When we are born, we are incapable of doing anything. We can’t even hold our head straight at that time, but eventually we start learning. Initially we all fumble, make tons of mistakes, fall down, and bang our head many times but slowly learn to sit, walk, run, write, and speak. As a built-in mechanism, we don’t require a lot of examples to learn about something. For example, just by seeing two to three houses along the roadside, we can easily learn to recognize a house. We can easily differentiate between a car and a bike just by seeing a few cars and bikes around. We can easily differentiate between a cat and a dog. Even though it seems very easy and intuitive to us as human beings, for machines it can be a herculean task.
Machine Learning is the mechanism through which we try to make machines learn without explicitly programming them to do so. In simple terms, we showcase the machine a lot of pictures of cats and dogs, just enough for the machine to learn the difference between the two and recognise the new picture correctly. The question here might be the following: What is the need of so many pictures to learn something as simple as the differntiating between cats and dogs? The challenge that the machines face is that they are able to learn the entire pattern or abstraction features just from a few images; they would need enough examples (different in some ways) to learn as many features as possible to be able to make the right prediction whereas as humans we have this amazing ability to draw abstraction at different levels and easily recognize objects. This example might be specific to an image recognition case, but for other applications as well, machines would need a good amount of data to learn from.
Machine Learning is one of the most talked about topics in the last few years. More and more businesses want to adopt it to maintain the competitive edge; however, very few really have the right resources and the appropriate data to implement it. In this chapter, we will cover basic types of Machine Learning and how businesses can benefit from using Machine Learning.
- 1.
Machine Learning is using statistical techniques and sometimes advanced algorithms to either make predictions or learn hidden patterns within the data and essentially replacing rule-based systems to make data-driven systems more powerful.
Let’s go through this definition in detail. Machine Learning, as the name suggests, is making a machine learn, although there are many components that come into the picture when we talk about making a machine learn.
One component is data, which is the backbone for any model. Machine Learning thrives on relevant data. The more signals in the data, the better are the predictions. Machine Learning can be applied in different domains such as financial, retail, health care, and social media. The other part is the algorithm. Based on the nature of the problem we are trying to solve, we choose the algorithm accordingly. The last part consists of the hardware and software. The availability of open sourced, distributed computing frameworks like Spark and Tensorflow have made Machine Learning more accessible to everyone. The rule-based systems came into the picture when the scenarios were limited and all the rules could be configured manually to handle the situations. Lately, this has changed, specifically the number of scenarios part. For example, the manner in which a fraud can happen has dramatically changed over the past few years, and hence creating manual rules for such conditions is practically impossible. Therefore, Machine Learning is being leveraged in such scenarios that learn from the data and adapts itself to the new data and makes a decision accordingly. This has proven to be of tremendous business value for everyone.
- 1.
Supervised Machine Learning
- 2.
Unsupervised Machine Learning
- 3.
Semi-supervised Machine Learning
- 4.
Reinforcement Learning
Each of the above categories is used for a specific purpose and the data that is used also differs from each other. At the end of the day, machine learning is learning from data (historical or real time) and making decisions (offline or real time) based on the model training.
Supervised Machine Learning
Customer Details
Customer ID | Age | Gender | Salary | Number of Loans | Job Type | Loan Default |
---|---|---|---|---|---|---|
AL23 | 32 | M | 80K | 1 | Permanent | No |
AX43 | 45 | F | 105K | 2 | Permanent | No |
BG76 | 51 | M | 75K | 3 | Contract | Yes |
In Supervised Learning, the model learns from the training data that also has a label/outcome/target column and uses this to make predictions on unseen data. In the above example, the columns such as Age, Gender, and Salary are known as attributes or features, whereas the last column (Loan Default) is known as the target or label that the model tries to predict for unseen data. One complete record with all these values is known as an observation. The model would require a sufficient amount of observations to get trained and then make predictions on similar kind of data. There needs to be at least one input feature/attribute for the model to get trained along with the output column in supervised learning. The reason that the machine is able to learn from the training data is because of the underlying assumption that some of these input features individually or in combination have an impact on the output column (Loan Default).
There are many applications that use supervised learning settings such as:
Case 1: If any particular customer would buy the product or not?
Case 2: If the visitor would click on the ad or not?
Case 3: If the person would default on the loan or not?
Case 4: What is the expected sale price of a given property?
Case 5: If the person has a malignant tumor or not?
- 1.
Linear Regression
- 2.
Logistic Regression
- 3.
Support Vector Machines
- 4.
Naïve Bayesian Classifier
- 5.
Decision Trees
- 6.
Ensembling Methods
Another property of Supervised Learning is that the model’s performance can be evaluated. Based on the type of model (Classification/Regression/time series), the evaluation metric can be applied and performance results can be measured. This happens mainly by splitting the training data into two sets (Train Set and Validation Set) and training the model on a train set and testing its performance on a validation set since we already know the right label/outcome for the validation set. We can then make the changes in the Hyperparameters (covered in later chapters) or introduce new features using feature engineering to improve the performance of the model.
Unsupervised Machine Learning
Customer Details
Customer ID | Song Genre |
---|---|
AS12 | Romantic |
BX54 | Hip Hop |
BX54 | Rock |
AS12 | Rock |
CH87 | Hip Hop |
CH87 | Classical |
AS12 | Rock |
In the above data, we have customers and the kinds of music they prefer without any target or output column, simply the customers and their music preference data.
Customer Details
Customer ID | Romantic | Hip Hop | Rock | Classical |
---|---|---|---|---|
AS12 | 1 | 0 | 2 | 0 |
BX54 | 0 | 1 | 1 | 0 |
CH87 | 0 | 1 | 0 | 1 |

Clusters post Unsupervised Learning
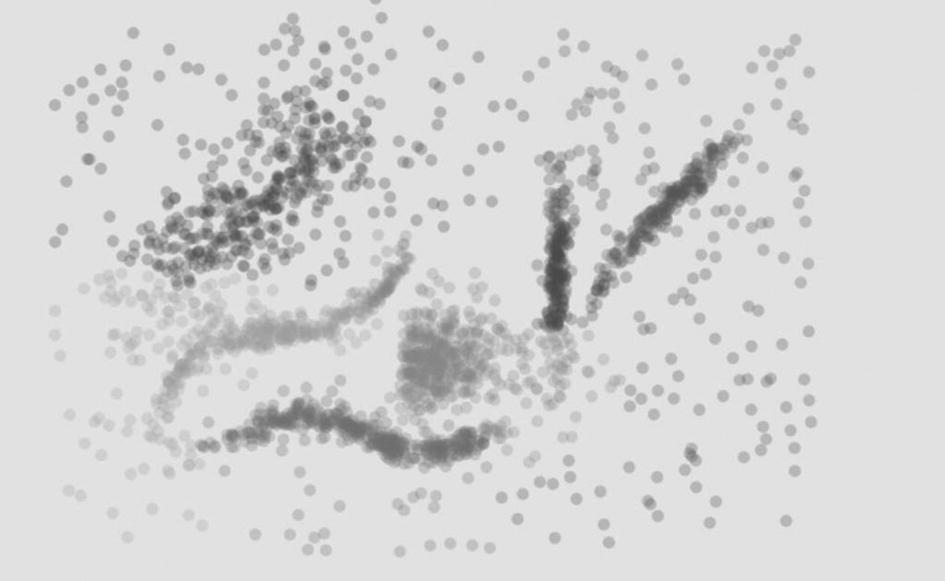
Overlapping Clusters
There are many applications that use unsupervised learning settings such as
Case 1: What are different groups within the total customer base?
Case 2: Is this transaction an anomaly or normal?
- 1.
Clustering Algorithms (K-Means, Hierarchical)
- 2.
Dimensionality Reduction Techniques
- 3.
Topic Modeling
- 4.
Association Rule Mining
- 1.
There is no labeled training data and no predictions.
- 2.
The performance of models in unsupervised learning cannot be evaluated as there are no labels or correct answers.
Semi-supervised Learning
As the name suggests, semi-supervised learning lies somewhere in between both supervised and unsupervised learning. In fact, it uses both of the techniques. This type of learning is mainly relevant in scenarios when we are dealing with a mixed sort of dataset, which contains both labeled and unlabeled data. Sometimes it’s just unlabeled data completely, but we label some part of it manually. The semi-supervised learning can be used on this small portion of labeled data to train the model and then use it for labeling the other remaining part of data, which can then be used for other purposes. This is also known as Pseudo-labeling as it labels the unlabeled data. To quote a simple example, we have a lot of images of different brands from social media and most of it is unlabeled. Now using semi-supervised learning, we can label some of these images manually and then train our model on the labeled images. We then use the model predictions to label the remaining images to transform the unlabeled data to labeled data completely.
The next step in semi-supervised learning is to retrain the model on the entire labeled dataset. The advantage that it offers is that the model gets trained on a bigger dataset, which was not the case earlier, and is now more robust and better at predictions. The other advantage is that semi-supervised learning saves a lot of effort and time that could go to manually label the data. The flipside of doing all this is that it’s difficult to get high performance of the pseudo-labeling as it uses a small part of the labeled data to make the predictions. However, it is still a better option rather than manually labeling the data, which can be very expensive and time consuming at the same time.
Reinforcement Learning
The is the fourth and last kind of learning and is a little different in terms of the data usage and its predictions. Reinforcement Learning is a big research area in itself, and this entire book can be written just on it. We will not go too deep into this as this book focuses more on building machine learning models using PySpark. The main difference between the other kinds of Learning and Reinforcement Learning is that we need data, mainly historical data to training the models whereas Reinforcement Learning works on a reward system. It is primarily decision making based on certain actions that the agent takes to change its state trying in order to maximize the rewards. Let’s break this down to individual elements using a visualization.
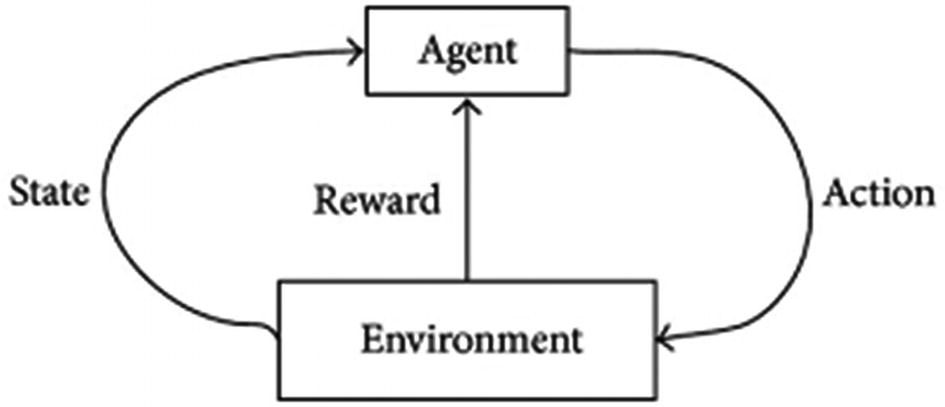
Autonomous Agent: This is the main character in this whole learning process who is responsible for taking action. If it is a game, the agent makes the moves to finish or reach the end goal.
Actions: These are sets of possible steps that the agent can take in order to move forward in the task. Each action will have some effect on the state of the agent and can result in either a reward or penalty. For example, in a game of Tennis, actions might be to serve, return, move left or right, etc.
Reward: This is the key to making progress in reinforcement learning. Rewards enable the agents to take actions based on if it’s positive rewards or penalties. It is a feedback mechanism that differentiates it from traditional supervised and unsupervised learning techniques
Environment: This is the territory in which the agent gets to play in. Environment decides whether the actions that the agent takes results in rewards or penalties.
State: The position the agent is in at any given point of time defines the state of the agent. To move forward or reach the end goal, the agent has to keep changing states in a positive direction to maximize the rewards.
The unique thing about Reinforcement Learning is that there is a feedback mechanism that drives the next behavior of the agent based on maximizing the total discounted reward. Some of the prominent applications that use Reinforcement Learning are self-driving cars, optimization of energy consumption, and the gaming domain. However, it can be also used to build recommender systems as well.
Conclusion
In this chapter we briefly looked at different types of Machine Learning approaches and some of the applications. In upcoming chapters, we will look at Supervised and Unsupervised Learning in detail using PySpark.