The apparent endemicity of bad research behavior is alarming. In their quest for telling a compelling story, scientists too often sculpt their data to fit their preferred theory of the world.
—RICHARD HORTON
EBOLA AND BPA
In October 2014 a paper appeared in a leading scientific journal purporting to show that volunteers who handled cash register receipts after using a hand sanitizer absorbed enough of the chemical bisphenol A, or BPA, from the thermal paper to put them at increased risk of a number of serious diseases.
1 This was only the latest in a long line of scientific studies linking BPA, which is widely used to line food containers and certain plastic bottles, to a wide range of adverse health effects. In fact, both the specific findings and the interpretation were in conflict with extensive evidence from high-quality scientific studies as well as reports by various national and international agencies that argued against the existence of a hazard. Yet the authors of the paper claimed that they were picking up a true phenomenon that other scientists were missing, due either to faulty methodology or to conflicts of interest and subservience to industry.
There is nothing unusual about the paper. Rather, it is representative of a genre that has become increasingly common in the health sciences literature, particularly where researchers study the potential contribution of environmental exposures to the risk of developing serious disease. However, the paper happened to come out at a time when the Ebola epidemic in West Africa was continuing to outstrip massive efforts to bring it under control. It is instructive to compare the putative hazards stemming from this most modern of technologies (thermal printing paper) and the all-too-real threat posed by the virus that originated in an isolated and remote region of Central Africa.
In the first case, we have an exposure that is habitually described as “ubiquitous.” Virtually everyone is exposed to cash register receipts, some of which contain BPA, which is used as a developer. Additionally, it is a widely publicized fact that many synthetic chemicals, like BPA, can be detected in the urine of most people, including children, in developed countries. These are the operative facts, and often they are enough to trigger concern. What few people who are concerned about BPA realize is that the levels of the chemical that are absorbed from food containers (the major source) are miniscule. Furthermore, the chemical is almost totally (>99%) metabolized and excreted in the urine, even in infants, and therefore does not accumulate in the body. Is it possible that exposure to BPA poses some hazard to some people? Yes, we can never rule out the possibility of some effect. But based on a large amount of accumulated scientific evidence, any adverse effect, if one exists, is likely to be very small. In other words, we can’t say that an adverse effect is impossible, but we can say that it is implausible.
If a connection between exposure to BPA and human health is tenuous and lacking any strong scientific support, the effects of the Ebola virus were all too real, immediate, and horrible. Daily images and news reports from West Africa conveyed the stark and unmistakable reality: people stricken by the virus lying in the street, dying where they collapsed, with bystanders looking on helplessly; workers encased in “moon suits” spraying chlorine disinfectant in the tracks of patients being led to a clinic. The connection between the exposure and its effects could not be clearer. The virus is spread only by direct contact with the body fluids of those with symptoms, but such contact is highly infectious—so infectious that health care workers carefully removing their protective clothing could inadvertently become infected. In those who were infected, the mortality rate was roughly 60 percent. By the time the World Health Organization declared the outbreak over in 2016 there were 28,637 known cases and 11,315 deaths.
So BPA and Ebola can be taken to represent two very different types of “health risks”—two extremes—one, theoretical and likely to be undetectable; the other, all too real. Their juxtaposition raises fundamental questions about how risks are perceived and acted on, and what aspects are most salient and receive attention and what aspects are ignored. To Americans, the drama unfolding in West Africa—one of the top news stories of 2014—appeared almost surreal owing to the vast socioeconomic and cultural distance separating us from these societies. More than anything, the images conjured up the alien and nightmarish logic of movies like
Outbreak and
Contagion. However, it took only one infected traveler from Liberia to present at a Dallas hospital with a fever for Ebola to take on a drastically different meaning. The hospital staff, which had no prior experience treating the disease, was caught off guard and did not initially consider Ebola. By the time the disease was recognized it was too late, and the patient had died. One of the treating nurses, however, was infected with the virus and was transferred to the National Institutes of Health, where she recovered. These events were enough to send the country into a paroxysm of fear regarding Ebola. One survey estimated that 40 percent of Americans were concerned that a large outbreak of the disease would occur in the United States within the next year.
2
What is most striking about the response to the handful of Ebola cases in the United States is how certain facts registered, whereas others of equal or greater importance were lost sight of. What accounts for the terrible toll of Ebola in West Africa is the woefully inadequate infrastructure and medical resources in countries that are among the poorest in the world. In addition, early on in the outbreak, people did not trust their government’s information campaigns regarding the disease and were reluctant to follow its directives. If infected people are identified early and adequate treatment is available, the odds of survival are greatly improved. In the United States, with a high level of medical care and with screening of passengers coming from Africa, the chances of Ebola becoming a serious problem were very slight. In contrast, the seasonal flu spreads easily through the air by means of exhaled droplets and is responsible for tens of thousands of deaths each year, mainly in the very young and the elderly. But few Americans worry about dying from seasonal flu.
I have focused on the contrast between these two health threats—BPA and Ebola—for two reasons. First, they represent two extremes of “risk.” In the case of Ebola, the risk is so immediate and potent that no study is needed to demonstrate causality. In the case of BPA, if a risk exists at all, it is a great many orders of magnitude more subtle. Second, the contrast between Ebola and BPA highlights the widespread and dangerous confusion that surrounds both real and potential health risks.
* * *
We are awash in reports, discussions, and controversies regarding a long list of exposures that, we are told, may affect our health: genetically modified (GM) foods; “endocrine disrupting chemicals” in the environment; cell phones and their base stations; electromagnetic fields from power lines and electrical appliances; pesticides and herbicides currently in use, such as atrazine, neonicotinoids, and glyphosate, or those used in the past, such as DDT; fine particle air pollution; electronic cigarettes; smokeless tobacco; excess weight and obesity; vaccines; alcohol; salt intake; red and processed meat; screening for cancer; and dietary and herbal supplements. Each of these issues is distinct and needs to be assessed on its own terms. Each has a body of scientific literature devoted to it. Yet on many of these questions there are sharply conflicting views. In some cases (e.g., endocrine disrupting chemicals, pesticides, salt intake, smokeless tobacco products, and electronic cigarettes), this stems from splits within the scientific community, which are mirrored in the wider society. In other cases (GM foods, vaccines), there may be an overwhelming consensus among scientists against something being a risk, but vocal advocates espousing contrary views can have a strong influence on susceptible members of the public. It should be noted that in some cases, the public’s persisting belief in a hazard that is no longer supported by the scientific community has its origin either in scientific uncertainty (GM foods) or in work that was later shown to be fraudulent (vaccines).
3 In this welter of discordant scientific findings and competing claims, how are we to make sense of what is important and what is well-founded?
Understanding the sources of confusion and error surrounding the steady stream of findings from scientific studies is not just an academic concern. Beliefs about what constitutes a health threat have real consequences and affect lives, as four current issues demonstrate:
• An outbreak of measles affecting 149 people in eight U.S. states and Canada and Mexico in the winter of 2014–15 has been traced to Disneyland in California. That outbreak has been attributed to the failure of parents to allow their children to be vaccinated, based on unfounded health concerns or due to religious beliefs.
4
• With forty million smokers in the United States, many of whom want to quit, the resistance on the part of many in the public health community to acknowledge the enormous benefits of electronic cigarettes and low-carcinogen, moist smokeless tobacco as alternatives to smoking represents an abdication of reason.
5
• Food-borne illnesses caused by Salmonella, E. coli, and several other pathogens are responsible for at least 30,000 deaths each year in the United States.
6
• The powerful health supplements industry, abetted by inadequate government regulation and safeguards, markets products of unknown purity and composition to adults and minors.
7 While the majority of these products may be harmless, there have been enough cases of organ damage and death that have been convincingly linked to specific products that the whole area of dietary supplements deserves attention. A recent report has estimated that 23,000 emergency room visits each year in the United States are due to adverse events involving dietary supplements.
8
It is symptomatic of the state of the public discourse concerning health risks that issues like these do not have anywhere near the same salience as the typical scare-of-the-week.
To begin to make sense of the conflicting scientific findings and disputes bedeviling these questions, at the outset we need to be aware of the type of studies that are the source of many of these findings, and how they work. One doesn’t have to take a course in epidemiology to understand the essentials of these studies, what they can achieve, and what their limitations are. It is simply that, in most journalistic reporting of results, for understandable reasons, the underlying assumptions and limitations are rarely even touched on. This is not too surprising in view of the fact that researchers themselves often fail to hedge their results with the needed qualifications. After a brief primer on how to think about epidemiologic studies, we can proceed to look at what is required to go from an initial finding regarding a possible link between an exposure and a disease to obtaining more solid evidence that this link is likely to represent cause and effect and to account for a significant proportion of disease. Many of the findings we hear about regarding certain questions are tentative and conflicting, and we need to distinguish between such findings and those where we have firm knowledge concerning risks that actually make a difference. This chapter addresses that crucial distinction.
WHAT IS AN OBSERVATIONAL STUDY AND WHAT CAN IT TELL US?
Many of the findings concerning this or that factor that may affect our health stem from epidemiologic or “observational studies.” The term
observational is used in contrast to “experimental” studies, which are considered the “gold standard” in medical research but which, for ethical reasons, cannot be used to test the effects of harmful exposures in human beings. Observational studies of this kind involve the collecting of information on an exposure of interest in a defined population and relating this information to the occurrence of a disease of interest. Broadly speaking, two main types of study design are widely used. First, cases of a disease of interest can be identified in hospitals (or disease registries) and enrolled in a study, and a suitable comparison group (“controls”) can be selected. This is referred to as a
case-control study. Information is then obtained from cases and controls in order to identify factors that are associated with the occurrence of the disease. Note that in this type of study, cases have already developed the disease of interest, and information on past exposure is collected going back in time and usually depends on the subject’s recall. The case-control design is particularly suited for the study of rare diseases. Owing to its retrospective nature, however, it is subject to “recall bias,” since the information on exposure may be affected by the diagnosis, and cases may respond to questions about their past exposure differently from controls.
Using another approach, the researcher can identify a defined population—such as workers in a particular industry, members of a health plan, members of a specific profession, or volunteers enrolled by the American Cancer Society—and collect information on their health status and behaviors, as well as clinical specimens at the time of enrollment. The members of the “cohort” are then followed over time for the occurrence of disease and death. Information on exposure can then be related to the development of a disease of interest. This type of study is variously referred to as a cohort, prospective, or longitudinal study. Since, in this type of study, information on exposure is collected prior to the development of disease, the problem of “recall bias” present in case-control studies can be avoided.
Observational studies can provide evidence for an association between a putative exposure, or agent, and a particular disease. In a cohort study, the existence of an association is determined by comparing the occurrence of disease in those who are exposed to the factor of interest to its occurrence in those without the exposure—or those with a much lower level of exposure. The measure of association in a cohort study is called the
relative risk. In a case-control study, one compares the occurrence of the exposure of interest between cases and controls. The measure of association summarizing this relationship is referred to as the
odds ratio. If the relative risk or odds ratio is greater than 1.0 (no association), and the role of chance is deemed to be low, there is a positive association between the exposure and the disease. If the relative risk or odds ratio is below 1.0, this indicates that the exposure is inversely associated with the disease, possibly indicating a protective effect.
But what is an association? An association is merely a statistical relationship between two variables, similar to a correlation. If two variables are correlated, as one increases, the other increases. Every epidemiology and statistics textbook emphasizes that the existence of an association does not provide proof of causation. There are innumerable examples of correlations that have nothing to do with causation. In the 1950s statisticians pointed out that, internationally, sales of silk stockings tended to be highly correlated with cigarette consumption. Another example is that taller people tend to have higher IQs, but height is clearly not a cause of increased intelligence.
Finding a new association between an exposure and a disease, or physiologic state, is only the first step in a demanding process of obtaining the highest-quality data possible to confirm the existence of the association and to understand what it means. Does it represent a statistical fluke? Is an observed association between two factors merely due to their association with a third factor and thus merely a secondary phenomenon? How strong is the association? How consistently is it observed in different studies, and particularly in high-quality studies? Is it overshadowed by other, stronger and more convincing, associations? Is it consistent with a causal explanation? Is it in line with what is known regarding the biology of the disease?
Let’s examine examples of associations that have been firmly established and accepted as causal. In the 1950s and 1960s both case-control and cohort studies showed that current smokers of cigarettes had between a ten- and twentyfold increased risk of developing lung cancer compared to those who had never smoked, while former smokers had a lesser but still palpable risk. In the 1970s a number of studies showed that women who used postmenopausal hormone therapy (which at that time tended to be high-dose estrogen) had roughly a fourfold increased risk of endometrial cancer. Other studies showed that heavy consumption of alcohol was associated with increased risk of cancers of the mouth and throat, with increased risks in the range of four- to sixfold.
These three associations have been confirmed by many subsequent studies and are among the solid findings we have concerning factors that have an effect on health. Examples like these represent success stories, where researchers addressing the same question pursued an initial association using different methods in different populations, and the resulting evidence has lined up to demonstrate that these are robust findings. If one were to give a graphical presentation of the findings of many different studies on these questions, with a single point representing each study, one would see a cloud of dots—with some spread to be sure—in the quadrant indicating the existence of a substantial association.
It is crucial to realize, however, that not all associations are equal. Appreciating the distance traversed from an initial observation of an association to establishing that a specific factor or exposure is, in fact, likely to be a cause of disease is fundamental to understanding the confusion that pervades much of the reporting regarding results from observational studies. The success stories represent a miniscule fraction of the many questions—associations—that are studied, and must be studied, to uncover new and important evidence regarding the causes of disease. We must always keep in mind the denominator—in other words, the large number of questions that had to be explored in order to reach a solid new finding that makes a difference.
Science works by reducing the complexity of a biological system to what the researcher posits may be crucial players. In the simplest instance, one posits that exposure A is associated with disease B. In epidemiologic studies involving humans, this means examining the association between A and B in the population one has selected to study. The association between A and B can be represented by the arrow connecting them, as in
figure 2.1. In the case of smoking and lung cancer, we have a strong exposure—that is to say, the average smoker may smoke roughly a pack of cigarettes per day for a period of more than forty years. Over decades, the repeated exposure of the lungs to the many toxins and carcinogens in tobacco smoke increases the likelihood of abnormal changes in the cells lining the airways and lungs, thereby increasing the chances that the smoker will develop lung cancer. Thus, in retrospect, based on hundreds of studies—epidemiologic, clinical, biochemical, and experimental—the association of cigarette smoking and lung cancer is now supported by a wealth of confirmatory evidence. So this is the basis for the statement that a current smoker has roughly a twentyfold increased risk of developing lung cancer.

What is too often lost sight of is that the established causal association between smoking and lung cancer is the result of decades of accumulated evidence. This strong association between a common exposure (roughly forty million Americans are still smokers) and a major cancer provides an important standard for gauging other reported associations. The two other exposures I mentioned above—use of postmenopausal hormones and heavy alcohol consumption—are also significant risk factors, although not as strong as smoking—and the associations observed with them are also credible.
Many of the research findings that get a great deal of media attention involve associations where the linkage between exposure A and disease, or physiologic state, B is preliminary and tenuous, and the observed association is much weaker than are those just mentioned. The one-line figure connecting an exposure and a disease, which are both isolated from their context, is hardly a realistic representation of what is involved. A more accurate representation would depict the many factors that are correlated with exposure A, the many other factors that may influence disease B, either positively or negatively, and the many intervening steps and linkages that are an integral part of the context in which exposure A may be associated with disease B.
Figure 2.2 depicts this more complete picture.

In addition to the complexity of the linkages between a particular exposure and a disease, another problem is that other exposures are typically ignored to focus on the association of interest. To make this point more concrete, let’s consider the situation of a researcher who is interested in the possible associations of human exposure to various chemicals in the environment on the risk of death and disease. This is an area of great interest to health researchers. However, the chemical pollutants that are studied are often correlated both with one another and with clinical variables, such as triglycerides, cholesterol, blood pressure, body mass index, and vitamin E levels, that are also associated with disease risk. This is shown in the “correlation globe” in
figure 2.3. The lines connecting different points on the circle indicate correlations (either positive or negative) among the various environmental pollutants and clinical risk factors measured in blood or urine in the National Health and Nutrition Examination Survey between 1999 and 2006.
9
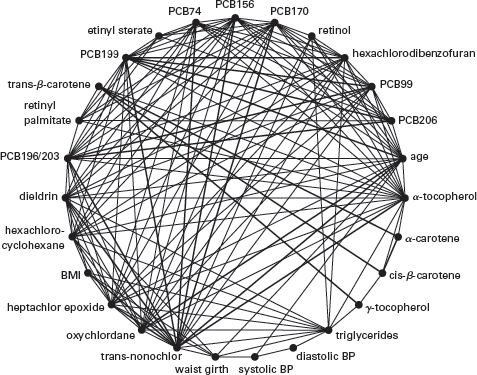
The figure drives home the importance of taking into account the many correlated exposures simultaneously to assess the importance of any specific factor, rather than isolating one factor, or a small number of factors, of interest and disregarding the complex nexus in which they are embedded. Many associations are there to be discovered; however, owing to the problem of correlation, and confounding, these associations tell us little about causation. Needless to say, the environmental and clinical factors shown in
figure 2.3 represent only a small fraction of all potentially relevant factors.
In spite of what is written in textbooks, taught to graduate students, and explained in the best science journalism, researchers and laypeople alike are capable of slipping back into acting as if a
potential association that is being studied represents a causal association—in other words, substituting the picture in
figure 2.1 for that in
figure 2.2. In many cases, the narrow focus on the question that interests the researcher and on the data he or she has collected crowds out attention to the many limitations of the study as well as to the context in which the findings should be interpreted. I will mention just a few common deficiencies, which raise questions about the validity of an observed association. First, often we are dealing with a one-time measurement of A, and the actual exposure is in many cases minute. Second, the single measurement may be temporally at a far remove from the occurrence of the putative effect (i.e., the disease or physiologic state). In other words, this is very different from the case of smoking, where, owing to its habitual nature, the exposure is both regular and substantial, and different from exposure to the Ebola virus, where the effects are apparent within a matter of days. Finally, in many studies the exposure of interest is considered in stark isolation from the many intervening and concomitant exposures that might modify or dwarf its effects. If one only considers the isolated association that one is interested in, one risks falling for the “illusion of validity” discussed in the previous chapter, and this blinkered focus can, in turn, blot out more important factors.
A recent example of the phenomenon of highlighting the association one is interested in and ignoring the all-important context and the nuances of the data is a paper by researchers at the University of California at Berkeley claiming an association of DDT exposure in utero and the development of breast cancer in women decades later.
10 The researchers used data from fifty-four years of follow-up of 20,754 pregnancies, resulting in 9,300 live-born female offspring. Blood samples were obtained from the mothers during pregnancy and immediately after delivery. DDT levels in the mother’s blood were compared between 118 breast cancer cases, diagnosed by age 52, and 354 controls. The authors reported that one of three isoforms of DDT was nearly fourfold higher in the blood of mothers of the breast cancer cases compared to that of mothers of controls. The point to keep in mind is that, even though the authors controlled for a number of factors in their analysis, the study only considered a one-time measurement of the factor of interest and related this measurement to the occurrence of disease some fifty years later. While it is conceivable that in utero exposure to DDT may influence a woman’s risk of breast cancer, one has to step back and view the reported result in the context of all that has been passed over in the fifty-year interval separating the in utero measurement from the occurrence of disease. Although the authors adjusted for a number of characteristics of the
mothers, their analysis ignored many intervening exposures and other factors that may influence both DDT levels and their endogenous effects, as well as factors that may influence breast cancer risk in the
daughters. These include the daughter’s postnatal exposure to DDT, age at menarche, age at first birth, how many children she has had, body weight, physical activity, breast-feeding history, alcohol consumption, and use of exogenous hormones. Thus while the study attracted widespread media attention, one has to realize how tenuous the result is. Like most studies of this kind, it raises more questions than it answers.
A SURFEIT OF ASSOCIATIONS
According to the online bibliographic database PubMed, in 1969 the number of published scientific papers in the area of “epidemiology and cancer” was 625. This number increased steadily over the next forty-five years, reaching 12,030 in 2015, representing nearly a twentyfold increase. A search on the terms “environmental toxicology and cancer” yields many fewer papers—2 in 1969 and 423 in 2015—but a two-hundredfold increase. The enormous increase in publications in this area reflects the dramatic growth of the fields of epidemiology and environmental health sciences in academia, government and regulatory agencies, nongovernmental organizations, and industry.
A major asset in the conduct of epidemiologic research has been the establishment of large cohort studies. These represent enormous investments of government funding and of researchers’ time required to design, implement, and monitor studies, many of which have tens or hundreds of thousands of participants. Such studies include information on a wide range of exposures and behaviors, including medical history, personal habits and behaviors, diet, and, increasingly, clinical and genetic information. Once a cohort is established, participants can be followed for decades, and new features can be added to the existing study. Modern technology, including bar-coding of samples and questionnaires, long-term storage of blood and other clinical samples, and computerized databases, has made possible the creation of immensely valuable repositories of data that can be used to address a potentially infinite number of questions concerning factors that influence health. There are now hundreds of cohort studies of different populations around the world designed to address different questions.
But there is another side to the existence of these large, rich databases. If one has information on, say, two hundred exposures and follows the population for years, ascertaining many different outcomes and considering different modifying factors, one has a very large number of associations to investigate. Such datasets make it possible to examine an almost infinite number of associations to see what turns up in the data. This is referred to as data dredging, or
deming.
11 Want to see whether people who consume more broccoli have a lower incidence of ovarian cancer, or whether those with higher levels of BPA or some other contaminant in their blood or urine have a greater frequency of obesity, diabetes, or some other condition? One can have an answer in a couple of strokes of the keyboard. There is no end to the number of comparable questions one can address. Researchers who are funded to develop, maintain, and exploit these datasets have a strong incentive to publish the results of such analyses, even when the data to address the question are limited and the question itself has only a weak justification. Furthermore, graduate and postdoctoral students need to find new questions and to publish papers in order to establish themselves in the field. Owing to these incentives, some prominent cohort studies are the source of a huge number of publications each year. The ability afforded by these large cohort studies to address an almost infinite number of questions is captured in a cartoon that originally appeared in the
Cincinnati Enquirer in 1997 (
fig. 2.4).
An example of an area in which much work has been done but where results have been weak, inconsistent, and disappointing is the field of diet/nutrition and cancer. By the 1970s, based on geographical differences in cancer rates and animal experiments, researchers had surmised that many common cancers might be caused by excesses or deficiencies of diet. Since the 1980s thousands of epidemiologic studies have been published investigating this issue, and certain findings have garnered widespread public attention. These include dietary fat and breast cancer; coffee intake and various cancers; lycopene intake as a protective factor for prostate cancer; broccoli and other cruciferous vegetables as potential protective factors for breast and other cancers; and many others. When one looks systematically at the results of these studies, however, very few associations stand up. In its updated summary of the evidence on diet and cancer, the World Cancer Research Fund/American Institute for Cancer Research conducted a detailed review of thousands of studies examining the association between diet and cancer. The evidence regarding specific foods and beverages was classified as “limited/suggestive,” “probable,” or “convincing.” The only associations that were judged to be “convincing” were those of alcohol intake with increased risk of certain cancers, of body weight with increased risk of certain cancers, and of red meat intake with risk of colorectal cancer.
12

Another approach to examining the accumulated evidence regarding diet and cancer was taken by Schoenfeld and Ioannidis, who noted that associations with cancer risk or benefits have been claimed for most food ingredients.
13 They randomly selected fifty foods or ingredients from a popular cookbook and then searched the scientific literature for associations with cancer risk. What they found was that for most of the foods studied, positive associations were often counterbalanced by negative associations. Furthermore, the majority of reported associations were statistically significant. However, meta-analyses of these studies (that is, studies that combined the results of individual studies to obtain what amounts to a weighted average, which is more stable) produced much more conservative results: only 26 percent reported an increased or decreased risk. Furthermore, the magnitude of the associations shrank in meta-analyses from about a doubling, or a halving, of risk to no significant increase/decrease in risk. This suggests that “many single studies highlight implausibly large effects, even though evidence is weak.” When examined in meta-analyses, the associations are greatly reduced.
WHY MOST RESEARCH FINDINGS ARE FALSE
In recent years there has been growing awareness of biases and misconceptions affecting published studies in the area of biomedicine.
14 One figure, however, stands out for his prolific and penetrating research into the “research on research” in the area of health studies. John P. A. Ioannidis first attracted widespread attention with a 2005 article published in the
Public Library of Science Medicine entitled “Why Most Published Research Findings are False.” The paper caused a sensation and is the most frequently cited paper published in the journal. Actually, Ioannidis argues that the seemingly provocative assertion of the title should not come as a surprise. As he explains, the probability that a research finding is true depends on three factors: the “prior probability” of its being true; the statistical power of the study; and the level of statistical significance. The prior probability of its being true refers to the fact that, if there is some strong support for a hypothesis or for a causal association before one undertakes a study, this increases the probability of a new finding being true. “Statistical power” refers to the ability to detect an association, which is dependent on the sample size of the study and on the quality of the data. Finally, the level of statistical significance is the criterion for declaring a result “statistically significant”—that is, unlikely to be due to chance. Declaring a given result to be true when it is actually likely to be false is the result of
bias. Bias has a different meaning from what we are used to in common parlance, where it suggests a moral failing or prejudice. With respect to research design, bias refers to any aspect of a study—its analysis, interpretation, or reporting—that systematically distorts the relationship one is examining. “Thus, with increasing bias, the chances that a research finding is true diminish considerably.”
15
The power of the approach taken by Ioannidis and colleagues comes from using statistics to survey the totality of findings on a given question to assess the quality of the data and the consistency and strength of the results. This approach provides the equivalent of a topographical map of the domain of studies on a given question. If a finding is real, it should be seen in studies of comparable quality and sample size that used similar methods of analysis. If the result is apparent only in weaker studies but not the stronger ones, then the association is probably wrong. If the results are inconsistent across studies of comparable quality, one must try to identify what factors account for this inconsistency. Rather than favoring those results suggesting a positive association, one can gain valuable insight by trying to explain such inconsistencies.
Only by examining the full range of studies on a given question and considering their attributes (sample size, quality of the measurements, rigor of the statistical analysis) and the strength and consistency of results across different studies, can one form a judgment of the quality of the evidence. Just as one has to look at large groups, or populations, to identify factors that may play a role in disease, so one has to examine the entire body of studies on a given question and the consistency and magnitude of the findings to make an assessment of their strength and validity.
In the PLoS paper, Ioannidis enumerated a number of different factors that increase the likelihood that a given result is false: (1) the smaller the size of the studies conducted in a given scientific field; (2) the smaller the “effect sizes” (that is, the smaller the magnitude of the association); (3) the greater the number and the lesser the selection of tested relationships in a scientific field; (4) the greater the flexibility in designs, definitions, outcomes, and analytical modes in a scientific field; (5) the greater the financial and other interests and prejudices in a scientific field; and (6) the “hotter” a scientific field (with more scientific teams involved). Notice that the factors influencing the likelihood of a false result range from the most basic design features (the size of the study) to sociological and psychological factors.
This approach to judging the credibility of the scientific evidence on a given question can be applied to vastly different content areas, and Ioannidis has collaborated with a large number of researchers in diverse fields to evaluate the evidence on many important questions. In hundreds of articles examining an extraordinary range of specialties and research questions—from etiology and treatment of specific diseases to the role of genetics, to the effectiveness of psychotherapy—Ioannidis and his collaborators have undertaken to map and analyze “an epidemic of false claims.”
16
A second paper by Ioannidis also published in 2005 asked the question: how often are high-impact clinical interventions that are reported in the literature later found to be wrong or found to be less impressive than indicated by the original report?
17 For this analysis, the author selected a total of forty-nine clinical research studies published in major clinical journals and cited more than one thousand times in the medical literature. He compared these papers to subsequently published studies of larger sample size and equal or superior quality. Sixteen percent of the original studies were contradicted by subsequent studies, and another 16 percent found effects that were stronger than those of the subsequent studies. Ioannidis concluded that it was not unusual for influential clinical results to be contradicted by later research or for researchers to report stronger effects than were subsequently confirmed.
Another major insight that has emerged from this work is that the quality and credibility of published results vary dramatically in different disciplines. Just as not all studies are equal in terms of quality, so not all disciplines are equal. For example, studies of the contribution of genetic variants to disease have become extremely rigorous owing to agreed-on standards for large populations and the requirement of replication. In contrast, epidemiologic studies of dietary and environmental factors tend to be much weaker and less credible. One analysis by Ioannidis and colleagues of ninety-eight meta-analyses of biomarker associations with cancer risk indicated that associations with infectious agents, such as
H. pylori, hepatitis virus, and human papillomavirus, with risk of specific malignancies were “very strong and uncontestable,” whereas associations of dietary factors, environmental factors, and sex hormones with cancer were much weaker.
18
In hundreds of articles written with many different collaborators, Ioannidis has identified the pervasive occurrence of largely unquestioned biases operating in the medical literature. His work suggests how incorrect inferences can become entrenched in the literature and become accepted wisdom, and that it takes work to counteract this tendency. Some of the main themes that emerge in different contexts in this work are that initial findings with strong results tend to be followed by studies showing weaker results (dubbed the “Proteus phenomenon”); most true associations are inflated; even after prominent findings have been refuted, they continue to be cited as if they were true, and this can persist for years; limitations are not properly acknowledged in the scientific literature; most results in human nutrition research are implausible; and there is an urgent need for critical assessment and replication of biomedical findings. Ioannidis and colleagues conclude that new institutions and codes of research need to be devised in order to foster an improved research ecosystem.
19
ASSESSING CAUSALITY
Most questions that arise concerning human health cannot be answered by conducting a clinical trial. Therefore experimental proof is usually not a possibility, and most often we have to rely on observational studies. For the past forty years there has been an ongoing debate in the public health community regarding the extent to which causal inferences can be drawn from observational studies, and methods for improving the validity of inferences from epidemiologic studies have become much more sophisticated in the past two decades. In 1965 the British statistician Austin Bradford Hill published a landmark paper titled “The Environment and Disease: Association or Causation?” in which he discussed a number of considerations for use in judging whether an association was likely to be causal.
20 These are the strength of the association, consistency, specificity, temporality, biological gradient, plausibility, coherence, experimental evidence, and analogy. These have become enshrined as the Hill “criteria of judgment,” although Hill never used the word “criteria” and never argued that a simple checklist could be used to make a definitive judgment about the causality of an association. Exceptions can be found to all of Hill’s items.
21 Even fulfillment of all the “viewpoints” does not guarantee that an association is causal. In his influential paper, Hill actually emphasized the pitfalls of measurement error and overreliance on statistical significance in drawing conclusions about causality. However, in spite of his clear caveats and in spite of the improvement in epidemiologic methods for dealing with bias and error in epidemiologic studies, the “criteria of judgment” are still widely taught and widely invoked in the medical literature to assess the evidence on a given question.
22 Ironically, his “considerations” may be most appropriate when they are applied to questions where we have strong evidence from a number of different disciplines that support a causal association, as in the cases of cigarette smoking and lung cancer and human papillomavirus and cervical cancer.
Our ideas about causality derive from our early experience of the world.
23 And we tend to simplify by paying attention to the single most evident or the most proximate cause of some event. We do not tend to think in terms of multiple causes, but, as is true of historical events, disease conditions never have just one cause. In fact, even in the case of smoking and lung cancer, where we have a very strong, established cause, smoking by itself is not sufficient to cause the disease, since only one-seventh of smokers develop lung cancer. Similarly, in the case of infectious diseases, some people will not be susceptible by virtue of their immune competence, whereas others may be particularly susceptible because of poor nutritional status or poor resistance. Other chronic diseases like heart disease have a large number of component causes. What this means is that causality in the area of epidemiology is much more complex than our everyday notions allow for.
In the absence of a simple checklist that can be used to determine whether a given association is likely to be causal, the epidemiologists Ken Rothman and Sander Greenland have proposed an alternative to the invocation of Hill’s “criteria.”
24 They argue that careful exploration of the available data is necessary and that, in addition to considering the evidence that appears to support an association, one must explore the many pitfalls and biases in the study design and the data that could produce a spurious association. They opt for an intermediate position between those who unreservedly support the use of a checklist of causal criteria and those who reject it as having no value. According to Rothman and Greenland, “such an approach avoids the temptation to use causal criteria simply to bolster pet theories at hand, and instead allows epidemiologists to focus on evaluating competing causal theories using crucial observations.”
The concluding paragraph of their article is worth quoting in full:
Although there are no absolute criteria for assessing the validity of scientific evidence, it is still possible to assess the validity of a study. What is required is much more than the application of a list of criteria. Instead, one must apply thorough criticism, with the goal of obtaining a qualified evaluation of the total error that afflicts the study. This type of assessment is not one that can be done easily by someone who lacks the skills and training of a scientist familiar with the subject matter and the scientific methods that were employed. Neither can it be applied readily by judges in court, nor by scientists who either lack the requisite knowledge or who do not take the time to penetrate the work.
25
Rothman and Greenland’s prescription for unstintingly critical assessment of individual studies and, by implication, of the entire body of studies bearing on a specific question represents an ideal to which the field should aspire. There is no simple formula for assessing the evidence on a given topic. The evidence has to be evaluated on its own terms, taking into account all relevant findings and holding them up to scrutiny. It is painfully clear, however, that many scientific reports fall far short of critically evaluating the data they present. Being critical of one’s own findings and of the work of others on a question in which one has a stake is a tall challenge and a commodity in short supply.
STRONG INFERENCE
In spite of the many pitfalls and occasions for bias in observational studies, somehow science manages to make undreamed of advances. Rothman and Greenland point out that science has made impressive advances that were not arrived at through experimentation, and they cite the discovery of tectonic plates, the effects of smoking on human health, the evolution of species, and planets orbiting other stars as examples.
26 And there are many other examples relating to the health sciences. Two questions at the heart of this book are, first, how is it that extraordinary progress is made in solving certain problems, whereas in other areas little progress is made, and, second, why do instances of progress get so little attention, while those issues that gain attention often tend to be scientifically questionable?
Part of the answer lies in the nature of the scientific enterprise. Science is defined as “knowledge about, or study of, the natural world based on facts learned through experiments and observation.” The Greek word for “to find” or “to discover” is heuriskein, and the word heuristics has made it into a common parlance to refer to techniques—such as “trial-and-error”—that lead to discovery. Use of the word heuristics implies that inherent in the scientific process is the fact that one cannot tell in advance where a given line of inquiry will lead, and that one doesn’t know what one has found until one has found it. (This undoubtedly accounts for much of the excitement and exhilaration of science.)
At the same time, we have seen that often shortcuts—or simplified ideas—are resorted to in order to make sense of a difficult question, and these too have been referred to as “heuristics.” The fact that the same word is used to refer both to the unpredictable process of arriving at solid new knowledge and to oversimplifications or false leads suggests how uncertain the process of scientific discovery is.
Where do strong hypotheses come from, and how does one identify a productive line of inquiry? While there is no way to generalize, it is clear that being able to formulate a novel hypothesis requires a thoroughgoing, critical assessment of the existing evidence on a question. In this task, there are no shortcuts or general prescriptions that are applicable to all circumstances. There is only the coming to grips with the particulars of what is known about a question and formulating a picture of the terrain and evaluating the important features in order to identify gaps, reconcile conflicting findings, and come up with new connections.
Because we are dealing with complex, multifactorial diseases, such as breast cancer, heart disease, and Alzheimer’s, it has to be understood that many alternative ideas must be explored, and most will not lead anywhere. This is not surprising, and there is no way to know in advance which approach will prove productive. However, the uncovering of new basic knowledge is likely to lead to a deeper understanding of a problem. Furthermore, progress is made in some areas and not in others. For example, striking progress has been achieved over the past thirty years in the treatment of breast cancer, whereas, in spite of an enormous amount that has been learned about the disease and its risk factors, we lack knowledge that would enable us to predict who will develop breast cancer or to prevent the disease.
27
The critical assessment of evidence is part and parcel of coming up with new and better ideas. Often a field gets fixated on a particular idea, and this can stand in the way of fresh thinking and block the path to coming up with new ideas. There is a tendency for researchers to rework the same terrain over and over and produce studies that add little or nothing to what was done earlier. A prominent epidemiologist has referred to this as “circular epidemiology.”
28 Another epidemiologist, who has been involved in the study of a number of high-profile environmental exposures, has stressed the importance of knowing when research on a topic has reached the point of diminishing returns, so that resources and energy can be devoted to other, more fruitful avenues.
29
What can one say about the attitudes and methods that lead to new ways of seeing a problem and making real progress? Of course, it is not possible to be programmatic or prescriptive. However, when one examines specific problems and what their study has yielded—as in the four case studies that make up the core of this book—there are characteristics and attitudes that appear to have played a major role in making possible dramatic progress. On the other hand, other areas of study have characteristics that help explain why a question can continue to be a source of concern and to attract research with little prospect of making progress.
The first, and least teachable, faculty or characteristic that can lead to transformative insight into a problem is being observant and being able to see things that are in front of one’s eyes, or in the data on a question. We will see striking examples of this in later chapters. Second, a productive hypothesis often stems from a strong signal. For example, why are the rates of a particular disease dramatically higher in one geographic region or in one social or ethnic group than another? Why has a disease increased, or decreased, in frequency? Or, if one is interested in a specific exposure, it makes sense to study a population or occupational group that has a high exposure to see if one can document effects in that population. These kinds of questions have often provided the starting point for epidemiologic investigations. Third, one must characterize the phenomenon under study in all its particularity, defining a disease entity and documenting its natural history, relevant exposures and cofactors, and environmental conditions associated with it. Fourth, it is important to look for contradictions in the evidence, for things that don’t fit. This can lead one to modify a hypothesis and sharpen it, or to reject it in part or in its totality. Fifth, a particularly fruitful tactic is to make connections between phenomena that have not previously been brought into relation. We will see examples of this in the last two case studies. Finally, one has to be willing to question the most basic prevailing assumptions and take a fresh look at the evidence, as Abraham Wald did when examining the pattern of damage on the returning Lancasters. This can lead to overturning of the reigning dogma, as occurred when Barry Marshall and Robin Warren demonstrated that, contrary to entrenched opinion, bacteria could grow in the stomach, in spite of the high acidity. And they proceeded to demonstrate that bacterial infection with Helicobacter pylori is a major cause of stomach ulcers and stomach cancer. Their work completely overturned the prevailing wisdom that ascribed stomach ulcers to psychological stress and to eating spicy food. Something similar occurred when Harald zur Hausen rejected the idea that herpes simplex virus was a cause of cervical cancer and turned his attention to a totally difference class of viruses, the papillomaviruses.
THE METHOD OF EXCLUSION
Although intangible factors like instinct and luck can play a role in identifying a productive line of inquiry, too little attention is given to the practice of rigorous reasoning in considering competing hypotheses and excluding possibilities. In 1964 the biophysicist John R. Platt of the University of Chicago published a paper in the journal
Science entitled “Strong Inference”—a paper that should be read by anyone with an interest in what distinguishes successful science.
30 Platt argued that certain systematic methods of scientific thinking can produce much more rapid progress than others. He saw the enormous advances in molecular biology and high-energy physics as examples of what this approach can yield. He wrote, “On any new problem, of course, inductive inference is not as simple and certain as deduction, because it involves reaching out into the unknown.” “Strong inference” involves (1) devising alternative hypotheses; (2) devising a crucial experiment that will exclude one or more hypotheses; (3) carrying out the experiment so as to get a clean result; and (4) repeating the procedure, devising further hypotheses to refine the possibilities that remain. Of course, in epidemiology and public health we are not talking about carrying out experiments. Nevertheless, Platt’s prescription for a rigorous approach to the evidence is highly relevant to epidemiology and very much in line with Rothman and Greenland’s and Ioannidis’s prescription for unstinting, critical evaluation of the biases and errors that afflict studies on a given question.
Platt aligns himself with Francis Bacon, who emphasized the power of “proper rejections and exclusions,” and Karl Popper, who posited that a useful hypothesis is one that can be falsified. And he goes on to cite the “second great intellectual invention,” “the method of multiple hypotheses” put forward by the geologist T. C. Chamberlin of the University of Chicago in 1890. Chamberlin advocated the formulation of multiple hypotheses as a means of guarding against one’s natural inclination to become emotionally invested in a single hypothesis.
31 This approach of pursuing evidence that bears on multiple hypotheses makes it possible to adjudicate between competing hypotheses. Following Chamberlin, Platt argues that adopting the method of entertaining multiple hypotheses leads to a collective focus on the work of disproof and exclusion and transcends conflicts between scientists holding different hypotheses.
32
Platt is unsparing in his assessment of those who collect and enumerate data without formulating clear hypotheses:
Today we preach that science is not science unless it is quantitative. We substitute correlations for causal studies, and physical equations for organic reasoning. Measurements and equations are supposed to sharpen thinking, but, in my observation, they more often tend to make the thinking noncausal and fuzzy. They tend to become the object of scientific manipulation instead of auxiliary tests of crucial inferences.
What I am saying is that, in numerous areas that we call science, we have come to like our habitual ways, and our studies that can be continued indefinitely. We measure, we define, we compute, we analyze, but we do not exclude. And this is not the way to use our minds most effectively or to make the fastest progress in solving scientific questions.
The man to watch, the man to put your money on is not the man who wants to make “a survey” or a “more detailed study” but the man with the notebook, the man with the alternative hypotheses and the crucial experiments, the man who knows how to answer your question of disproof and is already working on it.
33
Platt distinguished two types of science: science that uses a systematic method to identify the next step and make progress and science that is stuck in place and has no strategy to make exclusions and to move forward. But his focus is on the scientific establishment alone, and, tellingly, he has nothing to say about the interaction between science and the wider society. Nevertheless, one has no trouble imagining what he would think of scientists who resort to the court of public opinion to gain support for the importance of their work, rather than coming to grips with the totality of the evidence and subjecting a favored hypothesis to unstinting criticism. It is to the question of the interaction between science touching on high-profile health issues and the wider society that we must turn in the following chapter.