1 Introduction
The choice between parametric and nonparametric density estimates is a topic frequently encountered by practitioners. The parametric (maximum likelihood, ML) approach is a natural first choice under strong evidence about the underlying density. However, estimation of normal mixture densities with unknown number of mixture components can become very complicated. Specifically, misidentification of the number of components greatly impairs the performance of the ML estimate and acts incrementally to the usual convergence issues of this technique, e.g., [11]. A robust nonparametric alternative, immune to the above problems is the classical kernel density estimate (kde).
The purpose of this work is to investigate under which circumstances one would prefer to employ the ML or the kde. A goodness-of-fit test is introduced based on the Integrated Squared Error (ISE) which measures the distance between the true curve and the proposed parametric model. Section 2 introduces the necessary notation and formulates the goodness-of-fit test. Its asymptotic distribution is discussed in Sect. 3 together with the associated criteria for acceptance or rejection of the null. An example is provided in Sect. 4. All proofs are deferred to the last Section.
2 Setup and Notation












































3 Distribution of
Under the Null

- 1.
and
as
.
- 2.
The density
and its parametric estimate
are bounded, and their first two derivatives exist and are bounded and uniformly continuous on the real line.
- 3.Let
be any of the estimated vectors
and let
denote its estimate. Then, there exists a
such that
almost surely and
whereis a vector of the first derivatives of
with respect to
and evaluated at
while


![$$\begin{aligned} c(n)&= \frac{1}{nh}\frac{1}{2\sqrt{\pi }} + \frac{h^4}{4} \left\{ \sum _{l=1}^k\sum _{r=1}^k w_lw_r \phi _{(\sigma _l^2+\sigma _r^2 )^\frac{1}{2}}^{(4)}(\mu _l- \mu _r) \right\} +o(h^4)\\ \sigma _1^2&= \int \{ f''(x)\}^2 f(x)\,dx - \left\{ \int f''(x) f(x)\,dx\right\} ^2 = \mathrm {Var}\{f''(x)\}\\ \sigma _2^2&= \frac{1}{2 \sqrt{2\pi }}\sum _{l=1}^k \sum _{r=1}^k w_lw_r \phi _{(\sigma _l^2 +\sigma _r^2)^{\frac{1}{2} }}( \mu _l - \mu _r) \\ \sigma _{30}^2&= \sigma _{30}^2(\varvec{\mu , \sigma , w}) = \left[ \int D'f_0(x,\varvec{\mu , \sigma , w})f''(x)\,dx \right] A(\varvec{\mu , \sigma , w})^{-1} \\&\phantom { = \sigma _{30}^2(\varvec{\mu , \sigma , w}) =} \times \left[ \int Df_0(x,\varvec{\mu , \sigma , w})f''(x)\,dx \right] \end{aligned}$$](../images/461444_1_En_7_Chapter/461444_1_En_7_Chapter_TeX_Equ28.png)











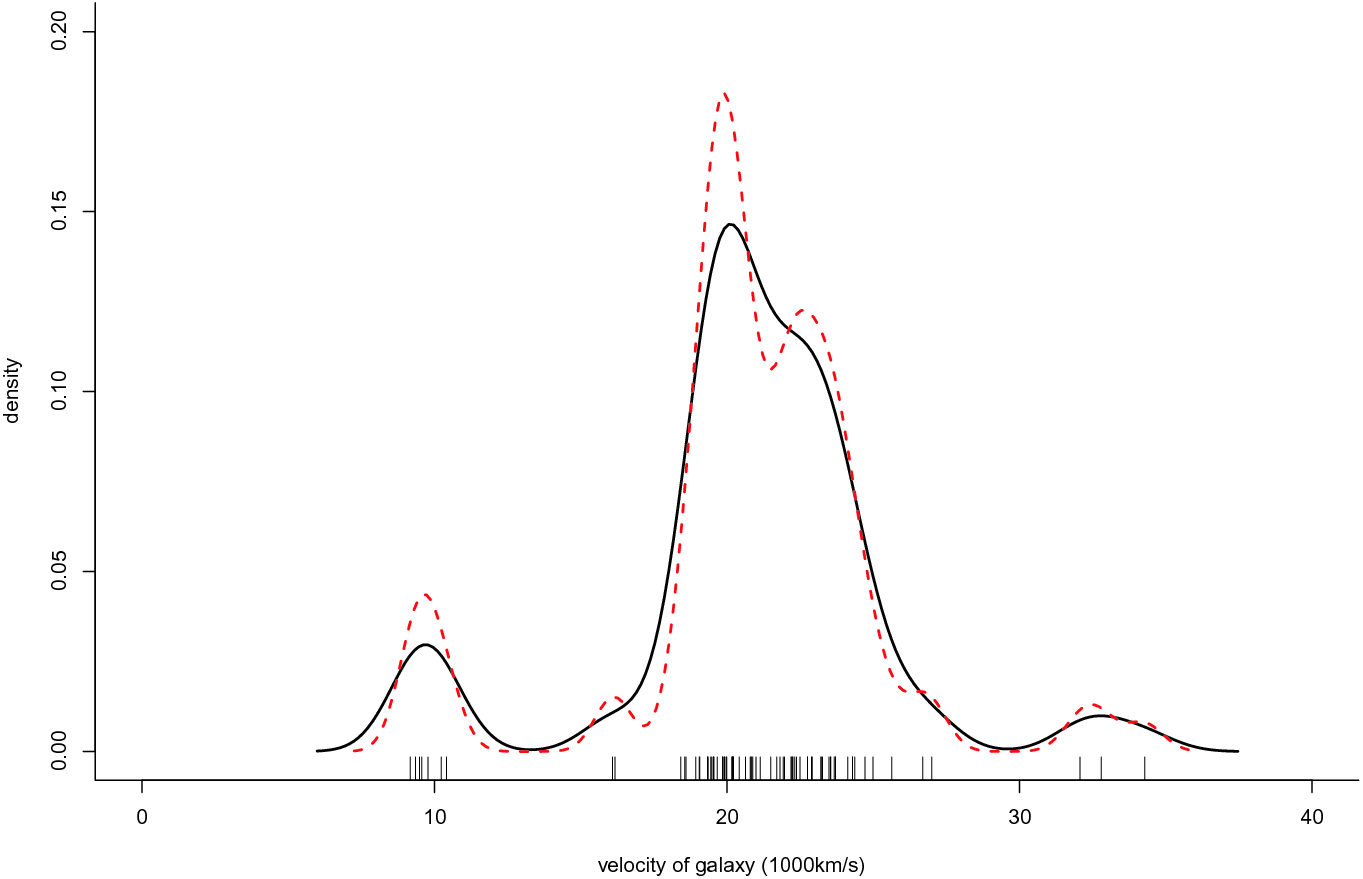
Variable bandwidth and ML estimates for the Galaxies data
4 An Example
As an illustrative example, the Galaxies data of [14] are used. The data represent velocities in km/sec of 82 galaxies from 6 well-separated conic sections of an unfilled survey of the Corona Borealis region. Multimodality in such surveys is evidence for voids and superclusters in the far universe.








5 Proof of Theorem 1


![$$\begin{aligned} I_3&= \int \left\{ \hat{f}(x; \varvec{\hat{\mu }, \hat{\sigma }, \hat{w}}) - f(x; \varvec{\mu , \sigma , w}) \right\} ^2\,\mathrm{d}x \nonumber \\&= \int \Bigg \{\sum _{i=1}^k \frac{ w_i}{2 \sigma _i^3} \Bigg [ (x- \mu _i)^2 - (x- \hat{\mu }_i)^2 \Bigg ] (1+o_p(n^{-1}))\Bigg \}^2\,\mathrm{d}x = o_p(n^{-1}), \end{aligned}$$](../images/461444_1_En_7_Chapter/461444_1_En_7_Chapter_TeX_Equ12.png)











![$$\begin{aligned} +2 \int \left[ \left\{ \hat{f}(x;h ) - \mathbb E\hat{f}(x; h) \right\} - \left\{ \hat{f}(x; \varvec{\hat{\mu }, \hat{\sigma }, \hat{w}}) - f(x; \varvec{ \mu , \sigma , w}) \right\} \right] \times \end{aligned}$$](../images/461444_1_En_7_Chapter/461444_1_En_7_Chapter_TeX_Equ20.png)














