



Fréchet means are perhaps the most basic object of statistical interest, and this chapter studies such means when the underlying space is the Wasserstein space . In general, existence and uniqueness of a Fréchet mean can be subtle, but we will see that the nature of optimal transport allows for rather clean statements in the case of Wasserstein space.
3.1 Empirical Fréchet Means in
3.1.1 The Fréchet Functional
As foretold in the preceding paragraph, the definition of a Fréchet mean requires the definition of an appropriate sum-of-squares functional, the Fréchet functional:


A Fréchet mean of (μ
1, …, μ
N) is a minimiser of F in (if it exists).
In analysis, a Fréchet mean is often called a barycentre. We shall use the terminology of “Fréchet mean” that is arguably more popular in statistics.2
The factor 1∕(2N) is irrelevant for the definition of Fréchet mean. It is introduced in order to have simpler expressions for the derivatives (Theorems 3.1.14 and 3.2.13) and to be compatible with a population version (see (3.3)).


3.1.2 Multimarginal Formulation, Existence, and Continuity








We refer to elements of Π(μ 1, …, μ N) (equivalently, joint laws of X 1, …, X N) as multicouplings (of μ 1, …, μ N). Just like in the Kantorovich problem, there always exists an optimal multicoupling π.

Let . Then μ is a Fréchet mean of (μ
1, …, μ
N) if and only if there exists an optimal multicoupling
of (μ
1, …, μ
N) such that μ = M#π, and furthermore F(μ) = G(π).


For the other inequality, let be arbitrary. For each i, let π
i be an optimal coupling between μ and μ
i. Invoking the gluing lemma (Ambrosio and Gigli [10, Lemma 2.1]), we may glue all π
i’s using their common marginal μ. This procedure constructs a measure η on
with marginals μ
1, …, μ
N, μ and its relevant projection π is then a multicoupling of μ
1, …, μ
N.






Since optimal couplings exist, we deduce that so do Fréchet means.


and when p > 1 equality holds if and only if μ 1 = ⋯ = μ N.

A further corollary of Proposition 3.1.2 is a bound on the support:

In particular, if all the μ i’s are supported on a common convex set K, then so is any of their Fréchet means.
The multimarginal formulation also yields a continuity property for the empirical Fréchet mean. Conditions for uniqueness will be given in the next subsection.
Suppose that for i = 1, …, N and let
denote any Fréchet mean of
. Then
stays in a compact set of
, and any limit point is a Fréchet mean of (μ
1, …, μ
N).
In particular, if μ
1, …, μ
N have a unique Fréchet mean , then
in
.
We sketch the steps of the proof here, with the full details given on page 70 of the supplement.
Step 1: tightness of . This is true because the collection of multicouplings is tight, and the mean function M is continuous.
Step 2: weak limits are limits in . This holds because the mean function has linear growth.
Step 3: the limit is a Fréchet mean of (μ
1, …, μ
N). From Corollary 3.1.3, it follows that must be sought on some fixed bounded set in
. On such sets, the Fréchet functionals are uniformly Lipschitz, so their minimisers converge as well.
3.1.3 Uniqueness and Regularity
A general situation in which Fréchet means are unique is when the Fréchet functional is strictly convex. In the Wasserstein space, this requires some regularity, but weak convexity holds in general. Absolutely continuous measures on infinite-dimensional are defined in Definition 1.6.4.


When Λ is absolutely continuous, the inequality is strict unless t ∈{0, 1} or γ 1 = γ 2.
The Wasserstein distance is not convex along geodesics. That is, if we replace the linear interpolant tγ
1 + (1 − t)γ
2 by McCann’s interpolant, then is not necessarily convex (Ambrosio et al. [
12, Example 9.1.5]).
}, \end{aligned}$$](../images/456556_1_En_3_Chapter/456556_1_En_3_Chapter_TeX_Equn.png)



As a corollary, we deduce that the Fréchet mean is unique if one of the measures μ i is absolutely continuous, and this extends to the population version (see Proposition 3.2.7).
We conclude this subsection by stating an important regularity property in the Euclidean case. See Agueh and Carlier [2, Proposition 5.1] for a proof.
Let and suppose that μ
1 is absolutely continuous with density bounded by M. Then the Fréchet mean of {μ
i} is absolutely continuous with density bounded by N
dM and is consequently a Karcher mean.
In Theorem 5.5.2, we extend Proposition 3.1.8 to the population level.
3.1.4 The One-Dimensional and the Compatible Case










![$$\displaystyle \begin{aligned} \left[\frac 1N \sum_{i=1}^N {\mathbf t_{\gamma}^{\mu^i}}\right]\#\gamma \end{aligned}$$](../images/456556_1_En_3_Chapter/456556_1_En_3_Chapter_TeX_Equt.png)
is the Fréchet mean of (μ 1, …, μ N).
A population version is given in Theorem 5.5.3.
3.1.5 The Agueh–Carlier Characterisation
Agueh and Carlier [2] provide a useful sufficient condition for γ to be the Fréchet mean. When , this condition is also necessary [2, Proposition 3.8], hence characterising Fréchet means in
. It will allow us to easily deduce some equivariance results for Fréchet means with respect to independence (Lemma 3.1.11) and rotations (3.1.12). More importantly, it provides a sufficient condition under which a local minimum of F is a global minimum (Theorem 3.1.15) and the same idea can be used to relate the population Fréchet mean to the expected value of the optimal maps (Theorem 4.2.4). Recall that ϕ
∗ denotes the Legendre transform of ϕ, as defined on page 14.






then γ is the unique Fréchet mean of μ 1, …, μ N.


A population version of this result, based on similar calculations, is given in Theorem 4.2.4.
The next two results are formulated in because then the converse of Proposition 3.1.10 is proven to be true. If one could extend [2, Proposition 3.8] to any separable Hilbert
, then the two lemmata below will hold with
replaced by
. The simple proofs are given on page 74 of the supplement.
Let μ
1, …, μ
N and ν
1, …, ν
N be absolutely continuous measures in and
with Fréchet means μ and ν, respectively. Then the independent coupling μ ⊗ ν is the Fréchet mean of μ
1 ⊗ ν
1, …, μ
N ⊗ ν
N.
By induction (or a straightforward modification of the proof), one can show that the Fréchet mean of (μ i ⊗ ν i ⊗ ρ i) is μ ⊗ ν ⊗ ρ, and so on.
If μ is the Fréchet mean of the absolutely continuous measures μ 1, …, μ N and U is orthogonal, then U#μ is the Fréchet mean of U#μ 1, …, U#μ N.
3.1.6 Differentiability of the Fréchet Functional and Karcher Means
Since we seek to minimise the Fréchet functional F, it would be helpful if F were differentiable, because we could then find at least local minima by solving the equation F′ = 0. This observation of Karcher [78] leads to the notion of Karcher mean.
Let F be a Fréchet functional associated with some random measure Λ in . Then γ is a Karcher mean for Λ if F is differentiable at γ and F′(γ) = 0.
Of course, if γ is a Fréchet mean for the random measure Λ and F is differentiable at γ, then F′(γ) must vanish. In this subsection, we build upon the work of Ambrosio et al. [12] and determine the derivative of the Fréchet functional. This will not only allow for a simple characterisation of Karcher means in terms of the optimal maps (Proposition 3.2.14), but will also be the cornerstone of the construction of a steepest descent algorithm for empirical calculation of Fréchet means. The differentiability holds at the population level too (Theorem 3.2.13).











It follows from this that an absolutely continuous is a Karcher mean if and only if the average of the optimal maps is the identity. If in addition one μ
i is absolutely continuous with bounded density, then the Fréchet mean
is absolutely continuous by Proposition 3.1.8, so it is a Karcher mean. The result extends to the population version; see Proposition 3.2.14.
It may happen that a collection μ
1, …, μ
N of absolutely continuous measures have a Karcher mean that is not a Fréchet mean; see Álvarez-Esteban et al. [9, Example 3.1] for an example in . But a Karcher mean γ is “almost” a Fréchet mean in the following sense. By Proposition 3.2.14,
for γ-almost all x. If, on the other hand, the equality holds for all
, then γ is the Fréchet mean by taking integrals and applying Proposition 3.1.10. One can hope that under regularity conditions, the γ-almost sure equality can be upgraded to equality everywhere. Indeed, this is the case:


- 1.
and the densities f, g 1, …, g N are of class C 0, α for some α > 0;
- 2.
U is bounded and the densities f, g 1, …, g N are bounded below on U.
The result exploits Caffarelli’s regularity theory for Monge–Ampère equations in the form of Theorem 1.6.7. In the first case, there exist C
1 (in fact, C
2, α) convex potentials φ
i on with
, so that
is a singleton for all
. The set
is γ-negligible (and hence Lebesgue negligible) and open by continuity. It is therefore empty, so F′(γ) = 0 everywhere, and γ is the Fréchet mean (see the discussion before the theorem).
In the second case, by the same argument we have for all x ∈ U. Since U is convex, there must exist a constant C such that
for all x ∈ U, and we may assume without loss of generality that C = 0. If one repeats the proof of Proposition 3.1.10, then F(γ) ≤ F(θ) for all θ ∈ P(U). By continuity considerations, the inequality holds for all
(Theorem 2.2.7) and since
is closed and convex, γ is the Fréchet mean by Corollary 3.1.3.
3.2 Population Fréchet Means
In this section, we extend the notion of empirical Fréchet mean to the population level, where Λ is a random element in (a measurable mapping from a probability space to
). This requires a different strategy, since it is not clear how to define the analogue of the multicouplings at that level of abstraction. However, it is important to point out that when there is more structure in Λ, multicouplings can be defined as laws of stochastic processes; see Pass [102] for a detailed account of the problem in this case.
In analogy with (3.1), we define:


Since W 2 is continuous and nonnegative, the expectation is well-defined.
3.2.1 Existence, Uniqueness, and Continuity
Existence and uniqueness of Fréchet means on a general metric space M are rather delicate questions. Usually, existence proofs are easier: for example, since the Fréchet functional F is continuous on M (as we show below), one often invokes local compactness of M in order to establish existence of a minimiser. Unfortunately, a different strategy is needed when , because the Wasserstein space is not locally compact (Proposition 2.2.9).
The first thing to notice is that F is indeed continuous (this is clear for the empirical version). This is a consequence of the triangle inequality and holds when is replaced by any metric space.
If F is not identically infinite, then it is finite and locally Lipschitz everywhere on .

![$$\displaystyle \begin{aligned} 2F(\gamma) - 2F(\theta) =\mathbb{E}[W_2(\gamma,\varLambda) - W_2(\theta,\varLambda)] [W_2(\gamma,\varLambda) + W_2(\theta,\varLambda)]. \end{aligned}$$](../images/456556_1_En_3_Chapter/456556_1_En_3_Chapter_TeX_Equad.png)

![$$\displaystyle \begin{aligned} 2|F(\gamma) - F(\theta)| &\le W_2(\gamma,\theta) [2\mathbb{E} W_2(\gamma,\varLambda) + W_2(\theta,\gamma)]\\ &\le W_2(\gamma,\theta) [2\mathbb{E} W_2^2(\gamma,\varLambda) + 2 + W_2(\theta,\gamma)]. \end{aligned} $$](../images/456556_1_En_3_Chapter/456556_1_En_3_Chapter_TeX_Equae.png)



Using the lower semicontinuity (2.5), one can prove existence on rather easily. (The empirical means exist even in infinite dimensions by Corollary 3.1.3.)
The Fréchet functional associated with any random measure Λ in
admits a minimiser.






When is an infinite-dimensional Hilbert space, existence still holds under a compactness assumption. We first prove a result about the support of the Fréchet mean. At the empirical level, one can say more about the support (see Corollary 3.1.4).
Let Λ be a random measure in and let
be a convex closed set such that
. If γ minimises F, then γ(K) = 1.
For any closed and any α ∈ [0, 1], the set
is closed in
because
is weakly closed by the portmanteau lemma (Lemma 1.7.1
).
The proof amounts to a simple projection argument; see page 79 in the supplement.
If there exists a compact convex K satisfying the hypothesis of Proposition 3.2.4, then the Fréchet functional admits a minimiser supported on K.
Proposition 3.2.4 allows us to restrict the domain of F to , the collection of probability measures supported on K. Since this set is compact in
(Corollary 2.2.5), the result follows from continuity of F.
From the convexity (3.2), one obtains a simple criterion for uniqueness. See Definition 1.6.4 for absolute continuity in infinite dimensions.
Let Λ be a random measure in
with finite Fréchet functional. If Λ is absolutely continuous with positive (inner) probability, then the Fréchet mean of Λ is unique (if it exists).
It is not obvious that the set of absolutely continuous measures is measurable in
. We assume that there exists a Borel set
such that
and all measures in A are absolutely continuous.
We state without proof an important consistency result (Le Gouic and Loubes [87, Theorem 3]). Since is a complete and separable metric space, we can define the “second degree” Wasserstein space
. The law of a random measure Λ is in
if and only if the corresponding Fréchet functional is finite.
Let Λ
n, Λ be random measures in with finite Fréchet functionals and laws
. If
in
, then any sequence λ
n of Fréchet means of Λ
n has a W
2-limit point λ, which is a Fréchet mean of Λ.
See the Bibliographical Notes for a more general formulation.
Let Λ be a random measure in with finite Fréchet functional and let Λ
1, … be a sample from Λ. Assume λ is the unique Fréchet mean of Λ (see Proposition 3.2.7). Then almost surely, the sequence of empirical Fréchet means of Λ
1, …, Λ
n converges to λ.
Let be the law of Λ and let
be its empirical counterpart (a random element in
. Like in the proof of Proposition 2.2.6 (with
replaced by the complete separable metric space
), almost surely
in
and Theorem 3.2.9 applies.
Under a compactness assumption, one can give a direct proof for the law of large numbers as in Theorem 3.1.5. This is done on page 80 in the supplement.
3.2.2 The One-Dimensional Case
As a generalisation of the empirical version, we have:

Let Λ be a random measure in with finite Fréchet functional. Then the Fréchet mean of Λ is the unique measure λ with quantile function
, t ∈ (0, 1).
Since L
2(0, 1) is a Hilbert space, the random element has a unique Fréchet mean g ∈ L
2(0, 1), defined by the relations
for all f ∈ L
2(0, 1). On page 80 of the supplement, we show that g can be identified with
.
Interestingly, no regularity is needed in order for the Fréchet mean to be unique. This is not the case for higher dimensions, see Proposition 3.2.7. If there is some regularity, then one can state Theorem 3.2.11 in terms of optimal maps, because is the optimal map from Leb|[0,1] to Λ. If
is any absolutely continuous (or even just continuous) measure, then Theorem 3.2.11 can be stated as follows: the Fréchet mean of Λ is the measure
. A generalisation of this result to compatible measures (Definition 2.3.1) can be carried out in the same way, since compatible measures are imbedded in a Hilbert space, using the Bochner integrals for the definition of the expected optimal maps (see Sect. 2.4).
3.2.3 Differentiability of the Population Fréchet Functional



where u is defined by (3.4). If the measures are compatible in the sense of Definition 2.3.1 , then u(θ, Λ) = δ∕2.
The proof is a slight variation of Ambrosio et al. [12, Theorem 10.2.2 and Proposition 10.2.6], and the details are given on page 81 of the supplement.


Thus, the Fréchet derivative of F can be identified with the map in the tangent space at θ
0, a subspace of
.



Let Λ be a random measure in
with finite Fréchet functional F, and let γ be absolutely continuous in
. Then γ is a Karcher mean of Λ if and only if
in
. Furthermore, if γ is a Fréchet mean of Λ, then it is also a Karcher mean.
The characterisation of Karcher means follows immediately from Theorem 3.2.13. The other statement is that the derivative vanishes at the minimum, which is fairly obvious intuitively; see page 82 in the supplement.
3.3 Bibliographical Notes
Proposition 3.1.2 is essentially due to Agueh and Carlier [2, Proposition 4.2], who show it on (see also Zemel and Panaretos [134, Theorem 2]). An earlier result in a compact setting can be found in Carlier and Ekeland [33]. The formulation given here is from Masarotto et al. [91]. A more general version is provided by Le Gouic and Loubes [87, Theorem 8].
Lemmata 3.1.11 and 3.1.12 are from [135], but were known earlier (e.g., Bonneel et al. [30]).
Proposition 3.1.6 is a simplified version of Álvarez-Esteban et al. [8, Theorem 2.8] (see [8, Corollary 2.9]).
Propositions 3.2.3 and 3.2.7 are from Bigot and Klein [22], who also show the law of large numbers (Corollary 3.2.10) and deal with the one-dimensional setup (Theorem 3.2.11) in a compact setting. Section 2.4 appears to be new, but see the discussion in its beginning for other measurability results.
Barycentres can be defined for any p ≥ 1 as the measures minimising . (Strictly speaking, these are not Fréchet means unless p = 2.) Le Gouic and Loubes [87] show Proposition 3.2.3 and Theorem 3.2.9 in this more general setup, where
can be replaced by any separable locally compact geodesic space.
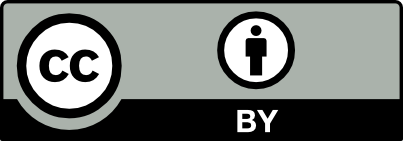
Open Access This chapter is licensed under the terms of the Creative Commons Attribution 4.0 International License (http://creativecommons.org/licenses/by/4.0/), which permits use, sharing, adaptation, distribution and reproduction in any medium or format, as long as you give appropriate credit to the original author(s) and the source, provide a link to the Creative Commons license and indicate if changes were made.
The images or other third party material in this chapter are included in the chapter's Creative Commons license, unless indicated otherwise in a credit line to the material. If material is not included in the chapter's Creative Commons license and your intended use is not permitted by statutory regulation or exceeds the permitted use, you will need to obtain permission directly from the copyright holder.