Introduction
The non-ferrous pyrometallurgy industry is providing increasing volumes of primary metal and is recycling an ever-widening variety of metals. These developments bring with them a number of challenges including issues associated with source materials scarcity, the increasing compositional and structural complexity of modern devices, environmental impact and sustainability .
The rapid increases in computer power, the ability to collect and analyse large volumes of data, the ability to measure change and control equipment in industrial plant, has created opportunities to further improve the performance of existing operations. The implementation of practices that take advantage of accurate process models would enable improved process stability, process and feed optimization , improved campaign planning, and ultimately the widespread implementation of feed-forward process control systems in pyrometallurgical processes. These actions have the potential to increase process throughput, metal recoveries and to enable the efficient treatment of complex but profitable feed sources. The research program outlined in this paper, on the development of the robust thermodynamic databases and process models, is driven by the need to respond to the above challenges and with the aim of taking advantage of these new opportunities.
The availability of accurate thermodynamics databases is essential for the development of accurate predictive models and their use in the optimisation of pyrometallurgical processes. These databases are the foundations of the process models—and determine the quality of the predictive outcomes. A variety of fundamental data are required to develop these databases including, but not limited to, information on solidus, liquidus , phase equilibria , solid and liquid solubilities, distribution coefficients, thermodynamic activities, vapor pressures, enthalpy functions in multi-component, multi-phase systems.
Overview of common phases and composition ranges observed in some pyrometallurgical Cu, Pb and Zn smelting and recycling processes
Cu | Pb | Zn | S | Fe | SiO2 | Al2O3 | CaO | MgO | As | Sn | Sb | Bi | Ag | Au | ||
---|---|---|---|---|---|---|---|---|---|---|---|---|---|---|---|---|
wt% | wt% | wt% | wt% | wt% | wt% | wt% | wt% | wt% | wt% | wt% | wt% | wt% | ppm | ppm | ||
Cu smelting | Gas/dust | 15–25 | 0.1–2 | 0.1–2 | 5–15 | 15–25 | 5–10 | 1–3 | 0.1–2 | 0.1–2 | 1–3 | 0.05–0.15 | 0.2–1.1 | |||
Slag | 0.5–1 | 0.5–2 | 30–45 | 30–45 | 2–5 | 1–3 | 0.3–3 | |||||||||
Matte | 50–70 | 0.1–5 | 0–2 | 20–26 | 10–25 | 0–1 | 0 | 0 | 0 | 0–0.5 | 0–0.5 | 0–0.1 | 0–3000 | 0–30 | ||
Cu direct-to-blister Cu converting | Slag | 15–25 | 0.1–5 | 0.05–2 | 0.1–1 | 30–40 | 15–30 | 2–5 | 1–3 | 0.3–3 | ||||||
Fayalite Slag | 2–5 | 0–1 | 0–0.5 | 0.2–1.5 | 35–50 | 30–40 | 0–0.5 | 0–0.5 | 0–0.5 | |||||||
Copper blow slag | 20–45 | 0–1 | 0–0.5 | 0–0.3 | 30–40 | 10–20 | 0–0.1 | 0–0.1 | 0–0.1 | |||||||
Ca-ferrite slag | 15–25 | 0.1–1.0 | 0–0.1 | 0–0.3 | 35–45 | 0.1–1.5 | 0–0.5 | 15–20 | 0–0.1 | |||||||
Matte | 50–80 | 0.1–5 | 0–2 | 19–25 | 0.5–10 | 0–0.1 | 0 | 0 | 0 | 0–0.5 | 0–0.5 | 0–0.1 | 0–3000 | 0–30 | ||
Blister | 98.0–99.7 | 0–0.01 | 0 | 0.01–1 | 0–0.01 | 0 | 0 | 0 | 0 | 0–0.03 | 0–5000 | 0–40 | ||||
Pb Sintering | Pb sinter | 0.3–1.5 | 35–60 | 4–10 | 1–43 | 8–14 | 5–13 | 0.5–2.5 | 2–11 | 0.3–2 | 0.1–0.5 | 0.1–1 | 0.01–0.1 | |||
Pb smelting | Pb smelting slag | 0.3–1.5 | 35–55 | 3–7 | 0–1 | 5–15 | 20–40 | 0.5–2 | 2–8 | 0.1–1 | ||||||
Pb Blast Furnace | Pb reduction slag | 0.1–0.7 | 1–3 | 10–20 | 1–3 | 20–30 | 20–25 | 2–5 | 10–20 | 0.5–2 | 0–0.1 | |||||
Pb bullion | 0–4 | 94–98 | 0–0.1 | 0–1 | 0–0.01 | 0–1 | ||||||||||
Matte | 10–40 | 10–30 | 3–13 | 5–15 | 3–25 | 0.5–2 | ||||||||||
Zn fuming | Slag | 0.01–0.3 | 0.01–2 | 0–20 | 0.4–0.7 | 20–25 | 25–30 | 5–8 | 13–20 | 3–7 | ||||||
Cu and Pb refining | Speiss | 20–30 | 30–50 | 0–5 | 3–10 | 0–3 | 5–12 | 0–3 | 1–3 | 0–0.4 | 0–1000 | 0–30 | ||||
Common solid solutions | Spinels | 0–0.1 | 0–0.5 | 0–25 | 0 | 55–70 | 0–10 | 0–0.1 | 0–5 | |||||||
Melilite | 0 | 0–5 | 1–20 | 0 | 20–30 | 20–25 | 1–5 | 20–25 | 2–5 |
Liquid Slag : | |
---|---|
(Al+3, Ca+2, Mg+2, Si+4,Cu+1, Fe+2, Fe+3, Pb2+, Zn2+, Sn2+, Sb+3, As+3, Bi+3, Ag+1, Au +1,)(O−2, S−2), Modified Quasichemical Formalism (MQF) in Quadruplet Approximation Spinel: [Cu+2, Fe+2, Fe+3, Al+3, Mg+2, Zn+2]tetr[Cu+2, Fe+2, Fe+3, Al+3, Ca+2, Mg+2, Zn+2, Vacancy0]2octO4, Compound Energy Formalism (CEF) Monoxide: (FeO, FeO1.5, CuO, AlO1.5, CaO , MgO), Bragg-Williams model (B-W) Olivine: [Fe2+, Ca2+, Mg2+, Zn2+]M2[Fe2+, Ca2+, Mg2+, Zn2+]M1SiO4, CEF Dicalcium silicates: (Ca2SiO4, Fe2SiO4, Mg2SiO4, Pb2SiO4, Zn2SiO4), B-W Wollastonite: (CaSiO3, FeSiO3, MgSiO3, ZnSiO3), B-W Melilite: [Ca2+, Pb2+]2[Fe2+, Fe3+, Al3+, Zn2+][Fe3+, Al3+, Si4+] 2O7, CEF Willemite: [Zn2+, Fe2+, Mg2+][Zn2+, Fe2+, Mg2+]SiO4, CEF Zincite: (FeO, ZnO, MgO), B-W Corundum: (FeO1.5, AlO1.5), B-W Mullite: [Al+3, Fe+3]2[Al+3, Si+4, Fe+3][O−2, Vacancy]5, CEF Calcium ferro-aluminates Ca(Al, Fe)2O4, Ca(Al, Fe)O7, Ca(Al, Fe)12O19 Pyroxenes: [Fe2+, Ca2+, Mg2+]M2[Fe2+, Fe3+, Mg2+, Al3+]M1[Fe3+, Al3+, Si4+]BSiAO6, CEF Liquid metal/matte/speiss: (CuI, CuII, FeII, FeIII, PbII,AsIII, ZnII, SnII, SbIII, BiIII, AgI, AuI, OII,SII), MQF in Pair Approximation Digenite-bornite: (Cu2S, FeS, PbS, ZnS, Vacancy2S) ‘Cu3As’, (Cu, As), MQF in Pair Approximation fcc and bcc solid alloys: (Co , Ni, Mn, Cu, Fe, Pb, O, S, Zn, As, Sb, Ag, Au ), B-W Ideal gas:> 100 species, including N2, CO , CO 2, S2, SO2, H2O, AsO, AsS, As4O6, PbO, PbS Stoichiometric compounds: > 150, including SiO2, FeS2, CaSO4, CuFeO2, Ca3Al2O6, S, ZnS, Sb2O3 |
The databases that are constructed contain fundamental descriptions of the chemical behavior of the systems and are independent of the technology that is used. This means that the databases can be used at the heart of predictive models of pyrometallurgical processes with additional parameters that take into account factors related to furnace design, construction and operation. The database can be used to predict the outcomes of copper , lead and zinc sulfide smelting processes, including copper smelting ; copper converting ; copper refining in anode furnaces; lead sintering and smelting ; lead reduction ; zinc and lead fuming; and copper and lead refining to extract precious metals . The databases can be used to describe reactions in suspended and bath smelting , blast furnaces; batch and continuous processes.
Outline of the Overall Program
In order to develop the databases for the complete range of conditions in copper , lead and zinc pyrometallurgy , an integrated research program of thermodynamic modelling and experimental measurements of phase equilibria is being undertaken.
- 1.
Slag /metal phase equilibria in the PbO-ZnO-CaO -FeO-Fe2O3-SiO2-CuxO-Al2O3-MgO slag —Pb-Cu-Fe-Zn metal alloy.
- 2.
Matte formation conditions within the gas/PbO-ZnO-CaO -FeO-Fe2O3-SiO2-CuxO-Al2O3-MgO slag and the Pb-Zn-Fe-O-S-Cu matte/alloy systems.
- 3.
Pb refining systems equilibria within the Pb-Cu-S-As-Sb-Sn-Fe matte/metal/speiss system.
- 4.
Elemental Distributions between slag , matte and metal of minor elements , including Ag, Au , As, Bi, Sn, Sb and Zn.
- 5.
Improvement of the thermodynamic database of oxide systems: Development of the thermodynamic database of the PbO-ZnO-CaO -FeO-Fe2O3-SiO2-CuxO-Al2O3-MgO slag in Pb-Cu-Fe-Zn metal alloy systems.
- 6.
Improvement of thermodynamic database of sulphur-containing systems: Development of the matte/metal/speiss thermodynamic database (focus on low temperatures) and the incorporation of minor elements .
These projects cover a wide range of chemical compositions and conditions (temperature , oxygen and sulphur partial pressures) relevant to the whole range of key lead smelting , refining and recycling systems.
For copper consortium companies the scope of the work includes complete experimental revision of the thermochemistry of the base system “Cu2O”-FeO-Fe2O3-SiO2-S with the Al2O3, CaO and MgO slagging components and As-Pb-Zn-Sn-Sb-Bi-Ag-Au other minor elements . The experiments involve determining equilibria between gas/slag /matte/blister/solid (tridymite, spinel) phases as functions of temperature , P(O2), P(SO2)/P(S2), slag Fe/SiO2 (and equilibria with tridymite or spinel). This development of thermodynamic database working with the FactSage software [5] for the above system provides direct support of the copper smelting industry sponsors.
Open experiments with P(O2) and P(SO2) in the gas/slag /matte and gas/slag /metal systems controlled by the CO /CO 2/SO2 gas mixtures, and
Closed experiments undertaken in sealed ampoules for the slag /matte/metal system.
- 1.
Base system with slagging components—initial description at Matte Grades between 50 and 80% Cu
Gas/Slag /Matte,
Gas/Slag /Metal [Cu-Fe-O-S-Si] x [Temperature ] x [P(O2)/P(SO2)] x [Fe/SiO2—Tridymite/Spinel] x [Al, Ca, Mg].
Slag /Matte/Metal.
- 2.
Distribution of Minor Elements As, Zn, Pb, Sn, Sb, Bi, Ag, Au
Gas/Slag /Matte,
Gas/Slag /Metal [Cu-Fe-O-S-Si] x [Temperature ] x [P(O2)/P(SO2)] x [Fe/SiO2—Tridymite/Spinel] x [Al, Ca, Mg]
Slag /Matte/Metal.
The techniques developed during this program for the first time enable the systematic accurate measurements of this kind to be undertaken, and these measurements provide an important foundation for the development of the thermodynamic database as well as for the overall quantitative description of the thermochemistry of copper smelting
The total number of experiments needed to completely and quantitatively characterise the whole chemical system as functions of key operational parameters is very large. The experimental needs are therefore carefully and critically reviewed. An overall summary of all required experiments is prepared and continuously revised to enable systematic analysis and selection of optimum research plan to support the development of the thermodynamic database , to close the gaps where no data is available and to resolve discrepancies.
An example of the systematic approach taken to experimental study and modelling for copper database development
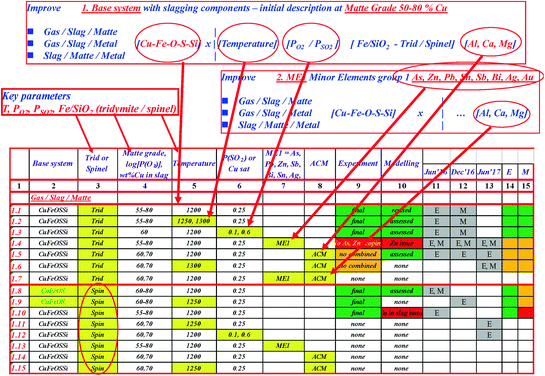
Columns 2 through 8 indicate the matrix of key parameters selected for characterisation
Columns 9 and 10 indicate current status of system description
line 1.1 represents gas/slag /matte equilibria on a tridymite substrate for a range of matte grades from 55 to 80 wt%Cu at base case conditions of 1200°C and P(SO2) = 0.25 atm.
line 1.2 presents the effect of temperature in the above system
line 1.3—the effect of P(SO2) in the above system, as so on for all key parameters.
In this way, the whole list of the experiments needed to quantitatively characterise the system as functions of the key parameters is being carefully analysed, and most critical experiments are selected and performed to support the development of the thermodynamic database using FactSage package [5].

Binary, ternary and higher order sub-systems in the system Cu2O-PbO-ZnO-Al2O3-CaO -MgO-FeO-Fe2O3-SiO2-S-(As-Bi-Sb-Sn-Ag-Au )
Demonstrating Capabilities
Complete Description of the Copper-Matte-Slag -Gas System Cu2O-Al2O3-CaO-MgO-FeO-Fe2O3-SiO2-S
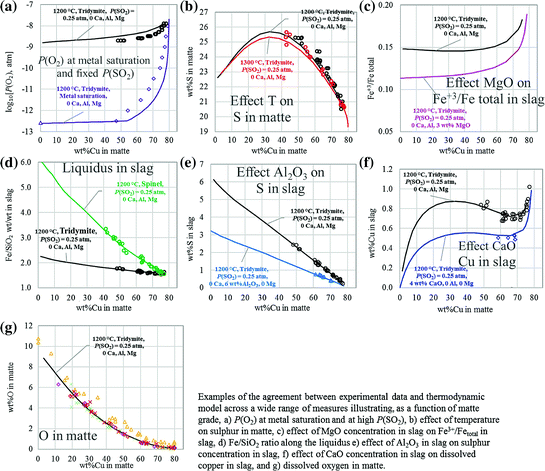
Resolving Complexities in Database Development

To effectively manage the complexity of the 16 component system Cu2O-PbO-ZnO-Al2O3-CaO -MgO-FeO-Fe2O3-SiO2-S-(As-Bi-Sb-Sn-Ag-Au ) the database development has been organised by splitting the system into two parallel, yet integrated tasks. One involves the experimental investigation and database development of the Cu-Fe-O-Pb-Zn-Ca–Si + (Ca–Mg) systems, that is the base slag /metal systems [18]. The second task is the optimisation of the slag /matte/speiss equilibria and minor element distributions between these phases.
Applications to Industrial Systems
Fluxing Diagrams
Having prepared the updated and optimised thermodynamic databases they can be used in conjunction with the FactSage computer platform to predict specific process outcomes.
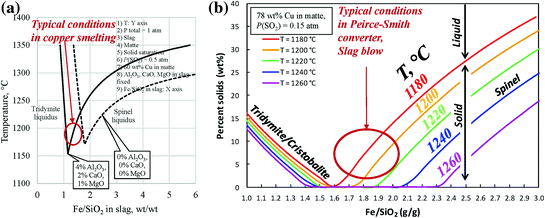
a Projection of the slag liquidus as a function of Fe/SiO2 ratio for P(SO2) = 0.5 atm. 60%Cu matte grade. b % solids in slag as a function of Fe/SiO2 and temperature for P(SO2) = 0.15 atm. 78%Cu matte grade
Pseudo-ternary Sections

Predicted liquidus surfaces of the CaO -FeO-SiO2-ZnO-Al2O3-MgO slags at lead metal saturation for a CaO /SiO2 wt. ratio = 0.72, b Zn/Fe wt. ratio = 0.8
Process Simulation
The thermodynamic databases and computer software that are now available can be applied to not only the determination of equilibria particular cases but also to the prediction and simulation of dynamic and multi-stage metallurgical processes. The databases now include critical information on a wide range of elements present in complex industrial process systems. Increasing the number of elements present in the system increases the computation time required to undertake the Gibbs Free Energy minimisation calculation routines particularly with the formation of complex solutions. This is potential limitation to the application of this technology to practice so it is worthwhile examining these issues and how they might be addressed.
By way of illustration we consider the simulation or modelling of a batch process. As in any thermodynamic calculation the first consideration is definition of the system boundaries. If this is a closed system, with no material of energy transfer taking place, then the outcomes of the reaction can be calculated in a single stage. In practice, this is rarely the case, material and energy is introduced or removed throughout the reaction; how can we deal with this and what are the implications of different calculation strategies?

Example of the process simulation of a typical Peirce-Smith converter cycle, consisting of two slag blow stages and one copper blow stage
This example demonstrates the capability of the current thermodynamic databases and computer modelling systems. In the present article, calculations were performed for the Cu-Fe-O-S-Si-(Pb-Zn-As-Bi-Sb-Sn-Ag-Au )-C-N-H chemical system, which is of interest for industrial practice. What becomes clear is that, i) the greater the number of chemical components the longer it takes to complete the calculation, and ii) the accuracy of the calculations depend on the time or increment steps considered. The smaller the steps between iterations the greater the accuracy but the longer the computational time. The time response is an important consideration if we are to introduce these tools into industrial practice. We need to select the calculation approach that is appropriate for the application. For example, an on-line “logistics” model to optimise ladle movement and charging cycles requires a rapid response, which is more important than accuracy of the predictions below a given level of uncertainty. On the other hand, accurate predictions may be required to maximise minor element recovery or impurity removal .

The effect of the number of calculation steps on the predicted outcomes of a typical Peirce-Smith converter cycle, consisting of two slag blow stages and one copper blow stage, a mass of copper blow slag , and b the concentration of sulphur in final blister copper
Optimising processing conditions, e.g. fluxing, temperature , oxygen to feed ratio and fuel utilisation.
Increasing process stability and throughput.
Improving metal recovery , through reduced losses of dissolved and entrained metal in slags.
Removing unwanted impurity elements, increasing and optimising complex feed intake.
Improving vessel integrity/lining life by reducing operating temperatures, tuning slag and refractory compositions, and stabilising operating conditions.
These predictive capabilities are already being used in industry. See an example of case study of performance of ISASMELT reactor with different types of converters [20]. As confidence in the use of these tools is increased and predictions verified by plant practice , the opportunities for the introduction of the next phase of implementation will arise—the introduction of feed forward control of pyrometallurgical reactors.
Summary
An integrated research program of thermodynamic modelling and experimental measurements of phase equilibria is being undertaken to develop accurate and internally-consistent thermodynamic databases that can be used to describe the chemical equilibria in copper , lead and zinc smelting , metal production and recycling systems. The databases contain fundamental thermodynamic descriptions of the gas-slag -matte-metal-speiss-solids phases Cu2O-PbO-ZnO-Al2O3-CaO -MgO-FeO-Fe2O3-SiO2-S-(As-Bi-Sb-Sn-Ag-Au ). The program has provided valuable new fundamental information on phase equilibria and thermodynamic properties of complex multi-component, multi-phase systems.
Acknowledgements
The authors would like to thank Australian Research Council Linkage program, Altonorte Glencore, Atlantic Copper , Aurubis, BHP Billiton Olympic Dam Operation, Kazzinc Glencore, Nyrstar, PASAR Glencore, Outotec (Espoo and Melbourne), Anglo-American Platinum , and Umicore for the financial and technical support.