Artificial intelligence (AI), we are led to believe, will soon be weaponized in a new information war. Such speculation happened in the aftermath of Cambridge Analytica and its shadowy use of data in politics (cf. Chessen, 2017). As one popular opinion piece by Berit Anderson (2017) warns, the infamous global consulting firm “is a piece of a much bigger and darker puzzle—a Weaponized AI Propaganda Machine being used to manipulate our opinions and behavior to advance specific political agendas.” Better models of voter behavior will “prey on your emotions” by varying messages to your current state of mind. Future campaigns may have “250 million algorithmic versions of their political message all updating in real-time, personalized to precisely fit the worldview and attack the insecurities of their targets.” It is not hard to imagine a coming dystopia where AI undermines democratic free will and fair elections.
How could AI be so easily imagined as a political weapon? How could developments in machine classification be consider relevant to politics? Especially when Cambridge Analytica and other applications are what Dave Karpf (2016) calls “bullshit,” offering further evidence of what Jesse Baldwin-Phillipi (2017) calls the myth of data-driven campaigning. Bullshit and myths overstate the power of these tools, allow them to be positioned as weapons, and convince parties and politicians to buy them—much like Cambridge Analytica did in convincing the world its tools worked and influenced elections.
These fears overlook the state-of-the-art too. Artificial intelligence is already part of political campaigns and has been for a while. In 2018, Blue State Digital (BSD), a leading digital political strategy firm, announced a partnership with AI startup Tanjo (Blue State Digital, 2018). BSD introduced the collaboration in a blog post, explaining that “AI is all around us, creating breakthrough innovations for companies and consumers alike.” Alike is an apt way to describe the benefits of the technology. Tanjo uses AI, or what it calls Tanjo’s Automated Persona (TAP), to model human behavior. Where message testing usually requires real people, Tanjo creates audiences on demand. TAPs, Blue State Digital continues, simulate “humans’ distinct personalities and unique interests” to “predict how they’ll react to content.” Whether TAPs work as promised is not my question here, nor is whether these fakes will undermine democratic trust. What I seek to explain is how we came to believe that humans—especially their political behavior—could be modelled by computers in the first place.
My chapter synthesizes how innovations in political science beginning in the 1950s led to the conditions of possibility for the use of artificial intelligence in politics today. Building on this book’s focus on the history of AI from rule-based to today’s temporal flows of classifications, my chapter offers a genealogy of artificial intelligence as a political epistemology. Through media genealogy, my project takes a broad view of media, following communication theorists Alexander Monea and Jeremy Packer, who define media as “tools of governance that shape knowledge and produce and sustain power relations while simultaneously forming their attendant subjects” (Monea & Packer, 2016, p. 3152). Media genealogy examines “how media allowed certain problems to come to light, be investigated, and chosen for elimination and how media aided in the various solutions that have been enacted” (p. 3155). Computers, as this chapter explores, came to be a solution to the problems of information war, the utility of the field, and the uncertainties of public opinion.
Over fifty years ago, a new wave of social scientists and programmers, part of what I call the New Political Science (White, 1961), began to experiment with computer simulation as a proxy for public opinion and government behavior. These experiments did not stay in academia. American federal agencies, the US military, and the Democratic Party, briefly, funded these experiments. As a result, digital simulation, forecasting, and modelling became part of new approaches to political systems reformatted as cybernetic systems, or what another important figure in the New Political Science, Karl Deutsch (1963), called in his book the “Nerves of Government.” Building on Orit Halpern’s (2014) expansive history of the implications of cybernetics to politics, one could say these nerves of government prefigured the neural nets now sold to Blue State Digital.
In trying to navigate the scope of this change, I focus on what Donna Haraway (1985) calls breakdowns, in this case, between computing and politics. These breakdowns happened at MIT: the Simulmatics Corporation and its academic successor Project Cambridge.1 Drawing on archival research, I analyze the constitutive discourses that formulated the problems to be solved and the artifacts of code that actualized these projects. Simulmatics Corporation and Project Cambridge—the other Cambridge analytics—integrated behavioralism with mathematical modelling in hopes of rendering populations more knowable and manageable.
My chapter begins by following developments in political science that laid the ground for Simulmatics and Project Cambridge to be heralded as major advances in the field. Harold Lasswell, in particular, encouraged the application of computing in the social sciences as a new weapon to fight what we would now call an information war (a continuation of Lasswell’s work in psychological warfare). If these desires were inputs for computers, then Simulmatics and Project Cambridge were the outputs. These projects reformatted politics to run on computers, and, in doing so, blurred voter opinion and behavior with data and modelling. These breakdowns enabled computers to simulate voters and facilitated today’s practices of what political communication scholar Phillip N. Howard (2006) calls thin citizenship—an approach to politics where data and the insights of AI stand in for political opinion. In doing so, these other analytics at Cambridge erased the boundaries between mathematical states and political judgments—an erasure necessary for AI to be seen as a political epistemology today.
Moody Behavioralists and the New Political Science
By the early 1960s, a mood had set in political science (Dahl, 1961). This mood resulted from deliberate work by researchers who desired a more scientific and objective field, complementary to the physical sciences. Some proponent voices included Harold Lasswell, Ithiel de Sola Pool, and Karl Deutsch, who were themselves influenced by the work of Oskar Morgenstern, John von Neumann, and Herbert Simon in economics and computer science (Amadae, 2003; Barber, 2006; Cohen-Cole, 2008; Gunnell, 1993, 2004; Halpern, 2014; Hauptmann, 2012). Advocates sought to aid government, particularly the US government, in what was a new turn in the long history of the social sciences as a state or royal science (Igo, 2007, p. 6). The distinguished political scientist Robert Dahl would call this a “behavioral mood.” Critics would call it the New Political Science.
Dahl described behavioralism2 as a mood because it was hard to define. He wrote, “one can say with considerable confidence what it is not, but it is difficult to say what it is” (1961, p. 763). The closest he came to providing a definition was, in a 1961 review of behavioralism, a quotation from a lecture at the University of Chicago in 1951 by prominent political scientist David Truman. Truman defined political behavior as “a point of view which aims at stating all phenomena of government in terms of the observed and observable behavior of men” (p. 767). Truman’s definition minimized resistance to future applications of computing. If behavior could be stated as observations, these observations could be restated as data. Looking back, behavioralism contributed to the breakdown between human and computer intelligence that Katherine Hayles describes as virtuality: “the cultural perception that material objects are interpenetrated by information patterns” (1999, pp. 13–14). The emphasis on observation may seem rather mundane now. That was Dahl’s point. As he concluded, “the behavioral mood will not disappear then because it has failed. It will disappear because it has succeeded,” i.e., fully incorporated into the field (Dahl, 1961, p. 770).
- 1.
The influence of the University of Chicago. Dahl mentions Harold Lasswell (who supervised Pool there) and Herbert Simon;
- 2.
The influx of European scholars after World War II, such as Paul Lazarsfeld (who Dahl cites as one of the first to develop the scientific approach to politics continued by behavioralism). Karl Deutsch, I suggest, is also part of this trend though Dahl does not mention him;
- 3.
Wartime applications of the social sciences (cf. Simpson, 2003);
- 4.
Funding priorities of the Social Science Research Council that focused on more scientific methods (Ross, 1991);
- 5.
Better survey methods that allowed for a more granular application of statistics that aggregated data; and,
- 6.
The influence of American philanthropic foundations (cf. Hauptmann, 2012).
These developments fit with the development of scientism, a faith in scientific knowledge’s power, that ran through the twentieth-century American social sciences.
Historian Dorothy Ross attributes the turn toward scientism to the leadership of Charles Merriam. The University of Chicago professor helped design the social sciences after World War I. Initially engaged in progressive politics, Merriam turned away from politics and the progressive movement led him to a view of the social sciences that mirrored the natural sciences. According to Ross (1991), Merriam’s late program of research “proposed a science defined by ‘method,’ orientated to ‘control’ and sustained by organized professional structures to promote research” (p. 396). Merriam’s program made its way into the New Political Science through his disciple Harold Lasswell, who became “the heir of Merriam’s technological imagination” (p. 455); “Lasswell became the father of the 1950s behavioral movement, in political science, an extension in elevation of scientism in the profession, and by that lineage Merriam was rightly the grandfather” (p. 457). The New Political Science manifested Lasswell’s vision for the field and created the possibilities for computing as a political epistemology.
Harold Lasswell and the A-bomb of the Social Sciences
Harold Lasswell was a defining figure in political science, communication studies, and policy studies. Lasswell not only led American academia, he also collapsed divisions between the state and the research community. During World War II, he helped formulate techniques of psychological warfare at the War Communications Division of the Library of Congress (along with his student Ithiel de Sola Pool) (Simpson, 2003). After the War, Lasswell was a leader in American social science and became president of the American Political Science Association (APSA) in 1956.
Lasswell outlined his vision for the field in his inaugural speech. Building on Merriam’s attempts at integrating the human and natural sciences, Lasswell began by declaring, “my intention is to consider political science as a discipline and as a profession in relation to the impact of the physical and biological and of engineering upon the life of man” (1956, p. 961). Behavioralism would be his way to solidify the scientific foundations of the field at a time when the American government needed insights into growing complexity and risk. He continues, “It is trite to acknowledge that for years we have lived in the afterglow of a mushroom cloud and in the midst of an arms race of unprecedented gravity” (p. 961). Political science, particularly in its behavioral mood, would be instrumental in this arms race.
War was a key frame to legitimate Lasswell’s vision for political science. As Nobel prize–winning American experimental physicist Luis Alvarez suggested to the Pentagon in 1961, “World War III... might well have to be considered the social scientists’ war” (quoted in Rohde, 2013, p. 10). The goal was not necessarily waging better war but rather waging what Lloyd Etheredge (2016) called a “humane approach” to politics (cf. Wolfe, 2018). Better weapons might lead to more humane wars. Speaking to journalist and close friend of the Simulmatics Corporation Thomas Morgan in 1961, Lasswell later suggested, “if we want an open society in the future, we are going to have to plan for it. If we do, I think we have a fighting chance” [italics added] (quoted in Morgan, 1961, p. 57).
What would be the weapon in this fight? What would be the weapon of choice for behavioralists and Lasswell’s followers? If the atom bomb was the weapon of World War II, then the computer might be the weapon of World War III. As Jennifer Light (2008) notes in her history of computing in politics, “first developed as calculating machines and quickly applied to forecasting an atomic blast, computers soon took on a new identity as modelling machines, helping communities of professionals to analyze complex structures and scenarios to anticipate future events” (p. 348). At the time of Lasswell’s speech, computers had gone mainstream. In 1952, CBS contracted Remington Rand and its UNIVAC computer to forecast election results. After UNIVAC predicted an overwhelming Eisenhower win, CBS decided not to air the prediction. It turned out to be correct. By the next election, all major broadcasters used computers for their election night coverage (Chinoy, 2010; Lepore, 2018, p. 559). In tandem, the US Census and the Social Science Research Council had begun to explore how computers could better make available data for analysis (Kraus, 2013).
Social sciences would create their own weapon too: the Simulmatics Corporation. It was an “A-bomb of the social sciences” according to Harold Lasswell in a magazine article that doubled as promotion for the new company. Simulmatics, he continued, was a breakthrough comparable to “what happened at Stagg Field” (quoted in Morgan, 1961, p. 53). Lasswell was referring to Simulmatics’ corporation offerings—its People Machine—that built on the company's early research for the 1960 Kennedy campaign, where computer simulations were used to advise a political party on how voters might respond to issues. Lasswell sat on the company’s first Advisory Council along with Paul Lazarsfeld, Morris Janowitz, and John Tukey. The project was a culmination of the coming together of scientism, behavioralism, and the New Political Science.
Simulmatics Corporation: The Social Sciences’ Stagg Field
The Simulmatics Corporation began as a political consultancy in 1959.3 The company sold itself as a gateway for companys and federal agencies to the behavioralism sciences. Where Jill Lepore (2020) tells the story of the company's founding in response to the failures of Democratic Presidential Candidate Adlai Stevenson, I emphasize its links to the New Political Science and its first project, research for the 1960 camapaign to elect John F. Kennedy as President.
are like the unsubstantiated sea serpent that appears periodically along the Atlantic coast. They are discussed every year by popular political analysts and commentators, but without much factual demonstration. (p. 12)
Voting trends were some of the facts Bean analyzed to measure these tides in his 1948 book How to Predict an Election, published with the encouragement of Paul Lazarsfeld.
Where Bean used demographic statistics to plot voter trends, Simulmatics could use survey data due to the maturation of the field. The production of public opinion had advanced considerably since the straw polls at the turn of the twentieth century. Surveys about the average American—usually assumed to be a white male—had gained institutional legitimacy, necessary for informed market and government research (Igo, 2007). Ongoing survey research provided a new way to measure the political tides, and Simulmatics made use of these newly public data collections. In 1947, pollster Elmo Roper donated his data to Williams College, creating one of the first repositories of social science data. By 1957, the collection had become the Roper Public Opinion Research Center. Pool and others arranged to have access to the Center’s surveys, under the agreement that their opponents in the Republican Party had the same access (which they did not use). Survey data became the basis for computer simulation soon after. William McPhee, coauthor of Voting: A Study of Opinion Formation in a Presidential Campaign with Bernard R. Berelson and Paul F. Lazarsfeld, 3 started work on a voter simulator at the Bureau of Applied Social Research at Columbia University by 1960. McPhee worked with Simulmatics to develop this model in its early political consultancy.
Behavioralism eased the slippage from survey to simulation. Citing Bernard Berelson, Paul F. Lazarsfeld, and William McPhee (1954), Simulmatics proposed the existence of “a human tendency to maintain a subjective consistency in orientation to the world” (Pool, Abelson, & Popkin, 1964, p. 9). Consistency, Pool and his colleagues explained, can be rather difficult to maintain in politics. Voters face many decisions that might cause inconsistencies. A desire to maintain consistency might cause a voter to switch party support, usually late in the campaign. In other words, the elusive swing voter could be found and modelled by looking for voters with contradictory opinions and forecasting whether the cross-pressure might cause a change in behavior.
Structure of the Simulmatics 1960 Model of the American Electorate reproduced from the original
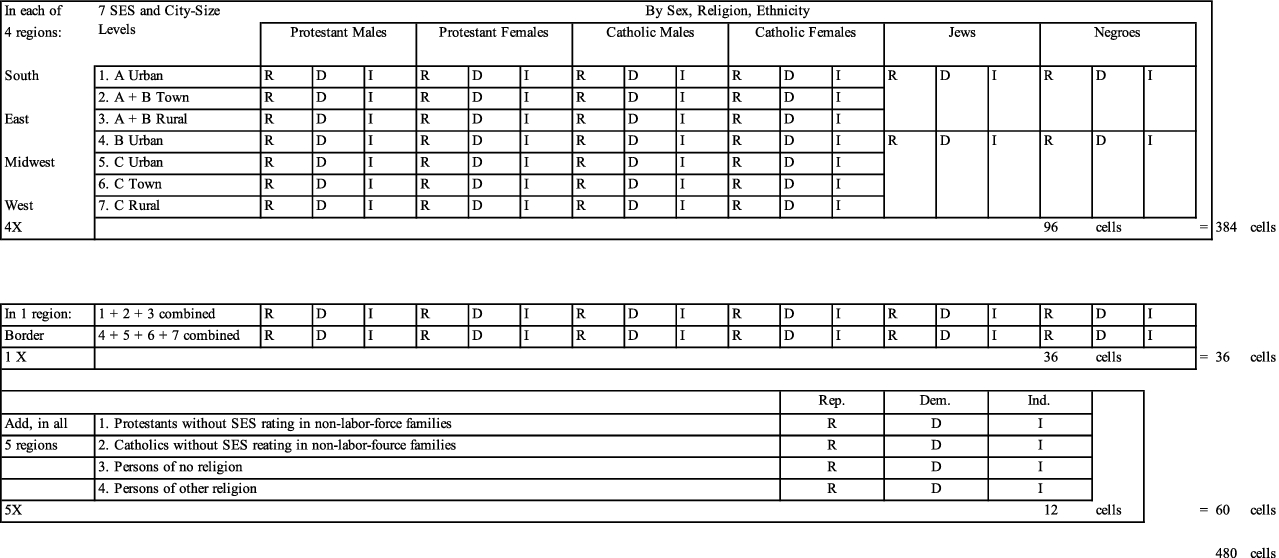
The matrix arranged the opinions of 480 voter types on 52 issues. The 480 types were “defined by socio-economic characteristics.” Pool and Abelson explained, “a single voter type might be ‘Eastern, metropolitan, lower-income, white, Catholic, female Democrats.’ Another might be, ‘Border state, rural, upper-income, white, Protestant, male Independents.’” In effect, each voter type was a composite of region, race, gender, and political disposition. Notably, voter types did not include education, age, or specific location. Voter types, instead, fit into one of four abstract regions. Each voter type had “opinions” on what Simulmatics called issue clusters. For each intersection of voter type and issue cluster, the databank recorded their occurrence in the population and their attitude (Pool et al., 1964, pp. 30–31).
The matrix masked the arduous interpretive work that created it. Voter types only approximated the survey results, since as Pool et al. (1964) write, “it will be noted that we did not use all possible combinations of all variables, which would have given us some 3,600 voter types, many of which would have been infinitely small” (p. 27). Issue cluster was “private jargon” that referred to their interpretation of multiple survey responses about opinions on political matters; “most of these were political issues, such as foreign aid, attitudes toward the United Nations, and McCarthyism” (p. 27).
What’s notable in this moment is the slippage between voter type and voter. Simulmatics’ methods directly acknowledge the influence of the seminal works in American political behavior, Voting from 1954 by Bernard Berelson, Paul F. Lazarsfeld, and Simulmatic’s own William N. McPhee and The American Voter from 1960 by Angus Campbell, Philip Converse, Warren Miller, and Donald E. Stokes. Both studies undermined the idea of individual voter behavior, suggesting instead that voting patterns could be predicted by group and partisan affiliations. These studies established a view to this day that individual choice matters less than group identity and partisanship (Achen & Bartels, 2017, pp. 222–224). By creating more dynamic ways to interact with the aggregate, Simulmatics demonstrated that the voter could be modelled without needing to be understood. Pressures between group identities, not individual opinions, animated the simulations. The behavior of voters not only became the behavior of voter types, but these models were dynamic and replaced political decision-making with probable outcomes.
Simulmatics delivered its first report to the Democratic National Committee just before its 1960 Convention (Lepore, 2018, pp. 598–599). Their first report focused on African Americans, marking a notable change in the object of public opinion; prior survey research largely ignored African Americans. Historian Susan Igo (2007) notes that the dominant pollsters George Gallup and Elmo Roper “underrepresented women, African Americans, laborers, and the poor in their samples” (p. 139). Indeed, the first Middletown survey of the 1920s excluded responses from African Americans (p. 57). The first Simulmatics report marks an important turn where race became a significant variable that divided calculations of the American public. The report found that non-voting was less of a concern than expected, and that African Americans might vote more frequently than might have been expected at the time. For Pool and others, the report proved that their data could provide insights into smaller subsets of the American population. While the first report did not involve computer simulation, it marked an early use of new computer technology to address emerging urban and racial issues in the US (McIlwain, 2020, pp. 214–217).
Reproduced from Pool et al. (1964, p. 46)
Cross pressure patterns | |||
---|---|---|---|
Republicans | Democrats | Independents | |
Protestants | (1) | X (2) | (2) |
Catholics | X (4) | (3) | (3) |
Other | (4) | (5) | (5) |
Though much of the simulation was manual, Simulmatics’ work here marks the beginning of when interests and values were used to model group behavior. These models in turn helped campaigns forecast reactions to future positions and platforms. Behavior, in other words, could be calculated and stand-in for stated opinion. This was a moment where the past behavior of individuals became autonomous, capable of behaving in ways never encountered by the fleshy double.
These innovations had a modest effect on the campaign, if any. Simulmatics did not change campaign strategy, and there is no evidence that subsequent campaigns of the 1960s turned again to simulations (Lepore, 2015). Pool never appears in iconic accounts of the 1960 election. Pool and Abelson (1961), reflecting on the campaign, noted that “while campaign strategy, except on a few points, conformed rather closely to the advice in the more than one hundred pages of the three reports, we know full well that this was by no means because of the reports. Others besides ourselves had similar ideas. Yet, if the reports strengthened right decisions on a few critical items, we would consider the investment justified” (pp. 173–174). Though Simulmatics tried to advise other campaigns, they never found another high-profile candidate like the Kennedy Campaign. The company had plenty of work elsewhere.
The Simulmatics Corporation moved into many directions—the Vietnam war effort, marketing, building simulation games—that drew on its mathematical models of behavior. The company continued to collect more survey data and advise its clients about how to study behavior more scientifically. James S. Coleman, a sociologist prominent for introducing mathematical modelling to the field, assisted Simulmatics in developing games as well as its Dynamark analysis for advertising and marketing on Madison Avenue. In Vietnam, Simulmatics earned approximately $24 million USD in ARPA contracts for data science, such as gauging public opinion about the US occupation of Vietnamese villages (Rohde, 2011). Foreign populations were not just calculated in Vietnam. In its ten-year run, Simulmatics modelled the behaviors of Americans, Venezuelans, and the Vietnamese. Results were mixed. ARPA and military records described a pained relationship with Simulmatics involving Vietnam with projects not meeting contractual or scientific expectations (Weinberger, 2017, pp. 180–181). By 1970, the corporation went bankrupt, nearly acquired by Mathematica, the company cofounded by Oskar Morgenstern (Lepore, 2020, p. 384n65). By then, the political ground had shifted with the Democrats out of the White House following Nixon’s win in 1968. Kissinger’s realpolitik replaced McNamara’s whiz kids. The prospects of a humane war in Vietnam became a farce.
Simulmatics’ Long Afterglow
Simulmatics generated some public debate about the consequences of political simulation. Its possible misuses fueled the plot of the novel The 480 by surfer-political scientist-novelist Eugene Burdick. Named after Simulmatics’ 480 voter types, Burdick’s bestselling novel described a presidential campaign that used computer modelling to manipulate the public—a dystopian scenario not far off from the present-day worries mentioned in the introduction (Anderson, 2018, pp. 89–92). Historian Jill Lepore (2018) nicely captures Burdick’s criticism that “if voters didn’t profess ideologies, if they had no idea of the meaning of the words ‘liberal’ or ‘conservative,’ they could nevertheless be sorting into ideological piles, based on their identities” (p. 599). Simulmatics thought otherwise. Writing in the introduction to a 1964 book about the project, Pool and his collaborators Robert Abelson and Samuel Popkin (1964) noted that the project “has been subject to a number of sensational newspaper and magazine articles and even of a work of fiction” citing Burdick and promising their book would “correct these lurid fantasies” (p. 1).
The project had its critics in academia, including Noam Chomsky and earlier Howard B. White, former dean of the New School. White coined the phrase the New Political Science. Writing in the summer 1961 issue of Social Research, he argued that these tools enabled better manipulation of voters, and he later cited Simulmatics as a key example of the New Political Science. White did not think voter manipulation was new, merely improved. As he wrote, “there is nothing new in manipulated opinion and engineered consent.... Even the sheer bulk of distortion is not altogether news, merely more refined” (1961, p. 150). “What is new,” White continued, “is the acceptability, the mere taken-for-grantedness of these things.” What he called a New Political Science5 accepted these innovations as mere tools and not profound revisions to the discipline’s normative project. He worried this “value-free” political science would legitimate social manipulation. Pool rejected these claims in a rejoinder published the following year in the same journal (Pool, 1962), and White defended his argument in a response (White, 1962).
Simulmatics was celebrated in the behavioral sciences. The Simulmatics Project became a story repeated in the annals of simulation and modelling in the social sciences (Guetzkow, 1962; IBM Scientific Computing Symposium on Simulation Models and Gaming & International Business Machines Corporation, 1964). A 1965 special issue of the American Behavioral Scientist, only eight years old at the time, celebrated the work of Simulmatics as the “benchmark in the theory and instrumentation of the social sciences” (de Grazia, 1965, p. 2). The editorial was written by Alfred de Grazia, an early editor of the journal. A staunch believer in the firm, he subsequently led Simulmatics’ ill-fated operation in Saigon for a time (Weinberger, 2017, pp. 174–182). Articles included Simulmatics studies of business systems, communications, and international relations as well as a postscript on the 1964 election. Harold Lasswell wrote the issue’s introduction entitled “The Shape of the Future.” In no uncertain terms, he explained that the power, and danger, of Simulmatics methods was when “new advances in technique make it possible to give the leaders of rival governments, political parties and pressure groups improved advice for managing their campaigns to influence public judgment of candidates, issues and organization” (Lasswell, 1965, p. 3). Perhaps in response to critics of this power like White and Burdick, Lasswell maintains that “officers of the Simulmatics Corporation are acting responsibly” (1965, p. 3). What is missing from this glimpse of the future is any sign that Simulmatics would be bankrupt four years later, and punch cards would be burned in opposition to this political technology.
In spite of its commercial failure, Simulmatics exemplified a move to use computers for forecasting and model testing in the social sciences (cf. Rhode, 2017). Certainly, the company had many contemporaries. Jay Forrester, also at MIT, developed the DYNAMO (DYNAmic Models) programming language at the MIT Computation Center that eventually resulted in the 1972 report “Limits of Growth” (Baker, 2019). MIT’s Compatible Time-Sharing System (CTSS) ran simulations scripted in GPSS and OP-3, another simulation language developed at MIT (Greenberger, Jones, Morris, & Ness, 1965). These are a few American examples of the early field of computer simulations and simulation languages that developed into its own subfield.
Given this afterglow, Simulmatics and its voter types should be seen as one predecessor to today’s Tanjo Animated Personas. The similarities are striking. Blue State Digital explains that they are “based on data. This data can include solely what is publicly available, such as economic purchase data, electoral rolls, and market segmentations, or it can also include an organization’s own anonymized customer data, housed within their own technical environment.” If data informs models, then Simulmatics signals an important moment when computation enabled a reevaluation of prior research. In its case, Simulmatics found value in the survey archives of the Roper Center. Today, TAPs are refined by a “machine learning system, along with a human analyst, [that] generates a list of topics, areas of interest, specific interests, and preferences that are relevant to the persona” to generate affiliations akin to issue clusters. Each TAP has a score attached to each interest, similar to the scores used by Simulmatics attached to voter types.
Political simulations, either in 1961 or 2019, promise something akin to thin citizenship. Phillip N. Howard (2006) describes thin citizenship as when data functions as a proxy for the voter. Blue State Digital explains that personas “are based on the hypothesis that humans’ distinct personalities and unique interests can predict how they’ll react to content. We can simulate these interests and conduct testing with them in a way that can augment, or sometimes be as valid as, results from focus groups and surveys.” These selling points could just as easily be lifted from a Simulmatics brochure’s discussion for its marketing products like Dynamark, a computer program “used to determine the relative value of different promotional activities and their effects upon the attitudes of different subgroups of the population” (Simulmatics, 1961).
TAPs, however, promise to be a much more integral part of a campaign than Simulmatics was. Even though Pool et al. (1964) suggested that the 1960 experiment had merit due to providing “on demand research concerning key issues” (p. 23), the experiment took place at the margins. Further, Simulmatics relied on survey research only for its political simulation. I now turn to the case of Project Cambridge, which provides a way to understand the scale of computers as a political epistemology.
Project Cambridge
Project Cambridge was part of Pool’s ongoing work on political simulation. As Simulmatics suffered financially, Pool began to collaborate with a professor returning to MIT, J. C. R. Licklider. Licklider is one of the key figures and funders of modern computing. By 1967, he had returned to MIT after working at the Advanced Research Projects Agency (ARPA) in 1964 followed by a stint at IBM. His research at the time nicely complemented Pool’s own interests in data and polling. Licklider was developing the idea of computer resources that he would come to call “libraries of the future,” while advocating for better connectivity to the databanks housed by information utilities—a vision not so far off from the design of the seminal ARPANET (Licklider, 1969). Where Simulmatics showed the utility of survey research for computer simulation, Licklider recognized the promise of digital data in general for computing. Pool and Licklider, then, began to collaborate on a project to better collect and operationalize data in government and politics, which became known as Project Cambridge.
- 1.
International data sets on public opinion, national statistics and demographics, communications and propaganda efforts, as well as armament spending; and
- 2.
Domestic data sets on US economic statistics and demographic statistics, including public opinion polls from the Roper Center.
Project Cambridge, then, was an early attempt to construct a unified system to manage databases. A project like Simulmatics would be just one of many databases in the system, ideally used in connection with each other.
noted the similarity between this agent and the work of AI researcher Oliver Selfridge. While this agent was by no means the autonomous intelligence that motivated the most hyped AI research, it grew directly out of the tradition of automating simple decision-making, and of giving machines the ability to direct themselves. (p. 178; cf. McKelvey, 2018)
Project Cambridge, by 1974, included Pool’s General Implicator, a textual analysis tool, as well as SURVEIR, a new data management tool for the Roper Center data. These projects proved to be more a result of statistical computing than artificial intelligence, but the desire certainly endured to use computers as a way to better model humans and societies.
The project also brought together many researchers associated with the New Political Science. Licklider served as principal investigator, but the project was led by Douwe Yntema, senior research associate in psychology at Harvard and senior lecturer at the MIT Sloan School of Management. These two, along with Pool, were only a small part of a massive academic project that listed more than 150 participants, including Karl Deutsch, in its first annual report.
Such ambition was another trend of the 1960s. Beginning in 1963, the US Census Bureau and the Social Sciences Research Council considered ways to convert federal statistics into databases for researchers. When made aware of the project, the press reacted poorly, raising privacy and surveillance concerns. The Pittsburgh Post-Gazette, for example, ran the headline “Computer as Big Brother” in 1966. That the project ultimately failed in part due to this public reaction was a warning unheeded by Project Cambridge (Kraus, 2013).
Project Cambridge was controversial from the beginning. As Mamo (2011) writes, the project had two problems: “defense patronage, which ran afoul of Vietnam-era student politics, and the fear that collections of data and analytical tools to parse this data would erode individual privacy” (p. 173). Computing was seen then, and perhaps again now, as a powerful tool of the state (Turner, 2006). Harvard withdrew direct participation in the project due to public outrage (Mamo, 2011, pp. 165–175).
While Licklider and Pool publicly made efforts to distance the project from the government, conceptually Project Cambridge resembled the kind of “on-demand” research described by Pool. It also resembled the work of Karl Deutsch, where digital agents could become the nervous system of a new cybernetic vision of government. Deutsch served as a member throughout the project and was also a member of the Advisory Committee on Policy at the time of the project’s renewal in 1971. He defended the project against criticism from the student press about its relationship with the government and the Vietnam War. Writing to the Harvard Independent in the fall of 1969, Deutsch argued, “At the present time, I think mankind is more threatened by ignorance or errors of the American or Soviet or Chinese government than it would be by an increase in the social science knowledge of any of these governments” (quoted in Mamo, 2011, p. 40). The computer was, in other words, the more humane approach to political realities.
Deutsch’s comments reflect his interest in improving the science of the social sciences. His 1963 book The Nerves of Government explicitly discusses the importance of models for the future of political science, moving from classical models to innovations in gaming and eventually to cybernetics. In fact, the book popularized cybernetics to the field. It ends with a flow chart labelled “A Crude Model: A Functional Diagram of Information Flow in Foreign Policy Decisions.” This diagram, according to Orit Halpern (2014), was inspired by computer programming; she explains that in it there “are no consolidated entities, only inputs, outputs and ‘screens’ that act to obstruct, or divert, incoming data” (p. 189). Where Halpern situates the chart in the larger reformulation of data through cybernetics and governance via neural networks, I wish to emphasize this diagram, the book, and Project Cambridge as a shared application of cybernetic thinking to politics itself. This integration of computing as both a model and a screen for politics was integral to Project Cambridge. Indeed, a second draft of the “Project Cambridge Proposal,” before Harvard withdrew its institutional support (see Lepore, pp. 287–288), repeatedly mentions Deutsch and his approach to data as inspiration and a resource to be mobilized. In other words, Deutsch and Project Cambridge conceptually integrated simulation into the political system, as part of a collective intelligence or neural network designed to optimize governance.
Such close integration with government never amounted to much. The project was always in a bind: “It needed to claim a defence mission to satisfy the Mansfield Amendment and receive funding, but it also needed to deny that very same purpose in order to attract researchers” (Mamo, 2011, p. 172). It also faced intractable technical problems, such as developing on the experimental MULTICS operating system and, more practically, coordinating its hodgepodge of data, models, and researchers into one unified research environment. Tackling this latter problem became the project’s lasting contribution. Project Cambridge partially initiated the formulation of the computational social sciences. Simultaneously, Stanford University developed its own program for computational social sciences, the Statistical Package for the Social Sciences (SPSS), still in use today (Uprichard, Burrows, & Byrne, 2008). Project Cambridge contrasted itself with SPSS, seeking to be a more radical innovation that became known as the Consistent System (CS) (Klensin & Yntema, 1981; Yntema et al., 1972). CS was “a collection of programs for interactive use in the behavioral sciences” that included “a powerful data base management system... with a variety of statistical and other tools” (Dawson, Klensin, & Yntema, 1980, p. 170). Though not as popular as SPSS or FORTRAN, Project Cambridge’s legacy enabled the crystallization of computational modelling in the social sciences.
While Project Cambridge had a direct influence on the tools of data science, it also furthered the breakdowns begun by the Simulmatics Corporation. Simulmatics established that public opinion as data could be manipulated in a simulation that could have utility as an on-demand and responsive tool for parties and other decision makers. In one sense, this indicates a prehistory to the observed reliance on data to make decisions in campaigns, or what’s called computational management (Kreiss, 2012). Project Cambridge further entrenched the New Political Science to funders, peers, and the public as the vanguard of the field. Even as its ambitions outstripped its technical output, Project Cambridge created a consistency beyond its Consistent System. The project pushed the behavioral sciences beyond survey research into integrated databases that in turn could train computational agents to stand-in for political and voter behavior.
Conclusion
Simulmatics and Project Cambridge, as well as the history that led to their development, provide a prehistory to artificial intelligence in politics. Through these projects, the utility of AI to politics became imaginable. In doing so, political science joins the ranks of what others call the cyborg sciences (Halpern, 2014; Haraway, 1985; Mirowski, 2002). This transformation was marked by looking at politics through computers, or what Katherine Hayles (1999) calls reflexivity: “the movement whereby that which has been used to generate a system is made, through a changed perspective, to become part of the system it generates” (p. 8). As Donna Haraway (2016), quoting Marilyn Strathern, reminds me “it matters what ideas we used to think other ideas (with)” (p. 12).
The other Cambridge analytics turn re-modelled what John Durham Peters calls the politics of counting. “Democracy,” Peters (2001) writes, “establishes justice and legitimacy through a social force, the majority, which exists only by way of math” (p. 434). Numbers legitimate democratic institutions as well as represent the public majority back to the public: “Numbers are... uniquely universal and uniquely vacuous: They do not care what they are counting. Their impersonality can be both godlike and demonic. Numbers can model a serene indifference to the world of human things” (p. 435). Simulmatics and Project Cambridge helped change the numerical epistemology by integrating computers, data, and behavioral theory. Democracy was still about the numbers. Ones and zeros, rather than polls and tallies, now functioned as political representation.
Reformatting politics led to technical not democratic change. The blowback felt by Project Cambridge and Simulmatics demonstrates public concern about technology out of control, not in the public service. Those feelings were widespread in popular culture and only mitigated by the rise of personal computing (Rankin, 2018; Turner, 2006). Pool’s ambitions make the limitations of this cybernetic turn all the more apparent—a warning applicable to artificial intelligence. These problems are well stated in E. B. White’s critical article about the New Political Science. To Lasswell’s characterization of the Simulmatics as the A-Bomb of the social sciences, White (1961) asked “why political scientists want so much to believe that kind of statement that they dare make it” (p. 150). He ends with perhaps the most damning of questions for the claimed innovation: “If Lasswell and Pool really believe that they have an A-bomb, are they willing to leave its powers to advertisers?” Pool did just that, selling Simulmatics to advertising and marketing firms as well as to the US military in Vietnam where the company reported on villagers’ opinions of the American occupation.
We might ask the same questions for AI in politics? Indebted to a fifty-year history, benefitting from tremendous concentrations of capital—be it financial, symbolic, or informational—and trusted to envision a new society through its innovations, the bulk of AI seems to be applied to better marketing and consumer experiences. The revolutionary potential of TAPs ultimately seems only to be better message testing in advertising. Where is the AI for good, or a model for a better politics?
The task for a critical AI studies might be to reimagine its political function. To this end, my history offers tragedy as much as critique. Scientists tried and failed to use computers to create a more humane politics; instead, their innovations were enlisted in new campaigns of American imperialism. Yet, the alternative is to wish for simpler times as seen in White’s own normative framework. White had strong opinions about the nature of democracy. In rallying against a value-free political science, he signals a vision of politics as a rarefied space of important decisions, similar to philosopher Hannah Arendt’s. As he writes, “of course a political decision takes a risk—but it can be considered irrational only if the voters do not care to consider the object of voting as related to the common good.” Political decisions matter, but they won’t matter “if we are educated to restrict reason to the kind of thing you use to decide whether to spend the extra fifteen cents for imported beer” (White, 1961, p. 134). White instead wanted to return politics to an even more abstract and intangible space than the banks of a computer. Today, White’s vision for democracy seems just as elusive and invites a certain curiosity Pool’s cold pragmatism and others building computers for a humane politics without defending them. Can a critical studies of AI find a radical politics for these machines aware of what past searches for new weapons in the past have done?
- 1
I learned that the Simulmatics Corporation was the subject of Dr. Jill Lepore's new book If Then: How the Simulmatics Corporation Invented the Future after submitting this chapter. Dr. Lepore’s tremendous historical work offers a detailed, lively account of the company's history including its start discussed here. Interested readers should refer to the Dr. Lepore’s book for a deeper history of the company. Thanks to Dr. Lepore for her kind reply and helpful comments. Any similarities are coincidental. Any errors are my own.
- 2
Behavioralism here should not be confused with the similarly named political behaviorism (Karpf, Kreiss, Nielsen, & Powers, 2015, p. 1892n5).
- 3
Samuel Popkin continued his work in the field of political polling, helping to popular low-information rationality in voter behavior.
- 4
The number is either 65 or 66. In their book, Pool, Abelson, and Popkin state they used 65 surveys, but they state 66 surveys in their 1961 article in Public Opinion Quarterly.
- 5
Not to be confused with the Caucus for a New Political Science organized at the annual meeting of the American Political Science Association in 1967. This new political science explicitly opposed the rise of behavioralism and state-sponsored research (Dryzek, 2006).