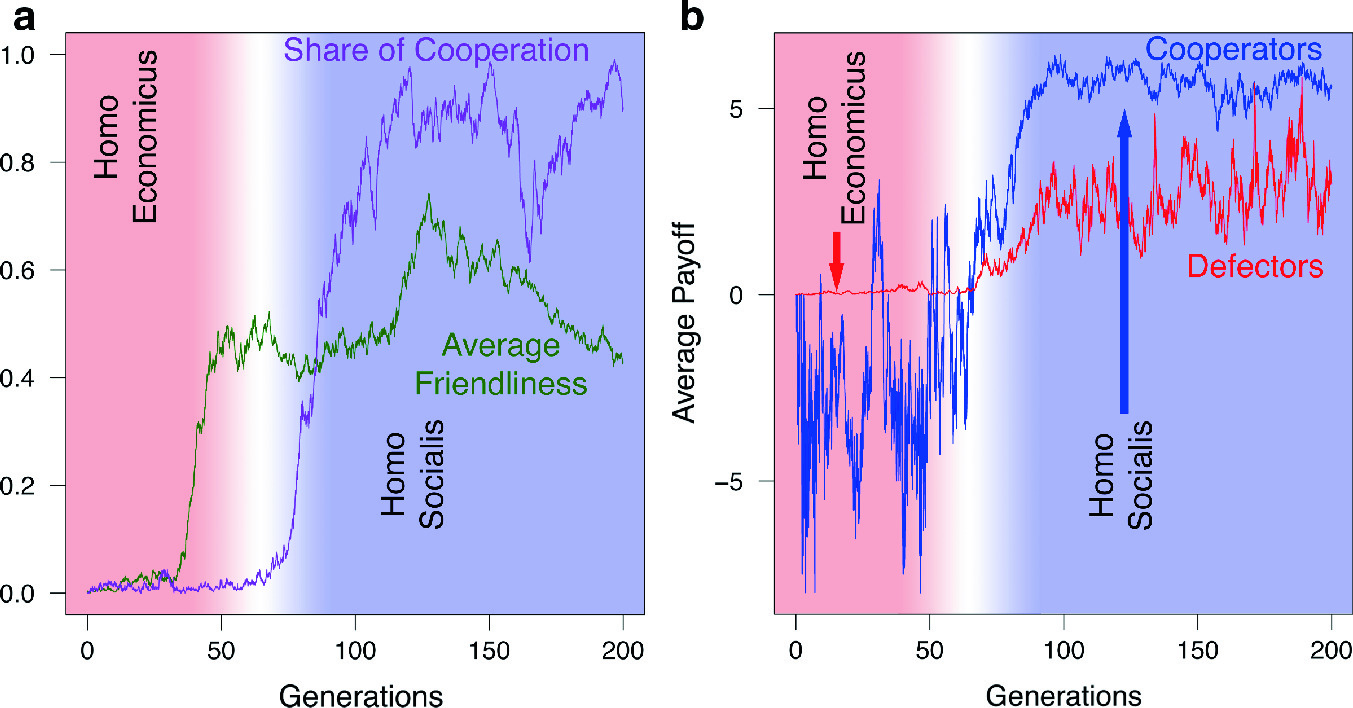
Illustration of the sudden increase in friendliness and payoff (after more than 50 generations), when “homo socialis” emerges. The increase in payoff results, as “homo socialis” is able to avoid a “tragedy of the commons”. The results of our computer simulations also show that the consideration of “externalities” (i.e. of external effects of decisions and actions) can yield a better system performance and benefits for everyone. This hints at the existence of superior organization principles for future economies, as discussed in the next chapter. (Reproduction from Grund et al. [14] with kind permission of the Springer Nature Publishing Group.)

Distribution of the level of friendliness in our simulated population. While everyone is assumed to be unfriendly in the beginning, over several generations people develop other-regarding preferences and get more friendly. (Reproduction from Grund et al. [14] with kind permission of the Springer Nature Publishing Group.)
Note that in the situation studied above, where everyone starts out as a non-cooperative “homo economicus”, no single individual can establish profitable cooperation, not even by optimizing decisions over an infinitely long period of time. Therefore, “homo socialis” can only evolve thanks to the occurrence of random “mistakes” (in this case, the birth of unconditionally cooperative “idealists” who are exploited by everyone). If such “errors” happen by coincidence in the neighborhood of other idealists or conditionally cooperative people (i.e. sufficiently friendly individuals), this can trigger a domino effect that causes an “upward spiral” towards more cooperation and eventually changes the society to the better. Then, cooperation yields higher payoffs than selfishness and “loving your neighbor as yourself” becomes rewarding.
In conclusion, the cooperativeness of “homo socialis” does not result from optimization, but from evolution. While being an idealist is a painful mistake in the beginning, later on, when other idealists or conditional cooperators join, it is the crucial success principle that makes it possible to avoid or overcome “tragedies of the commons”.
The consideration of one’s externalities on others evolves eventually, because the resulting system behavior is superior to one that ignores externalities. In fact, in an increasingly interdependent globalized world, problems caused by us tend to return to us like a boomerang. This applies, for example, to financial crises and wars, which affect us indirectly through the deterioration of public infrastructures, international migration, reduced security, etc.
9.10 “Networked Minds” Require a “New Economic Thinking”
An important lesson from the evolution of friendlier, “other-regarding” people is that such individuals consider the payoff and success of others when choosing their actions. As a consequence, their decisions become interdependent. While conventional statistical methods focusing on independent, unrelated events may sometimes suffice to characterize the decisions of “homo economicus”, we usually need complexity science to understand the interdependent decision-making of “homo socialis”. In fact, “homo socialis” may be best described by the term “networked minds”.
Note that, in congruence with the findings of social psychology, “homo socialis” has the ability to put him/herself into the shoes of others. By taking the perspective, interests, and success of others into account, “networked minds” consider the externalities of their decisions. That is, “homo socialis” decides differently from “homo economicus”. While the latter would never cooperate in a social dilemma situation, “homo socialis” is conditionally cooperative. Thus, he/she tends to cooperate if just enough neighbors do so as well. Putting it differently, “homo socialis” is able to harmonize competitive individual interests to make them more compatible with systemic efficiency. In the end, everyone can be better off. Being friendly to each other benefits everyone. The social world is not a zero sum game where one can only get more if others get less. On the contrary: in social dilemma situations or when creating collective goods, everyone can get more, if everyone behaves in an other-regarding and cooperative way.
This means that “homo socialis” is more successful than “homo economicus”, even if we measure success purely in terms of individual payoff. While the invisible hand often doesn’t work for “homo economicus” (as market failures show), it works for “homo socialis” because he/she interacts with like-minded individuals who consider the externalities of their decisions. Therefore, “homo socialis” creates systemic benefits while increasing his/her personal payoff, in contrast to “homo economicus”.
All of the above implies the need of a “New Economic Thinking” (“Economics 2.0”), which enables us to organize our economy more efficiently and effectively18 (see the next chapter and Appendix 9.2). I strongly believe that we are heading towards a new kind of economy, not just because the current economy no longer provides enough jobs in many areas of the world, but also because information systems and social media are changing our interactions and opening up entirely new opportunities. In particular, to cope with the increasing level of complexity of our world, we need to catalyze collective intelligence. In fact, the principle of “networked minds” makes it possible to bring the best ideas and skills together, and to leverage the hugely diverse range of knowledge and expertise in our societies. Let us discuss this now in more detail.
9.11 The Netflix Challenge
One of the most stunning examples of collective intelligence is the outcome of the Netflix challenge.19 The video streaming company Netflix was trying to predict what movies their users would like to see most, based on their previous movie ratings, but their predictions were frustratingly bad. So back in 2006, Netflix offered a prize of $1 million dollars to any team that could improve their own predictions of individual movie ratings by more than 10%. About 2000 teams participated in the challenge and sent in 13,000 predictions. The sample data contained more than 100 million ratings of almost 20,000 movies on a five-star scale, made by approximately 500,000 users. Netflix’ own algorithm produced an average error of about 1 star, but it took three years to improve it by more than 10%.
In the end, the prize was won by a team going by the name of “BellKor’s Pragmatic Chaos”. In the process, a number of really remarkable lessons were learned. First, given that it was very difficult and time-consuming to improve the standard method by just 10%, Big Data analytics wasn’t good at predicting people’s preferences and behavior. Second, even a minor improvement of the algorithm by just 1% created a significant difference in the top-10 movies predicted for each user. In other words, the results were very sensitive to the method used (rather than stable). Third, no single team was able to achieve a 10% improvement alone.
9.12 Diversity Wins, not the Best
The final breakthrough was made when the best-performing team decided to average over their own predictions and those made by other teams that weren’t as good. Surprisingly, collaborating with inferior teams boosted the prediction performance, while one would expect just the opposite! In other words, when complex problems must be solved, diversity wins, not the best. This seems really counter-intuitive: nobody is right, but the average of answers, none of which is good enough, gives the most accurate picture! The reason for this is subtle and extremely important for collective wisdom. Although each of the top teams almost achieved a 10% improvement over the original algorithm, each used different methods that were able to find different patterns in the data. However, no single algorithm could find them all, and “overfitting” (i.e. fitting random patterns that did not have a meaning) was probably a problem, too. By averaging the predictions, each algorithm contributed the knowledge it was specialized to find, while the errors of each algorithm were neutralized by the others.
Even more surprisingly, when more accurate predictions were given a higher weight, it didn’t improve the accuracy of the average prediction. Researchers have argued that this is because weighting more successful algorithms more highly only works if at least one algorithm is correct. In the case of the Netflix challenge, however, no single algorithm was perfect, and an equally weighted combination was better than any individual algorithm and also better than an individually weighted average that took the relative rank of each algorithm into account.245 This is probably the best argument for equal votes—in this case, however, equal votes for different approaches to problem-solving! Therefore, if we want to maximize collective intelligence, compared to our current mode of decision-making, we would have to take on board more knowledge of minorities. This would give rise to a “democracy of ideas”, which would allow us to make better use of ideas and innovations, to the benefit of our society.
9.13 Future Decision-Making Institutions
To conclude, when complex tasks are to be solved, specialization and diversity are key. So, what decision-making procedures and institutions do we need to maximize collective intelligence? Answering this question is of major importance to cope with the increasing difficulty of the challenges posed by our complex globalized world. We are in the awkward situation that no single person or computer system alone can fully grasp this complexity, and that we need to rely on inputs from others. So, for collective intelligence to work, having a knowledge base of trustable and unbiased information is essential, i.e. measures against information pollution are important.20 This requires a sufficient level of transparency and quality-ensuring mechanisms, and it requires a participatory (“crowd sourcing”) approach to get the best ideas on board (think, for example, of Wikipedia or GitHub).
Furthermore, based on what we have learned above, one should consider turning away from two modes of decision-making that are common today. First, decision-making should not be the sole province of a few “experts” taking decisions in a top-down way. Second, we must recognize that simple majority voting is also not the best decision-making mechanism. Therefore, if we want to make better decisions, we must say goodbye to the concept of the “wise king” and also to the principle of majorities deciding what should apply to everybody. This may be surprising, but in future we need better solutions to cope with the quickly increasing complexity of our world. This is particularly important in times of transition, where the majority sticks to old principles while those who understand the guiding principles of the future are still in a minority. The transformation of our current society into a digital society is a perfect example for this.
9.14 How to Produce Collective Intelligence
So, how could we create a better system to unleash the power of “collective intelligence”? First, we must abandon the idea that reality can be well described by a single model. In many cases, such as modeling traffic flow, there are several models which perform similarly well.21 This speaks for a pluralistic modeling approach. In fact, when the path of a hurricane is predicted or the impact of a car accident is simulated on a computer, it turns out that an average of several competing models often provides the best predictions.22
In other words, the complexity of today’s world cannot be grasped by a single model, mind, computer, or Artificial Intelligence system. Therefore, it’s good if several groups try to find the best possible solution independently of each other. Although all these models will give an over-simplified picture of our complex world, if we combine these different perspectives, we can often get a better approximation of the full picture. This might be compared to visiting an ornate cathedral in which every photograph only reflects some aspects of its complexity and beauty. One photographer alone, no matter how talented or how well equipped, cannot capture the full structure of the cathedral with a single photograph. A full 3D picture of the cathedral can only be gained by combining plenty of photographs representing different perspectives.
Let’s discuss another complex problem, namely finding the right insurance. Usually, it’s not realistic to read all of the small print and detailed regulations for every insurance policy on the market. Instead, we would probably ask our colleagues and friends about their experiences with their own insurers. We would then evaluate the insurances they recommend in greater detail to find the best policy that meets our needs. In this way, we engage in a collective evaluation process to solve a complex task that nobody could master alone. In the Internet age, this classical word-of-mouth system is increasingly being replaced by online reviews and recommender systems such as price comparison websites, which widens the circle of people contributing information and improves the chances that each individual will make better decisions.
While the above approach collates existing knowledge well, some methods can also create new knowledge, which is more than just the sum of its parts. Let us assume that a particular problem needs to be solved. One would then invite everyone to contribute to an online discussion of aspects that should be considered. With suitable software, it is possible to structure these aspects in an argument map, and to work out a number of different perspectives that matter.23 Once, the problem has been boiled down to anything between, say, 2 and 7 perspectives, it’s time to invite the leading representatives of these perspectives to a round table. Political or other decision makers could then moderate a deliberation process to develop an integrated solution (or a few good alternatives to choose from). When many different ideas “collide”, this often triggers innovative ideas, which may help to overcome conflicts of interest in favor of a win-win situation. This leads to better solutions, which find wider support. Therefore, rather than letting a majority impose partial interests on others, the future success principle will be to get as many good ideas on board and produce as many “winners” as possible.
The examples above illustrate how collective intelligence works. First, a number of teams needs to tackle a problem independently using diverse methodologies. Subsequently, these independent streams of knowledge need to be combined. If there is too much communication at the beginning of this process, each team may be tempted to copy promising approaches of others, which would reduce the diversity of ideas. However, if there is too little communication at the end of the process, the knowledge created by all these different approaches won’t be fully used.
9.15 What We Can Learn from IBM’s Watson Computer
It is also interesting to discuss how “cognitive computing” works in IBM’s Watson computer.24 The computer scans hundreds of thousands of sources of information, including scientific publications, and extracts potentially relevant statements. It can also formulate hypotheses and seek evidence to support or refute them. Afterwards, it produces a list of possible answers and ranks them according to their likelihood. All of this is achieved using algorithms based on the laws of probability, which calculate how likely a hypothesis is based on the available data. Compared to humans, however, Watson loses less information, which we would filter out due to limited memory and attention spans, cognitive biases, and our preference for consistency. For example, when applied in a medical context, Watson would come up with a ranked list of diseases that are compatible with a number of symptoms. While a typical doctor might assume the most common disease to cause the symptoms, Watson would also consider rare diseases, which may otherwise be overlooked.
In order to work well, it is important to feed Watson in a non-selective way with information reflecting different perspectives and potentially contradictory pieces of evidence. Then, Watson is trained by experts how to evaluate information sources, and in the end, Watson may outperform humans. The power, quantity and variety of information sources the cognitive computer can scan and the number of hypotheses it can generate and evaluate, are vastly greater than any single human could conceivably cope with. Whereas humans tend to seek and keep information that confirms their existing beliefs, Watson seems less prone to this bias, as it can consider much more pieces of information.
9.16 Collective Intelligence Versus Superintelligence
Let us finally address the question whether one could eventually create something akin to an omniscient superintelligence, if we used all the data of the Internet? In fact, the Google Brain project probably seeks to create such a superintelligence (and other projects, too). However, I expect a distributed, networked system of multiple brains interacting with Artificially Intelligences to be superior to a superbrain. Remember that the “wisdom of crowds” often exceeds that of the best experts.25 This implies that collective intelligence can outperform superintelligence. In a complex world the consideration of multiple different, independent perspectives and goals is key to success. Therefore, to cope with the challenges of the future, we need a pluralistic and participatory approach, as I will discuss it later.
It’s probably not by accident that our society is based on distributed intelligence, characterized by many moderately sized brains which think differently, with relatively small information flows among them. If this wouldn’t be useful, biological evolution might have created different solutions already.26 Smaller brains learn more quickly. Thus, collective intelligence can probably better adjust to the variability and combinatorial complexity of our world.27
9.17 There Is More to Come: New Rewards, Virtual Worlds
Diversity is often seen as an obstacle to efficient socio-economic solutions. However, it is not by chance that the most advanced species on Earth is so diverse, in contrast to social insects, for example. Diversity is and always has been a major driving force of evolution. Over millions of years, diversity has hugely increased, creating a growing number of different species. Diversity drove (and still drives) differentiation and innovation, creating new characteristics of life. Once humans became social and intelligent beings, cultural evolution set in.28 The slow process of genetic evolution was then complemented by a rapid evolution of ideas. Since then, humans have increasingly emancipated themselves from the limitations of matter and nature.
Nowadays, spreading ideas or memes (in the original sense of this word) has become more important than spreading genes. Now, besides the real world, digital virtual worlds also exist, such as massive multi-player on-line games. Thus, humans have learned to create new worlds out of nothing but information. Multi-player online games such as Second Life, World of Warcraft, Farmville, and Minecraft are just a few examples.
It is equally fascinating that new incentive systems have evolved out of these digital worlds, which are now influencing our behaviors as well. While it is perhaps unsurprising that some people care about their position in the Fortune 500 list of richest people, people are not only competitive about money and don’t just respond to material payoffs. There are many other drives as well. Tennis players and soccer teams worry about their rankings. Scientists care about the number of citations to their work, and actors live for the applause they receive.
Some people even care about the scores they reach in gaming worlds. Although such rankings usually don’t imply any immediate material or other real-world benefits, they motivate people to make an effort. It’s also pretty surprising how much time people spend to increase their number of Facebook friends or Twitter followers, or their Klout score. It is obvious, therefore, that virtual environments offer the potential to create multi-dimensional reward systems, as it is required to enable self-organizing socio-economic systems.
There can be little doubt that we are now living in a cyber-social world, in which much of our social interaction takes place online. The evolution of global information systems will drive the next phase of human social evolution. Information systems support “networked minds” and enable “collective intelligence”.29 Humans, computers, algorithms and robots increasingly weave a network that can be characterized as an “information ecosystem”. Therefore, one question becomes absolutely crucial—how will this change our socio-economic system?
9.18 Appendix 1: How Selfish Are People Really?
Our daily experience tells us that many people do unpaid jobs for the benefit of others. A lot of volunteers work for free and some organize themselves in non-profit organizations. In addition, we often leave tips on the restaurant table, even if nobody is watching and even if we’ll never return to the same place (and that’s also the case in countries where tips are not as socially obligatory as in the USA). Furthermore, billionaires, millionaires and normal people make donations to promote science, education, and medical assistance, often in other continents. Some of them do it even anonymously, meaning that they will never get anything in return, not even recognition.
Indeed, this has puzzled economists for quite some time. To fix the classical paradigm of rational choice based on selfish decision-making, they eventually assumed that everyone would have an individual utility function, which reflects their personal preferences. However, as long as there is no theory to predict personal preferences, the concept of utility maximization does not explain much. If we are to take rational choice theory seriously, we need to believe that people who help others must derive utility from it, otherwise they wouldn’t do it. But this appears to be pretty circular reasoning.30
9.18.1 Ultimatum and Dictator Games
In order to test economic theories and understand personal preferences better, scientists have performed a large number of decision experiments with people in laboratories. The findings were quite surprising and totally overturned conventional economic theory at the time.31 In 1982, Werner Güth used the “Ultimatum Game” to study stylized negotiations. In these experiments, a person (the “proposer”) was given a sum of money (say, $50) and asked to decide how much of this money he/she would offer to a second person (the “responder”). If the responder accepted the amount offered by the proposer, both got their respective share. If the responder rejected the offer, however, both received nothing.
According to the concept of the self-regarding “homo economicus”, the proposer should offer no more than $1 and the responder should accept any amount. After all, surely it is better to get a little money rather than nothing! However, it turns out that responders tend to reject small amounts, and proposers tend to offer about 40% of the money on average. A further surprise is that, all over the world, proposers tend to share with others. Similar experimental outcomes are found when playing for amounts as high as a monthly salary. To reflect these findings, Ernst Fehr (*1956) and his colleagues proposed that humans hold inherent principles of fairness and have an aversion to inequality.32 Others, such as Herbert Gintis (*1940), assumed that cooperation has a genetic basis (“strong reciprocity”).33
There is also a simpler game, known as the “Dictator Game”, which is even more stunning in some respects. In this game, one person receives some money and is asked to decide how much he/she would give to another person—it could be nothing! Although the potential recipient does not have any influence on the outcome, many people tend to share nevertheless. On average, people give away about 20% of the money they receive from the experimenter. Of course, there are always exceptions and some people don’t share.
Why do we have this tendency of people to share? In principle, this could result from the feeling of being watched by others, which might trigger behavior that complies with social norms. If this were the case, sharing wouldn’t occur when decisions are taken anonymously. To test this, we made a Web experiment with strangers who never met in person.34 Both the proposer and the responder received a fixed sum of money to participate in the experiment. However, rather than sharing money, they had to decide how to share a workload involving several hundred calculations. In the worst case, one of them would have to do all the calculations, while the other would get money without having to work! To our great surprise, even in this anonymous setting, the participants tended to share the workload quite fairly. Thus, there is no doubt that many people behave other-regarding when making decisions. They clearly have a preference for fair behavior. As observing people (“surveillance”) doesn’t largely increase the level of fairness, people apparently behave according to inborn principles or internalized norms.
9.19 Appendix 2: A Smarter Way of Interacting, not Socialism
Many governments currently try to counter inequality by redistributing wealth using centralized social welfare systems. In contrast, the concept of “homo socialis” has nothing to do with socialism or with wealth redistribution. Therefore, “homo socialis” should not be considered to be some form of tamed “homo economicus”, who shares some of his/her payoff with others. As we have discussed before, “homo economicus” tends to suffer as a result of “tragedies of the commons”, whereas “homo socialis” can overcome them by considering the externalities of decisions. Thus, “homo socialis” can generate higher profits on average. The consideration of externalities by “homo socialis” creates more favorable outcomes, at least in the long term.
In contrast, when everyone behaves like “homo economicus”, a few individuals generate high profits in social dilemma situations by exploiting others, while the vast majority of people lose out. Wealth redistribution does not overcome such “tragedies of the commons” and cannot produce average levels of profit comparable to those earned by “homo socialis”.35 In conclusion, an economy in which externalities are considered and “homo socialis” can thrive is expected to produce better and more sustainable outcomes. From a socio-economic and individual perspective, deciding like “homo socialis” is a smarter way of decision-making. It is far superior to an economy in which “homo economicus” dominates and social policies try to fix the damage afterwards (if at all).
Finally, let me address the question of whether friendly, other-regarding behavior is more likely if people have a lot of resources and can “afford” to consider the interests of others, or whether it also occurs under particularly bad conditions. In fact, in the desert and other high-risk environments, people can only survive through cooperative behavior. However, other-regarding behavior can also create benefits in low-risk environments, in which people could survive by themselves. By considering the externalities of our own behavior, our socio-economic system can continuously improve so that it produces increasingly better payoffs.36 In other words, “homo socialis” improves the performance of a socio-economic system, creating on average greater advantages for everyone. Even in a world with large cultural differences, it seems that those countries and cities achieve a particularly high quality of life, which manage to foster other-regarding behavior and consider externalities. As I said before, friendly, other-regarding behavior is to everyone’s benefit, if enough people (inter)act in this way. Nevertheless, it would be desirable to develop institutions that protect “homo socialis” from exploitation by “homo economicus”. Reputation systems and merit-based matching are such institutions, which can help to produce better outcomes in a globalized world.